Meta AI Framework for Disaster Prevention: Enhanced Prediction of Landslide Susceptibility
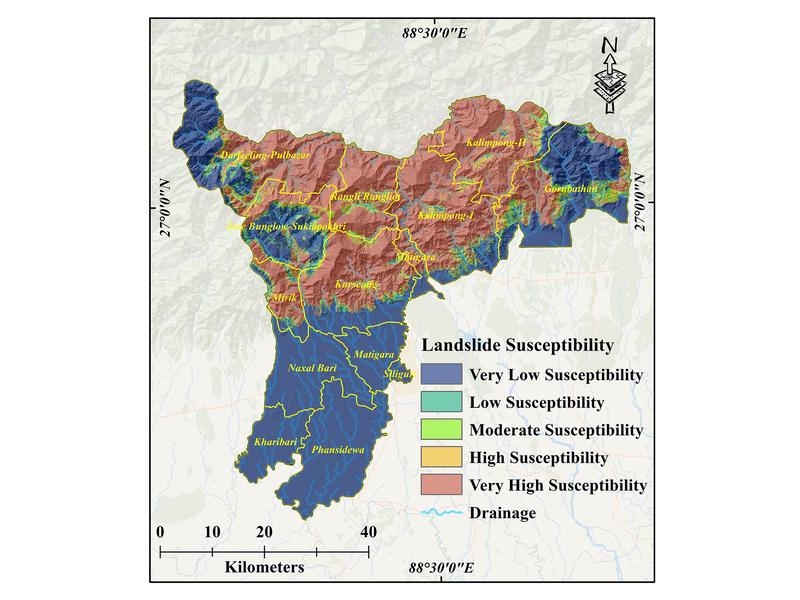
Understanding the New Advances in Landslide Prediction
The Importance of Landslide Prediction
Landslides are a major threat to communities and ecosystems across the globe. They can happen suddenly, leading to devastating consequences for people and the environment. In West Bengal’s northernmost region, researchers at the Leibniz Centre for Agricultural Landscape Research (ZALF), in collaboration with international teams, have created a cutting-edge framework that significantly enhances the prediction of landslides. This innovative model utilizes machine learning techniques, achieving an impressive 95.6% accuracy in identifying landslide risks. This research has been published in the scientific journal Scientific Reports.
How the Prediction Model Works
To effectively predict landslide risks, the model employs a combination of six different machine learning techniques. A simple analogy to illustrate this is comparing it to weather forecasting. Just as meteorologists analyze previous weather patterns and various indicators—like dark clouds and wind speed—to forecast the weather, this model examines environmental data to predict landslide occurrences.
Key Data Factors in Landslide Prediction
The model processes a vast set of environmental variables, including:
- Rainfall Levels: Identifies regions that receive significant precipitation.
- Soil Composition: Determines the stability of the soil, whether it is loose or consolidated.
- Landscape Slope: Measures the steepness of terrain, as steeper terrains are more likely to experience landslides.
- Vegetation Cover: Evaluates plant life that can support soil stability, noting areas that have experienced deforestation.
- Human Activities: Considers the impact of roads, buildings, and other developments that may compromise soil stability.
By analyzing this data in conjunction with past landslide events, the model can recognize patterns that point to areas at high risk.
The Learning Process of the Model
The model’s effectiveness is enhanced through a technique known as a meta-classifier, which integrates the predictive strengths of various machine learning models. The process consists of three major steps:
- Training Base Models: Several machine learning algorithms, such as Logistic Regression, Support Vector Machine, and Random Forest, are independently trained on the dataset.
- Generating Meta-Features: Predictions from these base models are compiled and used as input for further analysis.
- Training the Meta Classifier: A final classifier consolidates the predictions of the base models, improving decision-making accuracy.
According to Dr. Amit Kumar Srivastava and Mr. Krishnagopal Halder from ZALF, this new model allows for a far more accurate identification of areas prone to landslides.
Significance of the New Model
The ability to anticipate landslides can lead to proactive measures that protect communities. Traditional risk assessment tools tend to be slow or unreliable, but this new method analyzes extensive datasets quickly without sacrificing precision. The researchers specifically tested this model in West Bengal’s Sub-Himalayan region, an area frequently impacted by landslides, identifying that high-risk zones often correspond with heavy rainfall, unstable geological structures, and activities like urbanization and deforestation.
With this technology, authorities and disaster management teams can take timely action to safeguard vulnerable regions and provide warnings to those at risk.
Future Applications and Collaborative Research
While the primary focus has been on predicting landslides, the developed model could also be adapted for forecasting other natural disasters such as floods or land subsidence. Future adaptations may expand its usage globally, allowing for more widespread protection against natural hazards.
The project engaged several partners, including institutions like the University of Bonn and Columbia University, alongside various Indian universities. Financial support came from the German Research Foundation and the BMBF project BonaRes, highlighting the global collaboration in advancing natural disaster prediction technologies.
Research Acknowledgments
This research was articulated in the paper titled "Improving landslide susceptibility prediction through ensemble recursive feature elimination and meta-learning framework." The results are significant for enhancing our understanding of landslide risks and the implementation of improved safety measures in vulnerable areas. The research was published under the Open Access license, allowing broader access to these findings.
For further details, interested parties can reach out to Dr. Amit Kumar Srivastava at ZALF, whose contact information is available for inquiries related to this groundbreaking work.