Meta AI Unveils ParetoQ: A Comprehensive Machine Learning Framework for Sub-4-Bit Quantization in Large Language Models
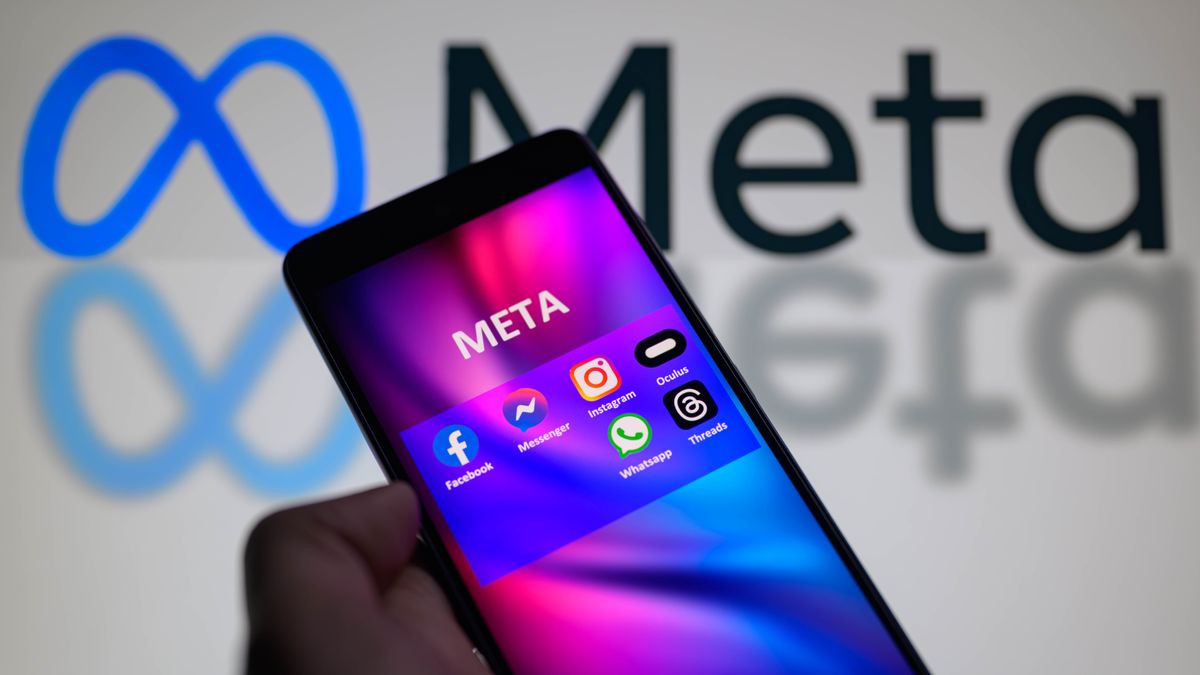
Meta AI’s Introduction of ParetoQ
Meta AI has recently unveiled a groundbreaking machine learning framework known as ParetoQ, which focuses on sub-4-bit quantization specifically for large language models (LLMs). This innovation is significant as it enables more efficient operation without compromising the performance of these models.
What is ParetoQ?
ParetoQ stands out as a unified framework designed to optimize large language models through advanced quantization techniques. Quantization refers to the process of reducing the precision of the numbers used in neural networks, which can lead to significant improvements in computational efficiency and memory usage.
Key Features of ParetoQ
Sub-4-Bit Quantization: This approach allows for the representation of model weights and activations with fewer bits, making it substantially lighter and faster while retaining a high level of performance.
Unified Framework: Rather than using multiple techniques for different aspects of quantization, ParetoQ consolidates these into a single framework, simplifying the process of model development and deployment.
- Efficiency: With ParetoQ, language models can be executed efficiently on lower-end hardware, broadening accessibility and usability for developers and researchers working in various settings.
Significance of Sub-4-Bit Quantization
Sub-4-bit quantization is a critical advancement in the realm of machine learning as it presents numerous advantages over traditional methods:
Advantages
Reduced Computational Cost: With fewer bits to process, models can perform tasks more quickly and with lower power requirements.
Smaller Model Sizes: This quantization leads to a decrease in the overall size of models, enabling them to be stored and transferred more easily.
- Enhanced Model Accessibility: Smaller, more efficient models make advanced machine learning more accessible to institutions and developers with limited resources.
How ParetoQ Works
ParetoQ uses a combination of various machine learning strategies to determine the best way to reduce bits for different components of language models. By analyzing trade-offs between model accuracy and efficiency, the framework allows for a smarter approach to quantization.
Process Overview
Initial Model Training: A language model is trained using standard methods, focusing on performance without any quantization.
Quantization Techniques: Applying ParetoQ’s techniques, the model undergoes quantization to progressively reduce the bit count.
- Performance Evaluation: After quantization, the model’s performance is evaluated to ensure it meets the necessary benchmarks without major losses in quality.
Applications and Future Implications
The introduction of ParetoQ by Meta AI has vast implications for various industries and sectors:
Natural Language Processing: Enhanced models can drive improvements in applications like virtual assistants, chatbots, and translation services.
Healthcare: Streamlined models can facilitate faster data processing, enabling better decision-making in real-time scenarios.
- Edge Computing: Smaller models are ideal for implementing AI solutions on devices with limited processing power, such as smartphones and IoT devices.
Meta’s commitment to researching and developing solutions like ParetoQ underscores a shift towards more efficient and accessible artificial intelligence technologies. The framework not only enhances the performance of large language models but also paves the way for future innovations in the field of machine learning. This development could significantly change how organizations leverage AI, making it possible for a broader range of applications and advancements.