One Week at Manus | IBM
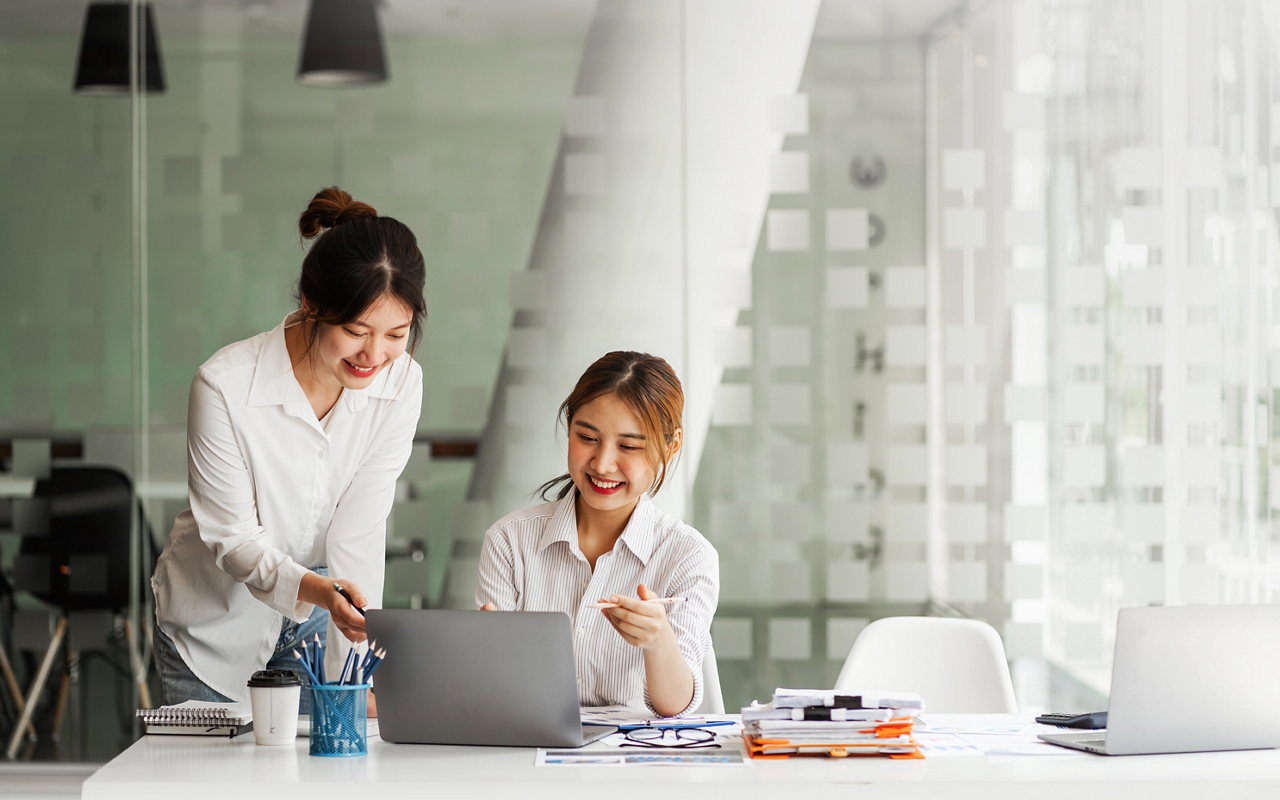
Understanding Autonomous AI Agents in Enterprises
Autonomous AI agents are gaining traction among consumers, but their adoption in businesses may take longer. The current technology primarily operates on Large Language Models (LLMs) that rely on publicly accessible information. However, in enterprise settings, more than 99% of data remains untapped, potentially representing a significant opportunity for companies that leverage these agents effectively.
The Challenge of Data Utilization
Most large language models today are not configured to utilize the vast amount of enterprise data that organizations possess. Only about 1% of this data has been integrated into LLMs, leaving a substantial reservoir of insights and value unexploited. This presents a unique challenge for enterprises looking to use AI agents to transform their operations.
Different Use Cases: Individual vs. Enterprise
The use of AI agents varies significantly between personal use and enterprise application:
Personal Use Cases: Individuals use AI agents from companies like Manus AI or OpenAI for straightforward tasks such as booking flights or editing resumes. These agents are designed for a broad audience and general utility.
- Enterprise Use Cases: Businesses, on the other hand, require AI agents that are either customized for specific purposes or built on proprietary or open-source tools. The focus is often on cost efficiency and security. Companies such as Salesforce have developed agent systems like Agentforce, while IBM offers solutions like Bee AI, which address these unique enterprise needs.
Security Considerations in Enterprise AI
A significant concern for enterprises is data security. Many organizations prefer to keep their sensitive data in-house rather than transferring it to third-party services. Using customized, fit-for-purpose language models, such as IBM’s Granite series, allows businesses to maintain tight control over their data while still harnessing the benefits of AI capabilities.
The Role of New Technologies
The landscape of AI agents is continually evolving, and companies are quickly adapting to new technologies. For instance, Alibaba recently introduced an AI assistant called Qwen 2.5 Max, which employs a mixture of experts (MoE) architecture. This framework activates only specific sub-networks required for particular tasks, enhancing efficiency by minimizing computational costs during both pre-training and inference.
Benefits of MoE Architecture
Cost Efficiency: Since it doesn’t require full activation of the network, the MoE model reduces overall computational expenses.
- Faster Performance: The architecture enables quicker responses, which is vital in a fast-paced business environment.
The Future of AI Autonomy
As for the question of whether true AI autonomy can be achieved through personal or enterprise agents, the debate is ongoing. Experts like Kaoutar El Maghraoui from IBM express skepticism regarding the potential of new models to redefine AI autonomy further. There is concern over whether advancements like Manus will mark a significant shift in AI capabilities or simply contribute to the ongoing competition between leading entities in the field.
In summary, while personal AI agents are currently more popular, the potential for tailored enterprise solutions based on untapped data is immense. As companies continue to explore AI technologies, the focus will likely remain on how these innovations can be effectively implemented while addressing security and operational needs.