Meta AI Unveils Token-Shuffle: An Innovative AI Method for Decreasing Image Tokens in Transformers
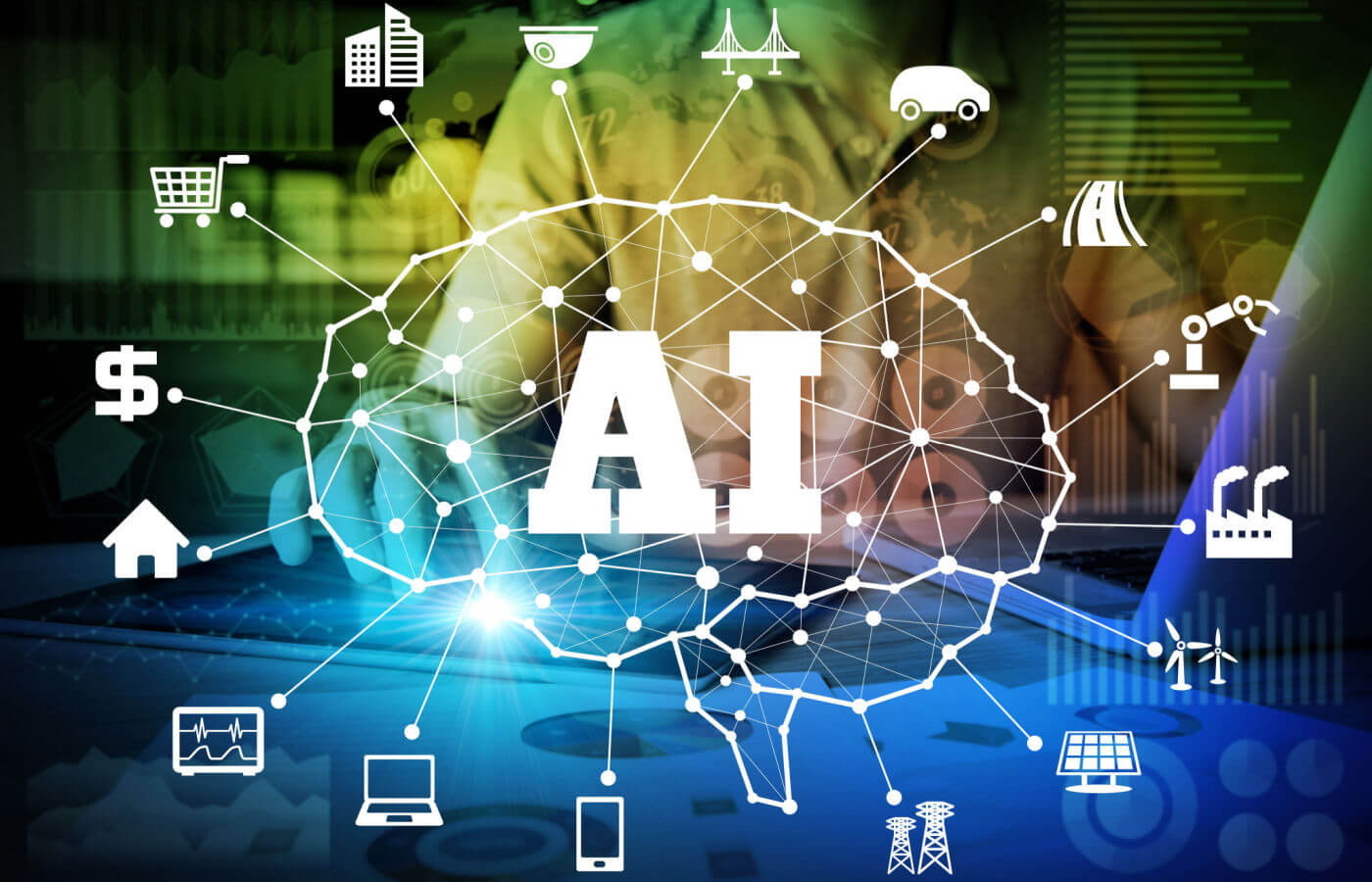
Meta AI Unveils Token-Shuffle: Streamlining Image Processing in AI
Meta AI has recently introduced a groundbreaking technique called Token-Shuffle, designed to enhance the efficiency of image processing in transformer models. This new approach aims to reduce the number of image tokens needed without compromising the quality of the output, thereby optimizing various applications in artificial intelligence.
Understanding the Need for Token Efficiency
In the realm of deep learning, especially when dealing with image data, transformers have emerged as a popular choice. However, these models traditionally process a large amount of data, resulting in significant computational requirements. This is particularly pronounced with image data, where each image is represented as a complex series of tokens.
The challenge is how to manage these tokens effectively. Excessive token usage can slow down processing speed and lead to increased computational costs. Meta AI’s Token-Shuffle technique addresses this specific problem by providing a more efficient way to manage image tokens.
What is Token-Shuffle?
Token-Shuffle is an innovative approach that modifies how image tokens are handled in transformer models. By shuffling tokens during the processing phase, the model can maintain essential visual information while reducing the total number of tokens that it needs to manage. This technique offers several advantages:
Reduced Data Load: With fewer tokens to process, the computational burden on the system is decreased.
Enhanced Processing Speed: Models can generate outputs faster, thereby improving overall performance and user experience.
- Maintained Quality: Despite reduced tokens, the integrity of the image processing remains intact, ensuring that outputs are still high quality.
Key Features of Token-Shuffle
Token-Shuffle comes with several notable features that enhance transformer efficiency in image processing:
Dynamic Token Management: Rather than using a fixed number of tokens, the model can dynamically adjust the token count based on the complexity of the image.
Adaptive Learning: The technique allows the model to learn which tokens hold more significant visual information, thus prioritizing essential features while discarding less useful data.
- Scalability: This method can be integrated into various transformer architectures, making it versatile for different AI applications.
Applications of Token-Shuffle
Token-Shuffle has broad implications across numerous fields that rely on image processing, including:
Computer Vision: From facial recognition to object detection, using fewer tokens can lead to faster and more efficient models.
Medical Imaging: In healthcare, the ability to swiftly analyze imaging data, such as X-rays or MRIs, could enhance diagnosis and patient care.
- Autonomous Vehicles: Improving the efficiency of image processing can aid in real-time decision-making for self-driving cars.
Conclusion
Meta AI’s Token-Shuffle represents a significant advancement in the field of artificial intelligence, particularly in how image data is processed. By reducing the number of image tokens needed while preserving essential visual information, it paves the way for improved performance, reduced costs, and faster technology across various applications. As AI continues to evolve, techniques like Token-Shuffle will play a crucial role in its development and effectiveness.