Meta AI Unveils Thought Preference Optimization for Enhanced AI Decision-Making
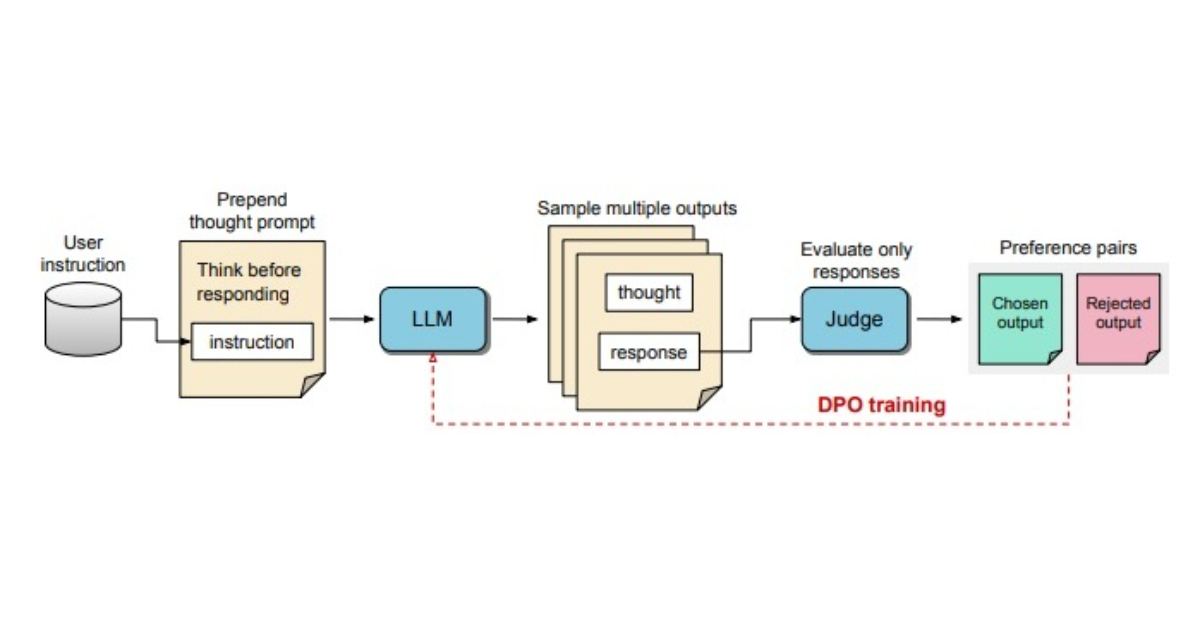
Exploring Thought Preference Optimization in AI
Researchers affiliated with Meta FAIR, the University of California, Berkeley, and New York University have developed an innovative technique named Thought Preference Optimization (TPO). This new method aims to enhance the quality of responses generated by instruction-fine-tuned Large Language Models (LLMs). Unlike conventional models that focus solely on delivering a final answer, TPO encourages LLMs to refine their internal thought processes first, aiming to produce responses that are not only accurate but also coherent.
The Role of Chain-of-Thought Reasoning
TPO builds upon a concept known as Chain-of-Thought (CoT) reasoning. This approach emphasizes that models should "think before they respond." By integrating structured internal reasoning into the training process, TPO helps models develop a well-organized flow of thoughts prior to generating a final answer.
However, traditional CoT prompting has its challenges. It can sometimes lead to lower accuracy rates and is often difficult to implement due to the scarcity of explicit thought steps in instruction datasets. TPO addresses these challenges effectively, as it allows models to optimize their internal thought processes without displaying these intermediate thoughts to users.
How TPO Works
The TPO process involves several steps:
Generating Thoughts: The LLM is prompted to create a range of potential thoughts before constructing a final response.
Evaluation by a Judge Model: Outputs produced by the LLM are then appraised by a judge model. This assessment identifies which responses are the most effective and which are less desirable.
Selection of Response Pairs: The outputs are categorized into chosen and rejected pairs. This forms the basis for Direct Preference Optimization (DPO) training.
- Iterative Training: The model undergoes iterative training cycles where it refines its internal processes based on a streamlined feedback mechanism.
Through this structured approach, the model enhances its ability to generate relevant and quality responses.
Evaluating Effectiveness
The performance of the TPO method is benchmarked against other models, such as Llama-3-8B-Instruct. Initial training starts with a baseline model that does not utilize thought prompting, so TPO gradually optimizes the generation of thoughts iteratively. The results indicate that TPO significantly outperforms these baseline models, showcasing its effectiveness in various tasks.
Notably, the TPO method is versatile, going beyond simple logic and math problems. It proves beneficial for a wide array of instruction-following tasks, including creative fields like marketing and healthcare.
Broader Implications of TPO
The advancements brought by Thought Preference Optimization could have far-reaching implications across multiple sectors. An example can be seen in comments from digital health expert, Dr. Karan Verma, who expressed excitement over how this technique might enhance AI applications in healthcare, potentially leading to better patient outcomes.
The Structural Advantage
The internal thought processes enabled by TPO allow LLMs to handle complex instructions with greater ease. This capability may extend the model’s application in fields that require holistic reasoning and nuanced understanding without needing specific human-generated thought processes.
As TPO evolves, its potential to improve adaptability and effectiveness in LLMs suggests that it could become a fundamental technique for enhancing AI response generation across a multitude of contexts.
By shifting the focus from solely delivering answers to cultivating a thoughtful internal reasoning process, TPO marks a significant advancement in the way artificial intelligence can operate, potentially changing how we interact with technology in our daily lives.