New ChatGPT Models by OpenAI Reportedly Exhibit Increased Hallucination Frequency
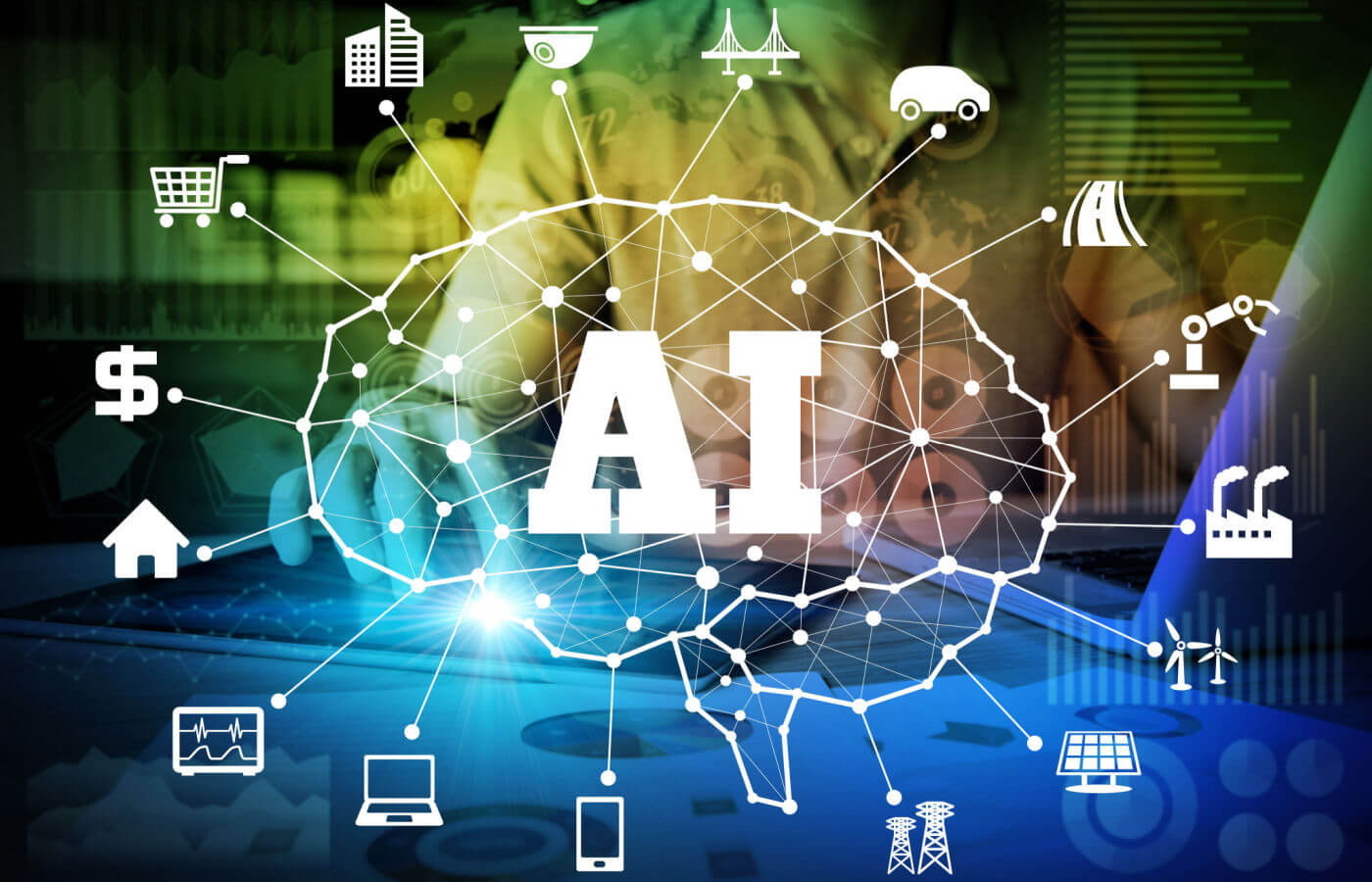
Understanding AI "Hallucinations" in ChatGPT Models
Artificial intelligence is evolving at a rapid pace, and recent advancements in models like ChatGPT from OpenAI have sparked significant interest and scrutiny. One of the more intriguing phenomena observed in these AI models is their tendency to "hallucinate." This term refers to the generation of incorrect or nonsensical information, even when the AI is asked for factual data. Let’s delve deeper into this topic to demystify the concept of hallucinations in AI.
What are AI Hallucinations?
AI hallucinations occur when a model produces outputs that are either completely false or misleading. Unlike human imagination, which can be creative yet grounded in reality, AI hallucinations stem from the model’s training data and algorithms working together in unintended ways.
Characteristics of AI Hallucinations:
- Inaccuracy: The information provided may seem plausible but is factually incorrect.
- Confidence: The model often presents these inaccuracies with a high degree of confidence.
- Context Misunderstanding: Hallucinations can arise from the model misunderstanding the context of a question or prompt.
Why do AI Hallucinations Happen?
Several factors contribute to the occurrence of hallucinations in AI models like ChatGPT:
Training Data: AI models are trained on large datasets that include diverse types of information. If the underlying data is erroneous or biased, the model can replicate these inaccuracies.
Language Patterns: AI primarily learns patterns in language rather than factual information. As a result, it can generate sentences that sound correct but lack truthful content.
Complex Queries: When faced with complex or ambiguous questions, the model may not have enough context to provide a correct answer, leading to hallucination.
- Limitations in Reasoning: Current AI lacks true reasoning abilities. So, it cannot always cross-reference or validate information like a human might.
Examples of AI Hallucinations
Here are a few common examples that illustrate how AI can hallucinate:
- Historical Events: When asked about a specific historical event, the model might fabricate dates or details.
- Scientific Facts: It may misinterpret technical jargon and provide incorrect scientific explanations or definitions.
- Personal Information: In cases where it generates fictional feedback about individuals, it can mistakenly create entire narratives based on incomplete or incorrect data.
Addressing Hallucinations in AI
Dealing with AI hallucinations is crucial for developers and users alike. Here are some strategies being implemented to minimize this phenomenon:
Enhanced Training: Continuous updates and refinements to training datasets help improve accuracy.
Fine-Tuning Models: By adjusting the model’s parameters, developers can instruct it to focus more on factual accuracy rather than merely generating text.
- User Feedback: Incorporating user feedback into the system helps create a more robust AI. When users highlight inaccuracies, these can be used as learning points for future model training.
The Future of AI and Hallucinations
As AI continues to advance, understanding and mitigating hallucinations will be a key area of focus. Researchers are exploring ways to enhance AI’s grasp of context and fact verification, striving for a system that offers reliable information.
Ultimately, acknowledging AI hallucinations is part of creating responsible AI technologies. By maintaining awareness of these limitations, we can better harness the potential of AI while minimizing misinformation.