Key Insights for MSSPs on Tackling AI Security Vulnerabilities
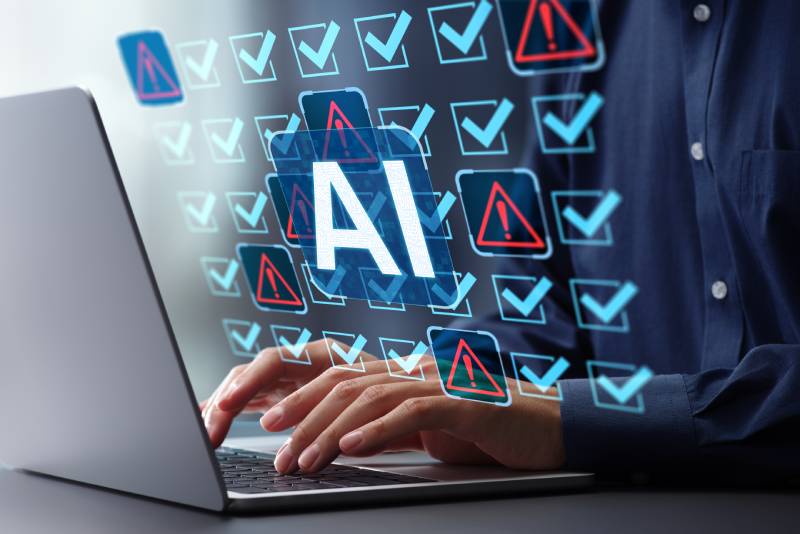
The Rising Need for AI Security in Managed Security Services
As we navigate the exciting frontier of artificial intelligence (AI), recent advancements reveal a turning point in how we understand and interact with technology. In a remarkable test at UC San Diego in March 2025, AI was able to pass the Turing Test 73% of the time, outperforming human counterparts during similar assessments. The growth of the generative AI market reached an impressive $36.06 billion in 2024, with projections estimating it could surge to $356 billion by 2030. While this exhilarating pace invites businesses to adopt AI quickly, it also raises significant security concerns that are frequently overlooked.
Understanding Shadow AI Vulnerabilities
One of the emerging security challenges is the concept of shadow vulnerabilities, which are risks found in open-source AI libraries and models. Unlike traditional vulnerabilities, these flaws often lack Common Vulnerabilities and Exposures (CVE) identifiers, making them difficult to detect with standard security tools. When organizations prioritize rapid deployment over robust security measures, they inadvertently create gaps that sophisticated attackers can exploit.
Sophisticated attacks targeting popular AI frameworks, such as PyTorch, Keras, and TensorFlow, highlight the necessity for specialized monitoring and detection strategies that traditional security systems may overlook. Recognizing these vulnerabilities presents a unique opportunity for Managed Security Service Providers (MSSPs) to enhance their offerings.
Key AI Security Risks
The AI security landscape encompasses several high-impact risks, which MSSPs must consider when crafting their service portfolios. The most pressing risks include:
1. Data Breaches and Information Exposure
AI systems can be susceptible to adversarial inputs that exploit weaknesses in APIs. Security incidents have surfaced, where misconfigured systems led to sensitive data leaks from AI chatbots. For instance, in March 2025, researchers identified 117 systems leaking private conversations due to vulnerabilities. Common scenarios for data breaches linked to AI systems include data scraping and unsecured training data.
Recommended Action: MSSPs should conduct regular tests on AI endpoints to identify misconfigurations and possible data leakage. Expanding Data Loss Prevention (DLP) measures to include interactions with AI services is also essential.
2. Resource Hijacking (LLMjacking)
LLMjacking involves the unauthorized misuse of AI infrastructure for unintended purposes, such as cryptomining, which raises operational costs and disrupts performance. This vulnerability can stem from exploiting zero-day vulnerabilities or hijacking AI model capabilities.
Recommended Action: MSSPs should track unusual API call volumes and establish baselines for normal AI behavior to quickly identify irregularities.
3. AI-Enabled Social Engineering
The rise of AI-powered phishing attacks shows how social engineering tactics have evolved. Reports from 2023 to 2024 indicated a staggering 1200% increase in AI-driven phishing attempts. The use of deepfake technology has made scams more convincing, as evidenced by a $25 million loss linked to a fraudulent video call.
Recommended Action: MSSPs can enhance cybersecurity awareness by providing training on recognizing deepfakes and AI-crafted phishing attempts.
4. Supply Chain Risks in AI
The reliance on third-party AI components can introduce significant risks if those elements harbor hidden flaws or malicious backdoors. Attackers often target trusted frameworks and plugin systems, where a single compromise can cause widespread disruption.
Recommended Action: MSSPs should assess the security of third-party AI tools through routine evaluations and ensure sandboxing practices for open-source libraries to minimize damage.
Strategic Approaches for MSSPs
1. AI-Specific Runtime Monitoring
Implement continuous monitoring solutions for AI systems to identify unusual behaviors that may indicate attacks. This could include special tools tailored to the unique telemetry generated by AI systems.
2. Supply Chain Security Assessment
Develop capabilities to evaluate the security measures of third-party AI components used in client environments. This includes assessing libraries and frameworks for vulnerabilities proactively.
3. AI Incident Response Plans
Establish customized incident response playbooks designed specifically for AI-related security incidents. MSSPs can leverage insights gained from across multiple clients to detect emerging threats early.
4. Continuous Threat Intelligence Gathering
Set up dedicated research teams focusing on emerging threats in AI. These teams can monitor open-source platforms for rogue models and inform proactive threat hunting and detection strategies.
5. Regulatory Guidance
Act as trusted advisors to clients navigating the regulatory landscape surrounding AI security. Help them comply with frameworks such as the EU AI Act while aligning their AI model documentation accordingly.
The MSSP Advantage
With the increasing complexity of AI systems, MSSPs have a prime opportunity to fill the gap in organizations lacking in-house expertise on AI risks. While nearly 80% of IT leaders express confidence in their preparedness for AI challenges, only about half of practitioners share this viewpoint.
MSSPs that prioritize AI security as part of premium service offerings can leverage their cross-client visibility to identify trends and vulnerabilities within AI systems. The forecast for the global AI cybersecurity market indicates it may expand from $22.4 billion in 2023 to over $133.8 billion by 2030, establishing a clear path for forward-thinking MSSPs to lead in cybersecurity advancements.