OpenAI Research Lead Noam Brown Believes AI ‘Reasoning’ Models Could Have Emerged Decades Earlier
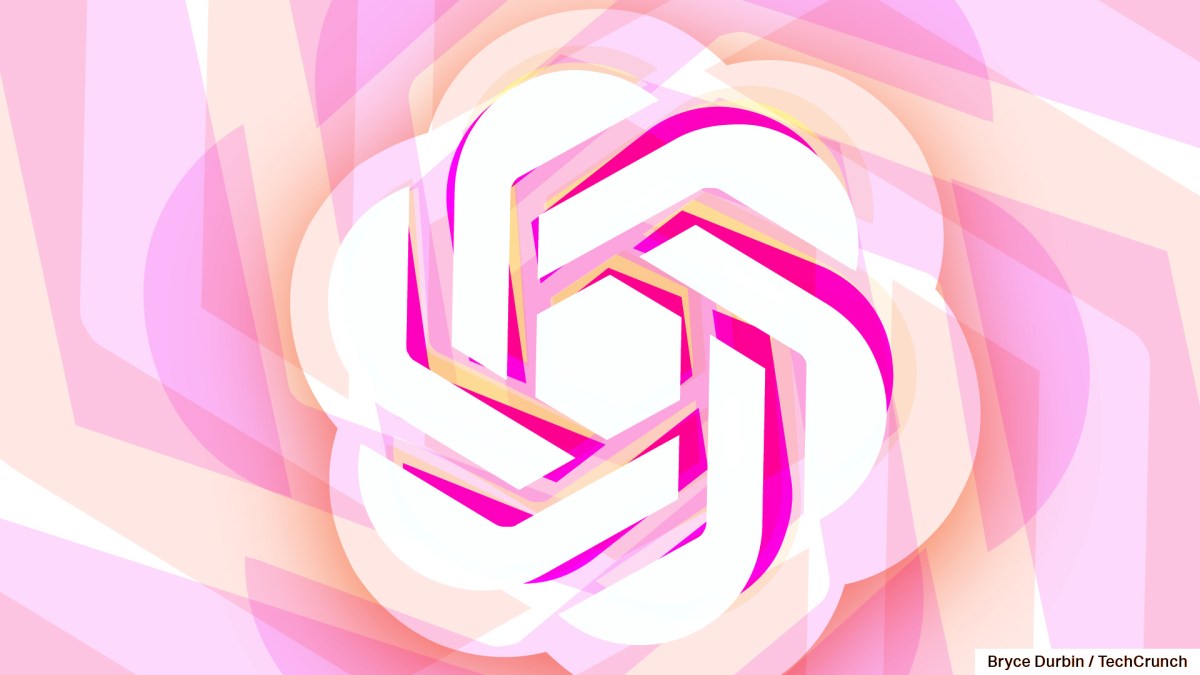
Understanding Reasoning in AI: Insights from Noam Brown
Noam Brown, who is a leader in AI reasoning research at OpenAI, recently expressed that advanced AI models designed for reasoning, such as OpenAI’s o1, could have emerged two decades earlier if researchers had figured out the effective methodologies and algorithms needed. This discussion took place at a panel during Nvidia’s GTC conference in San Jose.
The Necessity of Reasoning in AI
Brown emphasized the importance of "reasoning" in AI, pointing out that humans often take time to deliberate before making decisions in challenging situations. He believes that instilling this trait in AI could enhance its functionality and reliability. Over the years, he observed a gap in current AI research and posited that an approach that mimics human reasoning could be vital for advancements.
Introduction to o1 and Test-Time Inference
The o1 AI model is one of Brown’s major contributions, which uses a method known as test-time inference. This technique allows AI models to employ additional computation when responding to queries, enabling them to "think" before providing answers. As a result, reasoning models like o1 tend to demonstrate higher accuracy and reliability, especially in complex fields like mathematics and science.
Key Features of Test-Time Inference
- Enhanced Accuracy: This method leads to more precise responses.
- Complex Problem Solving: Models can tackle intricate tasks that require more than just surface-level information.
- Improved Reliability: Responses generated are more trustworthy than those from traditional AI models.
The Current Landscape of AI Research
Brown also discussed the state of AI research and the role of pre-training, which involves training models on vast datasets. He assured the audience that while the focus is shifting from pre-training to test-time inference, both approaches remain essential. AI labs, including OpenAI, are now balancing their time between these two methodologies, viewing them as complementary rather than mutually exclusive.
Opportunities for Academia in AI
During the panel, a question arose regarding whether academic institutions could conduct experiments comparable to those undertaken by large AI labs like OpenAI. Brown acknowledged the challenges posed by the increased computational demands of modern AI models. However, he encouraged academics to explore areas that require less computing power, such as designing new model architectures.
Collaboration Potential
- Partnerships: Brown mentioned the possibility of collaborating between AI frontier labs and academic institutions.
- Impactful Research: He pointed out that many laboratories actively look at academic publications and may consider supporting research that shows promise when scaled.
AI Benchmarking: A Critical Area for Improvement
Brown raised significant concerns about the current state of AI benchmarking, claiming it presents an accessible opportunity for academic contributions. He criticized the existing benchmarks, describing them as ineffective and too focused on niche knowledge. This inadequacy leads to misunderstandings about what AI models can actually accomplish.
Problems with Current AI Benchmarks
- Lack of Relevance: Many benchmarks do not assess skills that are relevant to real-world applications.
- Ambiguity in Performance: The scores generated often do not accurately reflect the model’s capabilities.
- Need for Better Standards: Brown highlighted the necessity for improved benchmarks that align more closely with meaningful tasks.
In summary, Noam Brown’s insights illuminate the past, present, and potential future of reasoning in AI, showcasing the ways in which both academia and large research labs can collaborate to advance understanding and effectiveness in this rapidly evolving field.