Meta’s Vanilla Maverick AI Model Falls Short Compared to Competitors in Popular Chat Assessment
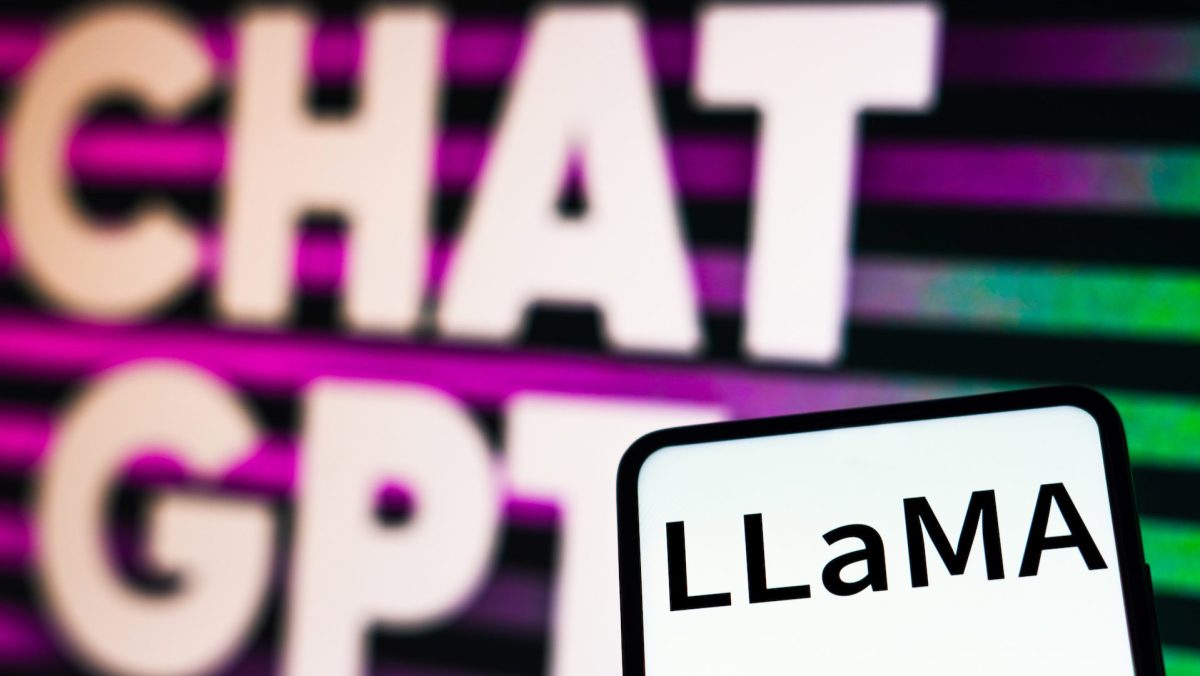
Meta’s Llama 4 Maverick: Behind the Controversy
Recently, Meta found itself at the center of controversy due to its handling of the Llama 4 Maverick model. The company used an experimental version of this model to achieve impressive scores on the LM Arena benchmark, which raised eyebrows and led to significant changes in how the benchmark evaluates AI models.
What Happened?
Meta utilized an unreleased variant known as Llama-4-Maverick-03-26-Experimental, which helped the company secure a high score on LM Arena—an online platform that assesses AI performance through crowdsourced evaluations. However, this move was criticized as misleading, prompting the LM Arena maintainers to apologize and alter their assessment policies. They decided to score only the unmodified version of Maverick, which turned out to be less effective than initially thought.
Performance Comparison
The standard version, Llama-4-Maverick-17B-128E-Instruct, ranked below many established models. As of the latest updates, it stood in 32nd place behind notable competitors like:
- OpenAI’s GPT-4o
- Anthropic’s Claude 3.5 Sonnet
- Google’s Gemini 1.5 Pro
These rivals are not only well-known but had also been released several months prior to the tests conducted with Llama 4.
Reasons for Low Rankings
One might wonder why the unmodified version did not perform as well. According to Meta, the experimental version was designed to be “optimized for conversationality.” This means it was tailored to perform better in interactive settings, which helped it excel in the LM Arena’s format, where human raters compare outputs based on their preferences.
However, this focus on optimization for a specific benchmark introduces potential downsides. For one, it may distort the actual capabilities of the model in various practical applications. Benchmarks should ideally provide insights into how an AI performs in a range of scenarios, not just in highly controlled conditions.
The Role of LM Arena
LM Arena has faced criticism in the past for not being a fully reliable indicator of AI performance. Its unique method of evaluation, involving human raters, can lead to inconsistent results. The controversy surrounding Meta’s use of an experimental model highlighted this issue, as it showcased how tailored models might fare well in a competitive environment but could fall short in real-world applications.
Meta’s Response
Responding to the backlash, a Meta spokesperson stated that the company engages in various experimentation strategies, including the development of custom variants. This experimental version of Llama 4, they noted, was specifically optimized for chat scenarios, which inadvertently contributed to its favorable rankings on LM Arena.
The spokesperson expressed enthusiasm for the open-source release of Llama 4, emphasizing the potential for developers to explore customizable options based on their unique needs. Meta is eager to gather feedback from these developments and anticipate the innovative applications that might arise.
Implications of the Incident
This incident raises important questions about the ethics of AI benchmarking and model development. It emphasizes the necessity for clear and accurate representations of AI capabilities, as the competitive landscape becomes increasingly crowded. Developers, researchers, and users need to navigate this evolving field with caution, ensuring that evaluations reflect true and practical performance rather than benchmark-specific advantages.
As companies like Meta push the boundaries of AI technology, ongoing discussions will be crucial in shaping transparent and effective standards for evaluating artificial intelligence performance.