OpenAI PaperBench: Pioneering Progress in AI for Machine Learning Research
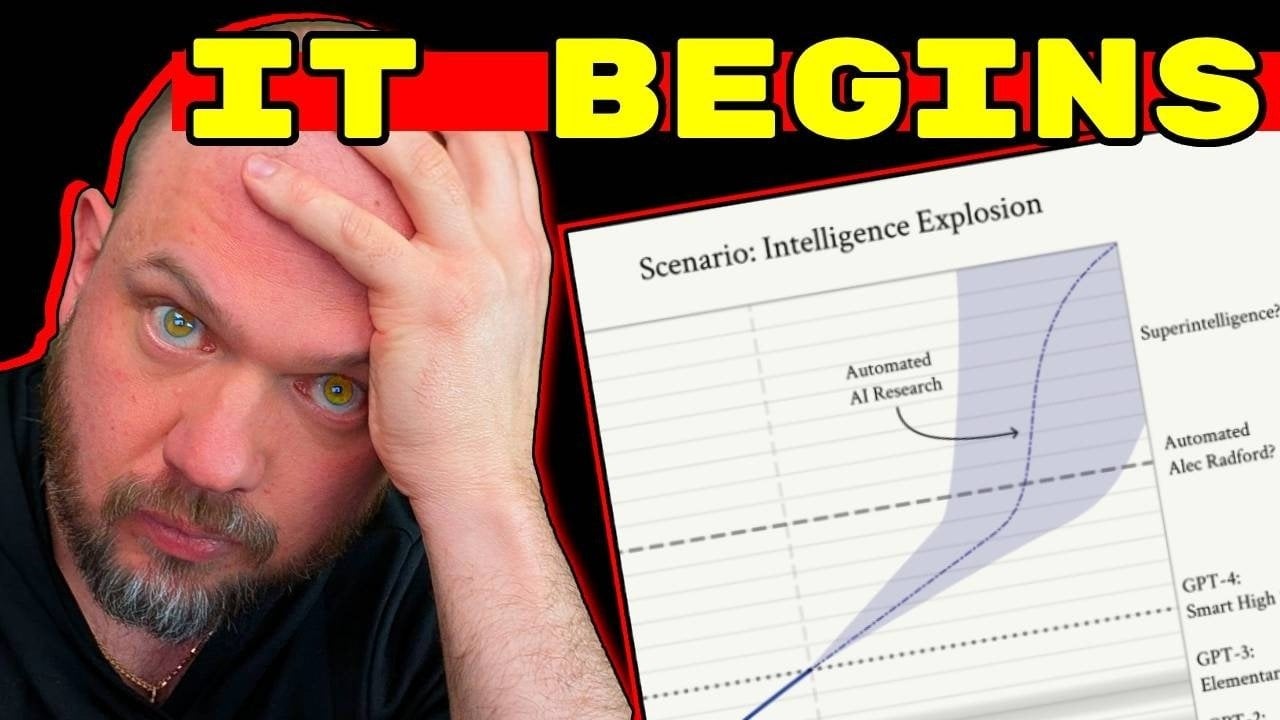
OpenAI has introduced an innovative tool called “PaperBench,” which aims to evaluate how well artificial intelligence (AI) can replicate cutting-edge machine learning research. This tool is part of OpenAI’s broader goal to assess the risks and capabilities of AI systems, especially in high-stakes situations. By testing AI models on their ability to reproduce high-level research papers, PaperBench offers important insights into the strengths and weaknesses of AI in driving scientific progress.
Understanding PaperBench
PaperBench is a benchmarking tool that challenges AI systems to replicate findings from 20 selected machine learning papers from the ICML 2024 conference. The assessments do not involve using existing resources; instead, AI agents must:
- Understand: Grasp the methodologies and content presented in the research papers.
- Develop: Create codebases from scratch.
- Reproduce: Achieve the same experimental results without prior access to the original code or other aids.
This approach places AI in realistic scientific settings, providing a more rigorous evaluation than typical benchmarks, which often focus on isolated or narrow tasks. PaperBench assesses AI’s performance using three main metrics:
- Accuracy: How closely the reproduced results align with the original findings.
- Code Correctness: The quality and functionality of the code produced.
- Experimental Execution: The ability to execute and complete experiments successfully.
Key Insights from PaperBench
1. OpenAI’s PaperBench serves as a way to measure AI’s efficiency in replicating state-of-the-art research.
2. The best-performing AI model managed a replication success rate of 21%, compared to a 41.4% success rate for human researchers.
3. Despite AI’s advancements, it still struggles with intricate problem-solving tasks and complex experiments.
4. The benchmark could face scalability issues due to its reliance on detailed grading rubrics.
5. Ethical concerns arise, particularly around AI’s self-improvement and autonomy.
Challenges Facing PaperBench
While PaperBench reveals valuable insights into AI capabilities, it also experiences some notable challenges:
- Scalability: Dependence on collaboration with paper authors leads to limitations in applying benchmarks across various research areas.
- AI Limitations: Current AI models can struggle with replicating complex experimental setups and lack the nuanced comprehension needed for ongoing problem-solving.
AI’s Position in Scientific Research
The introduction of PaperBench demonstrates how AI could dramatically change scientific research. AI has the potential to speed up discoveries by automating repetitive tasks like replicating experiments and confirming results. Some AI models have even produced research articles that were accepted for peer review, indicating their ability to add value to scientific conversations. However, AI systems are still limited. They often find it difficult to maintain accuracy and adaptability in intricate research contexts, necessitating further development to enhance their reliability.
Comparing AI and Human Researchers
Despite advancements in capability, AI systems have not yet surpassed the performance of human researchers in replicating complex experiments. For instance, in assessments using PaperBench, human researchers achieved a 41.4% success rate while the best AI model achieved 21%. While AI excels in tasks like reading papers and generating basic code, it often stumbles when faced with complex experiments that require prolonged focus and adaptation. This gap illustrates the distinctive expertise and problem-solving abilities human researchers possess, along with areas where AI still has progress to make.
Future Implications for Scientific Discovery
The use of AI in scientific research holds substantial implications for future discoveries. By automating the repetition of experiments and the documentation of findings, researchers can allocate more time to innovative exploration. Nevertheless, this shift also raises ethical issues, particularly concerning AI’s potential for autonomous operation and unforeseen consequences. Responsible governance and ethical considerations are crucial to ensure scientific integrity and prevent the misuse of AI technologies.
Navigating AI’s Future in Research
AI continues to evolve rapidly but remains several steps behind human expertise when it comes to complex scientific tasks. PaperBench plays a vital role in evaluating AI’s present capabilities while identifying potential areas for future enhancement. As AI becomes more embedded in scientific work, it is essential to navigate associated risks while promoting responsible deployment. This benchmark not only assesses AI’s current abilities but also sets the stage for ethically leveraging AI in shaping future scientific advancements.