JetFormer: A Unified Multimodal Approach Free from Modeling Limitations
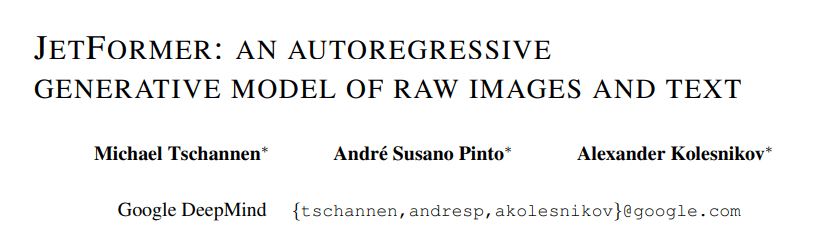
JetFormer: Advancements in Multimodal Generative Modeling
Recent developments in large multimodal models aim to break away from traditional limitations and create unified frameworks that can operate across different data types, such as images and text. While there has been progress in this area, many models continue to utilize separately trained components, including distinct encoders and decoders tailored for each modality. A new paper titled "JetFormer: An Autoregressive Generative Model of Raw Images and Text" introduces a novel solution. The research team from Google DeepMind presents JetFormer, an autoregressive, decoder-only Transformer that directly models raw data. This model is capable of understanding and generating both text and images seamlessly, without the need for pre-trained components.
Key Innovations in JetFormer
The team has highlighted several groundbreaking features that position JetFormer at the forefront of multimodal AI research.
1. Utilizing Normalizing Flows for Image Representation
One of the major advancements in JetFormer is its use of a powerful mechanism known as a normalizing flow—referred to as a "jet." This component encodes images into a latent format that is compatible with autoregressive modeling. Traditional methods that work with raw image pixels have faced challenges due to the intricate nature of image structures. JetFormer overcomes these issues by offering a lossless and invertible representation, allowing for seamless integration within a multimodal framework. This invertibility feature is particularly useful during inference, as it simplifies the process of decoding images back into their original forms.
2. Enhancing Focus on High-Level Information
To help the model concentrate on significant high-level features, the researchers implemented two innovative techniques:
Progressive Gaussian Noise Augmentation: This approach involves adding Gaussian noise during the training phase, which is gradually reduced. This encourages the model to focus on important features early in the training process.
- Managing Redundant Image Data: JetFormer allows for the selective exclusion of unnecessary dimensions in natural images, which streamlines the autoregressive model. Additionally, the technique of Principal Component Analysis (PCA) is explored to decrease dimensionality while retaining essential information.
Performance Evaluation
The team conducted extensive evaluations of JetFormer on two demanding tasks: ImageNet class-conditional image generation and web-scale multimodal generation. Results indicate that JetFormer stands out as highly competitive with existing models that may be less versatile. Its ability to generate coherent text and images effectively underscores its capabilities.
Advantages of JetFormer
End-to-End Training: JetFormer can be trained entirely as a single system, enhancing its flexibility.
- Unified Modeling Approach: By streamlining the modeling process for both images and text, JetFormer simplifies the architecture of multimodal systems, making the model more efficient.
Implications for Future Research
JetFormer signifies a notable advancement in the pursuit of streamlined multimodal architectures. By combining innovative strategies such as normalizing flows and a focus on high-level features, it opens up new avenues for further exploration in unified multimodal systems. The overarching goal is to create more integrated and effective approaches in AI model development, which could lead to more powerful and versatile applications in various fields, including natural language processing, computer vision, and beyond.
The research paper "JetFormer: An Autoregressive Generative Model of Raw Images and Text" can be found on arXiv and serves as a significant contribution to ongoing discussions in the AI community about the future of multimodal learning.