Introducing NSA: A Hardware-Aligned, Natively Trainable Sparse Attention Mechanism for Ultra-Fast Long-Context Training and Inference by DeepSeek AI
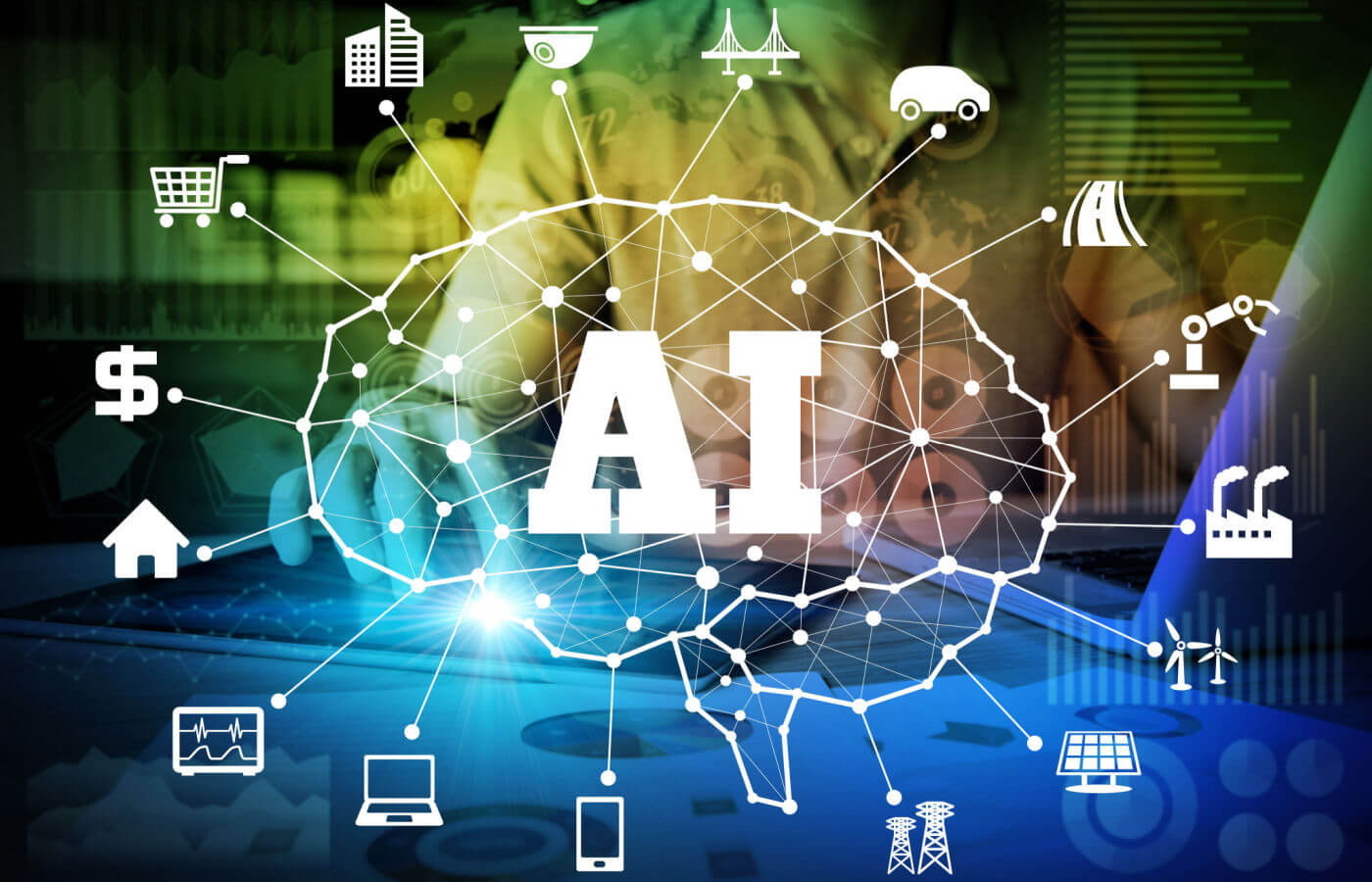
DeepSeek AI Unveils NSA: A New Approach to Sparse Attention
In the evolving field of artificial intelligence and machine learning, speeding up training and inference without sacrificing accuracy is a continual challenge. DeepSeek AI has recently made important strides by introducing a new mechanism known as NSA, which stands for Natively Trainable Sparse Attention. This innovative technology is designed to enhance training speed and efficiency, particularly for long-context processing tasks.
What is NSA?
Sparse Attention Mechanism
The core concept behind NSA is its sparse attention mechanism. Traditional attention mechanisms in neural networks typically evaluate all input elements, which can become computationally expensive, especially for long sequences. NSA offers a solution by focusing on only the most relevant parts of the input, thus reducing computational load and time.
Hardware Alignment
A standout feature of NSA is its hardware alignment. This means that the architecture of NSA is specifically designed to effectively leverage the capabilities of current hardware used for AI processing. Such alignment enhances performance by minimizing bottlenecks and maximizing processing speed.
Benefits of NSA
Implementing NSA brings several advantages that could significantly impact AI practices:
- Increased Speed: By focusing on sparse inputs, the NSA mechanism streamlines the training and inference processes, enabling faster processing times.
- Efficiency with Long Contexts: NSA is particularly beneficial for tasks involving large and complex datasets, as it maintains high levels of performance while managing long contextual information.
- Lower Resource Consumption: With reduced computational requirements, NSA allows for more efficient use of hardware resources, which can lead to cost savings and less energy consumption.
Applications of NSA
Given its unique benefits, NSA can be effectively utilized in various applications, including:
Natural Language Processing (NLP)
In NLP, tasks often require understanding lengthy texts or documents. NSA’s ability to manage long contexts efficiently can enhance models for translation, summarization, and question answering.
Image Processing
Image models that require spatial attention, such as those used in object detection and recognition, can also benefit from NSA, improving processing speeds while maintaining high accuracy.
Time-Series Forecasting
NSA’s capabilities can be advantageous in time-series analysis, where large datasets with temporal sequences are common. Its sparse attention can help in quickly identifying trends and patterns.
How NSA Works
Training Process
NSA is designed to be natively trainable, meaning it can be trained directly with minimal pre-processing. This streamlined approach reduces the complexity often associated with integrating new attention mechanisms into existing models.
Inference Speedup
During inference, NSA quickly selects relevant information from input sequences. This tightening of focus reduces the amount of data processed, leading to quicker outputs without compromising the quality of the results.
Conclusion
DeepSeek AI’s introduction of the NSA mechanism marks a significant advancement in efficient attention models for AI. By aligning closely with hardware capabilities and focusing on only the most pertinent data for processing, NSA not only enhances speed and efficiency but also holds the potential for widespread application across various fields in AI technology. With ongoing developments, the future looks promising for leveraging such sophisticated mechanisms in real-time data processing and analysis.