Leveraging Artificial Intelligence to Aid in Classifying Glandular Tissue Components in Dense Breast Ultrasounds
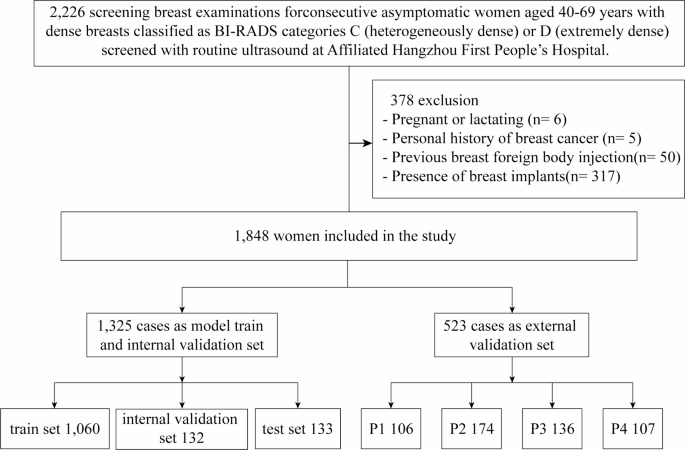
Study Sample
Overview of the Study
The study received approval from the Ethics Committee of Hangzhou First People’s Hospital (Permission Number IIT20240527-0175-01) and adhered to the principles outlined in the Declaration of Helsinki. Conducted at the Affiliated Hangzhou First People’s Hospital, School of Medicine, Westlake University, the research focused on ultrasound data collected from 2,226 healthy women. The data collection occurred between June 1st and November 25th, 2024.
Inclusion and Exclusion Criteria
Participants in the study were women aged between 40 and 69 years who met specific criteria. They needed to show a fibroglandular density classified as ACR BI-RADS category C or D in mammograms, as confirmed by two experienced radiologists. The study only included images that showed clear glandular structures and had no noticeable lesions such as cysts, nodules, or calcifications. Certain participants were excluded from the study, including those who were pregnant or nursing, had a history of breast surgery, had breast implants, or produced low-quality images. All participants voluntarily agreed to take part in the study and their personal information was anonymized.
Image Acquisition
Equipment Used for Imaging
Ultrasound images were gathered using numerous advanced devices, including Siemens S2000 and S3000, as well as equipment from Philips, Samsung, GE HealthCare, and Mindray. Each device employed high-frequency linear array probes tailored for optimal imaging performance.
Imaging Protocol
The ultrasound imaging adhered to a standardized protocol. Participants were positioned supine with their arms raised to expose their breasts fully. A layer of coupling gel was applied, and imaging was conducted radially from the edge where glandular tissue met surrounding fat, starting from two centimeters away from the nipple. The aim was to select images with clear glandular structures while avoiding the nipple and areola areas.
Image Interpretation
Assessment by Radiologists
The accuracy of the image classification was ensured by three senior radiologists, each possessing over a decade of experience in breast ultrasound diagnostics. These professionals underwent specialized classification training and reviewed over 50 cases before evaluating the study images. Two of the radiologists labeled the ultrasound images through a systematic process that involved determining the percentage of glandular tissue and classifying the images into four categories based on this metric.
Ensuring Data Integrity
To maintain the integrity of the results, the dataset was organized such that there was no overlap of patient data across subsets. A double-blind approach was adopted during the labeling process, allowing a third radiologist to resolve any discrepancies. The final labeled data set was formatted uniformly for model training and validation.
AI System
Training of the Model
Based on the annotated dataset, two deep learning models were developed: one for image classification and the other for segmentation. The classification model used ResNet101 to improve feature extraction and tackle common issues in deep learning networks. Various preprocessing steps, such as grayscale conversion and data augmentation, aided the model in enhancing image quality before training.
Loss Functions and Optimization Techniques
During training, the cross-entropy loss function was utilized to measure classification errors. An early stopping strategy was implemented to avoid overfitting, ensuring the model retained its optimal state throughout the process. For segmentation tasks, a combination of ResNet and Fully Convolutional Network (FCN) techniques was applied to achieve accurate pixel-level segmentation.
Reader Selection and Evaluation
Validation Process with Radiologists
External evaluation of the model involved six radiologists, divided into two groups based on their specializations in breast imaging. Prior to reviewing the images, the radiologists underwent training to ensure consistent assessments. They conducted evaluations independently initially, followed by assessments using the proposed AI system after a month.
Statistical Analysis
Metrics for Performance Evaluation
The performance of the AI system was contrasted with that of radiologists using three key metrics: sensitivity, specificity, and positive predictive value. Data analysis was performed using SPSS software, focusing on descriptive statistics and intergroup comparisons through various statistical tests. The classification model’s performance was evaluated using Receiver Operating Characteristic (ROC) curves and the associated area under the curve (AUC), providing insights into its predictive capabilities.
This comprehensive approach combined with rigorous methods ensured the study’s findings on breast ultrasound imaging and classifier performance were reliable and actionable.