Meta Tests Its First In-House AI Training Chip to Reduce Costs and Supplier Dependence
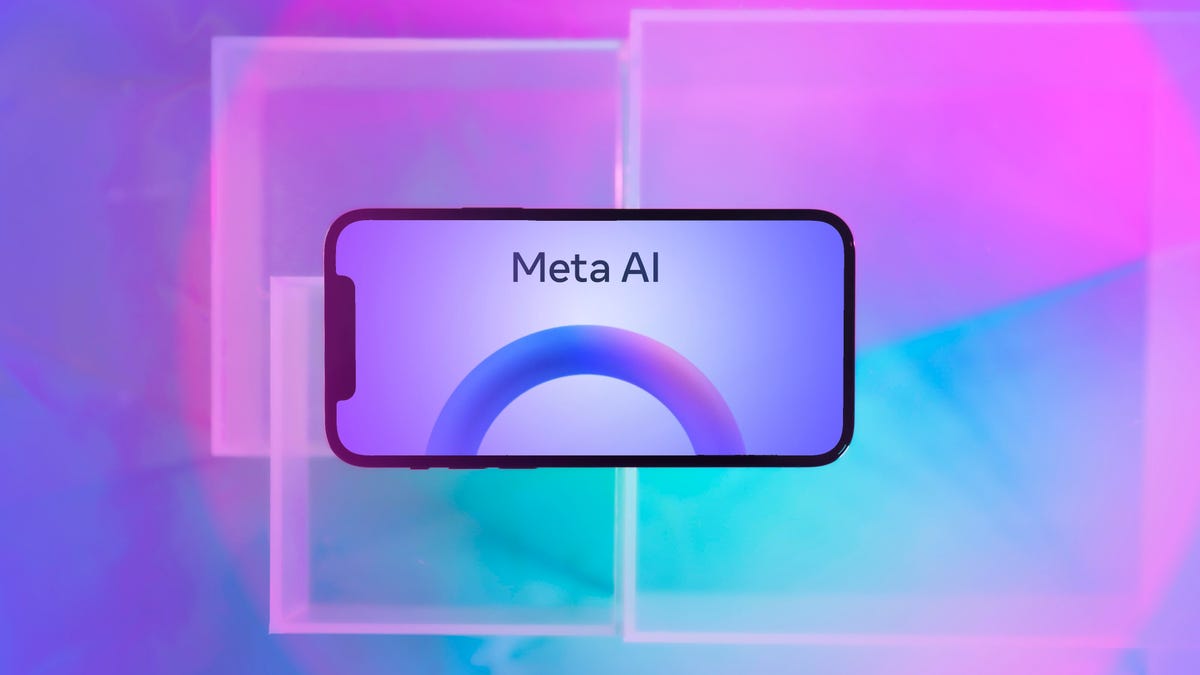
Meta, the parent company of Facebook, Instagram, and WhatsApp, has begun testing its first in-house chip designed for training artificial intelligence (AI) systems. This marks a significant step in Meta’s strategy to develop custom silicon and reduce reliance on external suppliers like Nvidia. The chip is being deployed on a small scale, with plans for broader implementation if testing proves successful.
Key Objectives and Features
- Cost Reduction: Developing in-house AI chips is part of Meta’s long-term plan to lower its massive infrastructure costs. The company has projected 2025 expenses of $114–$119 billion, with up to $65 billion allocated for AI-related capital expenditures.
- Efficiency: The new chip, part of Meta’s “Meta Training and Inference Accelerator” (MTIA) series, is a dedicated AI accelerator designed to handle AI-specific tasks more efficiently than traditional GPUs.
- Applications: Initially, the chip will support recommendation systems, such as those used on Facebook and Instagram. Over time, it may also power generative AI products like chatbots.
Development and Challenges
The chip was developed in collaboration with Taiwan Semiconductor Manufacturing Company (TSMC). Following its first “tape-out”—a critical milestone in chip design—the test phase began. Tape-outs are costly and time-intensive, with no guarantee of success. A failure would require redesigning and repeating the process.
Meta has experienced setbacks in its custom silicon program before. For instance, an earlier MTIA inference chip was scrapped after underperforming in tests. Despite these challenges, Meta successfully deployed a first-generation MTIA chip last year for inference tasks in its recommendation systems.
Future Plans
Meta aims to use its chips for both recommendation systems and generative AI by 2026. Chief Product Officer Chris Cox described the development process as a gradual progression but noted that the first-generation inference chip had been a “big success.”
Competition and Market Context
Meta remains one of Nvidia’s largest customers, relying heavily on GPUs for training models like its Llama foundation series. However, the value of scaling up large language models using GPUs has come under scrutiny as researchers explore more efficient methods.
At the same time, competitors like Google and Amazon are advancing their own custom AI chips. For example:
- Google recently launched its fifth-generation TPU for AI training.
- Amazon has developed multiple custom AI chip families.
Meta’s efforts to develop in-house chips reflect its ambition to achieve greater independence from third-party suppliers while keeping pace with rivals in the rapidly evolving AI landscape.