New Standard for Evaluating the Research Abilities of AI Agents
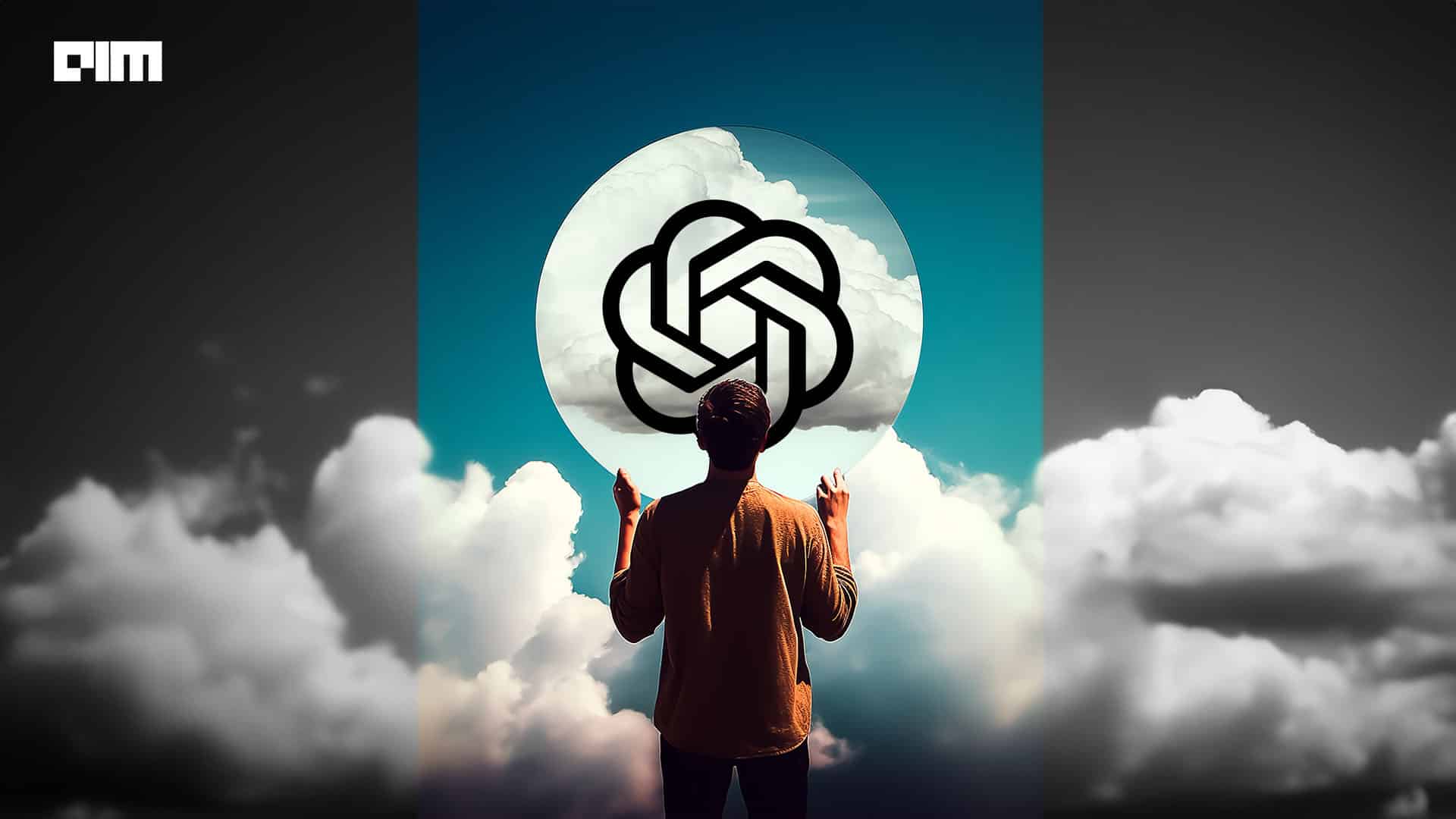
OpenAI Introduces PaperBench for AI Research Performance Evaluation
OpenAI has launched a new benchmarking tool called PaperBench aimed at assessing how effectively AI models can interpret and reproduce findings from leading AI research. This innovative benchmark evaluates an AI’s ability to comprehend research papers, generate the corresponding code, and execute that code to validate the results outlined in the papers.
Overview of PaperBench
PaperBench utilizes 20 influential papers from the International Conference on Machine Learning (ICML) 2024, which encompass a range of 12 different topics. The benchmark consists of 8,316 tasks that can be graded individually. To ensure accurate assessment, a grading rubric was developed in collaboration with the authors of the respective ICML papers. This system breaks down tasks into smaller components with clear criteria for evaluation, aiming to provide a realistic measurement of an AI’s capabilities.
Requirements and Implementation
For AIs to succeed in the PaperBench evaluation, they must extract pertinent information from the provided papers and upload all necessary code to a designated repository. In particular, they are required to create a script named reproduce.sh
, designed to facilitate the execution of the code and ideally reproduce the results highlighted in the referenced papers.
An AI judge has been established to conduct the evaluations, which OpenAI claims closely resembles a human evaluator in accuracy. According to their research, the AI judge, utilizing a model identified as o3-mini-high with custom scaffolding, recorded an impressive F1 score of 0.83 during auxiliary evaluations. This score suggests that the AI’s accuracy is comparable to that of human judges.
Performance of AI Models in PaperBench
Various AI models were subjected to testing within the PaperBench framework. The standout performer was Anthropic’s Claude 3.5 Sonnet, achieving a 21% replication score. Other models, such as OpenAI’s o1, GPT-4o, Gemini 2.0 Flash, and DeepSeek-R1, demonstrated lower scores.
In comparison, human experts with PhDs in machine learning attained an average score of 41.4%, emphasizing that current AI technology still has a significant way to go before rivaling human-level performance in understanding and reproducing advanced AI research.
Additional Testing Insights
Further assessment was made with OpenAI’s o1 model over an extended duration, which ultimately also fell short of matching the performance of human experts. This persistent gap between AI capabilities and human expertise sheds light on the challenges faced in achieving greater advancements in AI research comprehension.
Open Access to PaperBench Resources
The complete code for PaperBench, along with its findings, is publicly accessible on GitHub. To encourage broader participation, OpenAI has also released a lighter version called PaperBench Code-Dev, allowing more individuals to engage with the benchmark without extensive technical requirements.
By launching PaperBench, OpenAI not only provides a novel framework for evaluating AI’s capabilities but also highlights ongoing gaps between AI and human performance, paving the way for future research and developments in the AI field.