OpenAI Outperforms DeepSeek in Sentence-Level Reasoning
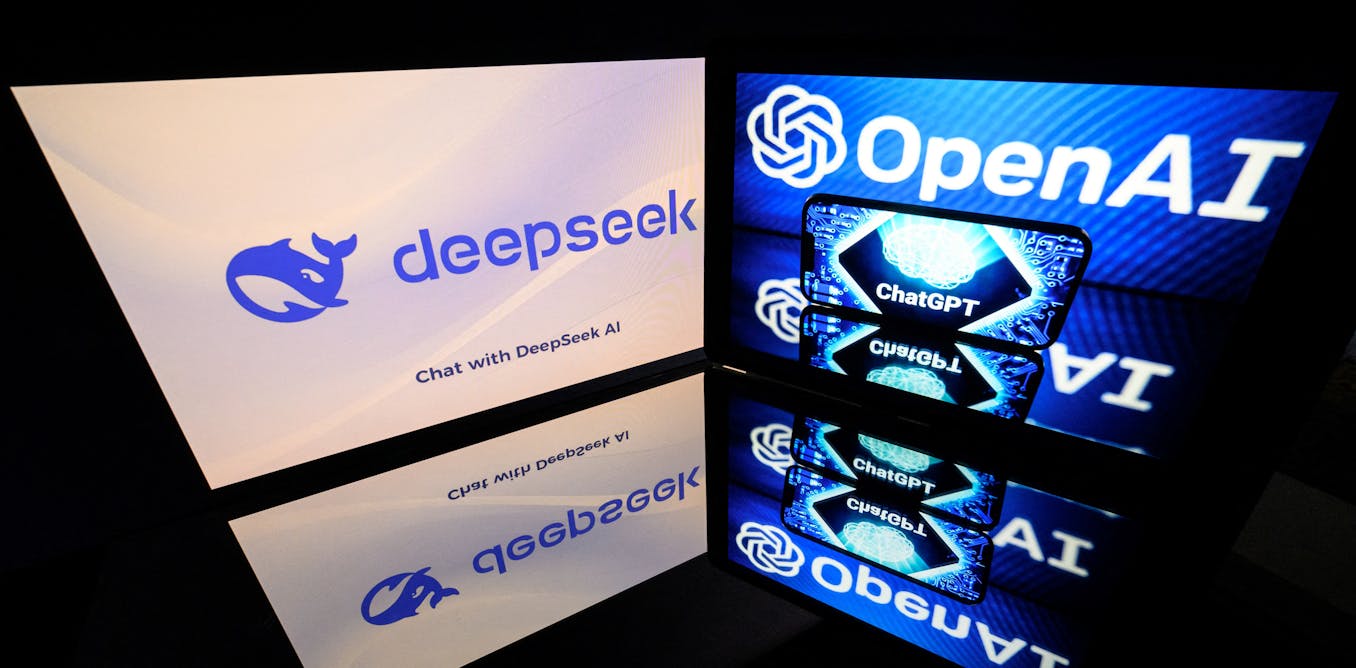
Understanding AI Models and Citation Accuracy
AI chatbots, particularly those like ChatGPT, are designed to understand and generate human-like text. However, they sometimes produce inaccurate information, including incorrect scientific and legal citations. Assessing how accurately an AI model can generate citations is an important way to evaluate its reasoning capabilities.
The Reasoning Process of AI
An AI model’s reasoning ability involves analyzing a query step-by-step. This process is similar to how students learn to solve math word problems: breaking down the information and addressing each part systematically.
For the ideal citation generation, an AI would need to grasp the main concepts within a document, create a list of pertinent papers to cite, and explain how each piece of research supports the text. This would include making clear connections between the text and the references, showcasing why each source is relevant.
Trust in Connections and Reasoning
The crucial question arises: can users trust AI models to accurately make these connections and justify their choices? The answer isn’t simple. It extends beyond just citation accuracy to encompass how effectively large language models operate in various information retrieval tasks.
Insights from Research
As a computer scientist, I, along with a team from several academic institutions, developed the Reasons benchmark. This benchmark evaluates how well large language models, such as DeepSeek’s R1 and OpenAI’s o1, can generate research citations and articulate the reasoning behind them.
In our comparisons, we found that while DeepSeek R1 offers impressive efficiency and cost-effectiveness, it still falls short in reasoning accuracy compared to OpenAI’s model.
The Importance of Sentence-Level Analysis
Sentence-Level vs. Paragraph-Level Reasoning
The quality of AI-generated citations largely depends on whether the model analyzes information at the sentence, paragraph, or document level. When citations are derived from larger text segments like paragraphs or documents, the AI can overgeneralize and misinterpret key details in individual sentences.
For instance, using a whole paragraph as a basis for citation can lead to misunderstandings, where the AI addresses overarching themes instead of the specific nuances of a sentence. This issue is compounded when models are asked to analyze lengthy documents, as they often excel at capturing information presented in the introduction or conclusion but struggle with details scattered throughout.
Addressing Limitations
The Reasons benchmark specifically targets these challenges by scrutinizing how well language models generate citations while reasoning through the material.
Comparing AI Models’ Performance
In January 2025, after the launch of DeepSeek R1, we assessed its citation accuracy and reasoning quality against OpenAI’s o1 model. We compiled a small dataset of around 4,100 research articles across four key topics related to neuroscience and computer science, including cognition, human-computer interaction, databases, and AI.
The models were tested on two primary metrics: F-1 score (which gauges citation accuracy) and hallucination rate (which measures how often the model produces misleading or incorrect responses).
Performance Findings
Our findings revealed significant performance variations between OpenAI’s o1 and DeepSeek R1 across numerous scientific areas. OpenAI’s model excelled in linking different subjects coherently while maintaining accuracy. It consistently outperformed DeepSeek R1 in all evaluation metrics, particularly in reducing hallucination rates and completing assigned reasoning tasks successfully.
OpenAI’s o1 also demonstrated better semantic integration of ideas, while DeepSeek R1’s focus on responding to every citation request led to higher rates of incorrect reasoning. The hallucination rates were stark — approximately 35% for o1 compared to nearly 85% for R1.
In terms of statistical accuracy, OpenAI’s o1 showed an F-1 score around 0.65, indicating it was correct about 65% of the time, coupled with a BLEU score of 0.70, signifying its ability to produce natural-sounding text. Meanwhile, DeepSeek R1 scored around 0.35 on the F-1 and only 0.2 on the BLEU test, reflecting less proficiency in natural language generation.
Competitive Landscape in AI Development
While DeepSeek R1 performs comparably to OpenAI o1 in some domains like mathematics and coding, the notable differences in our benchmark highlight that o1 is more reliable in terms of factual accuracy and reasoning integration.
The results reinforce the idea that OpenAI has a distinct edge in citation generation and reasoning capabilities, likely stemming from the diverse and extensive data used in training its models. Recently, OpenAI introduced a new deep research tool that can generate reports accompanied by citations, engage in follow-up questions, and elucidate the reasoning for its outputs.
While the effectiveness of such tools for research needs to be established, one crucial takeaway for users remains: always verify the citations provided by AI models.