Noam Brown, research lead at OpenAI, believes that some AI ‘reasoning’ models could have been developed decades earlier.
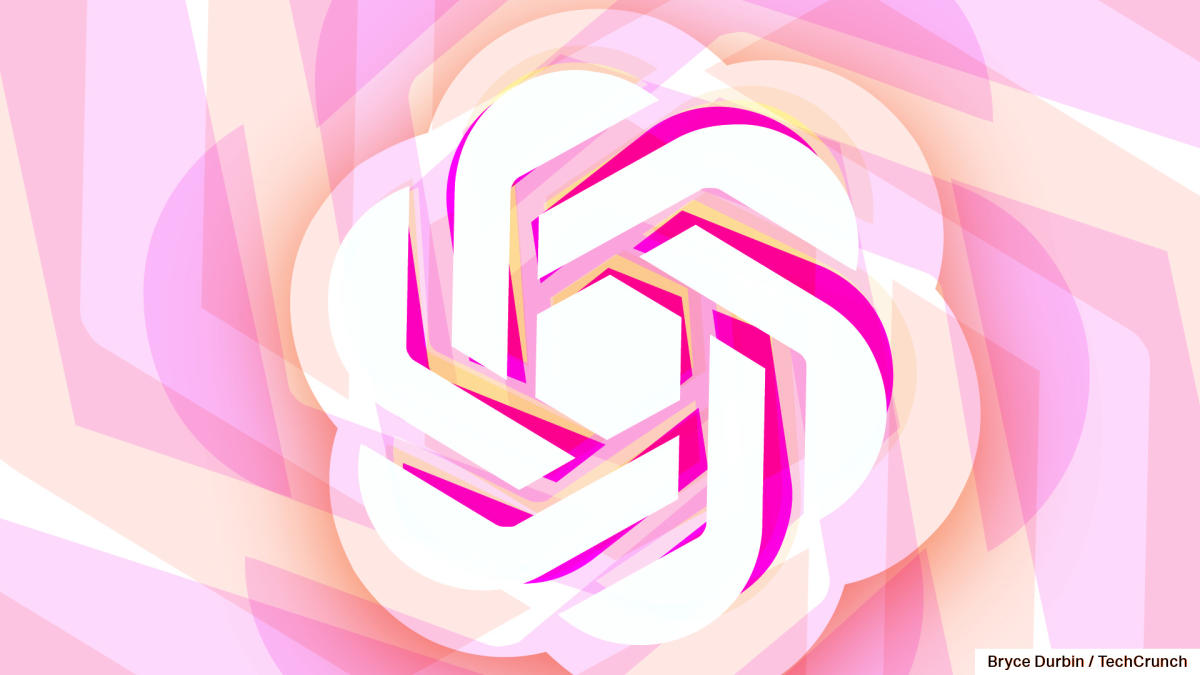
Understanding AI Reasoning According to Noam Brown
The Role of AI Reasoning Research
Noam Brown, who leads AI reasoning research at OpenAI, highlights that the development of "reasoning" AI models could have taken place two decades ago if researchers had followed the right methods and algorithms. He made these remarks during a panel discussion at Nvidia’s GTC conference in San Jose, where he discussed the potential for AI to imitate human thinking processes.
The Neglected Need for Reasoning
Brown pointed out that researchers overlooked crucial aspects of AI development for various reasons. He noted, “Humans spend a lot of time thinking before they act in a tough situation. Maybe this would be very useful in AI.” This revelation has led him to explore how AI can be designed to think critically in complex scenarios.
Game-Playing AI: A Case Study
One of Brown’s notable contributions is in the realm of game-playing AI at Carnegie Mellon University. He played a significant role in the creation of Pluribus, an AI model that successfully defeated skilled human poker players. Unlike traditional AI methods that relied heavily on brute-force calculations, Pluribus utilized a reasoning approach. This method allowed it to analyze situations more effectively, showcasing the potential of reasoning-based AI.
Innovative Techniques in AI Development
In addition to his work with Pluribus, Brown is a key architect behind the OpenAI model known as o1. This model incorporates a technique called test-time inference, which enables it to "think" before formulating responses. Test-time inference requires extra computational power to enhance the model’s reasoning abilities. As a result, reasoning models like o1 have shown to be more accurate and reliable in fields such as mathematics and science compared to traditional AI models.
The Challenge for Academia in AI Research
During the panel discussion, Brown commented on the difficulties faced by academic institutions when it comes to conducting large-scale AI experiments. He acknowledged that as AI models have grown more complex and computing-intensive, it has become harder for universities to keep pace. However, he also pointed out that academics can still contribute significantly by focusing on areas that require less computational power, such as model architecture design.
Opportunities for Collaboration
Brown emphasized that there is a chance for collaboration between leading AI labs and academic institutions. He stated, “Frontier labs are looking at academic publications and thinking carefully about compelling arguments that, if scaled up further, could be very effective.” This opens the door for academia to work alongside industry experts, allowing promising research to be evaluated for practical applications.
Impact of Funding Cuts on AI Research
Amidst a backdrop of significant funding cuts to scientific grants under the Trump administration, Brown’s comments take on added urgency. These cuts threaten not only AI research in the United States but also globally, as many experts argue this jeopardizes essential advancements in the field. Renowned figures in AI, like Nobel laureate Geoffrey Hinton, have publicly criticized these budget reductions, asserting they could hinder progress.
Benchmarking in AI
Brown also pointed out that AI benchmarking represents a valuable opportunity for academic research. He remarked, “The state of benchmarks in AI is really bad, and that doesn’t require a lot of compute to do.” Improving benchmarks can greatly enhance the field of AI without the necessity for extensive computational resources, making it an accessible avenue for academic contributions.
By focusing on thoughtful reasoning in AI, the potential for significant advancements in both practical applications and theoretical understanding remains promising. Understanding and addressing these gaps in AI research will be essential for its future development.