OpenAI Research Lead Noam Brown Believes Some AI Reasoning Models Could Have Emerged Decades Earlier
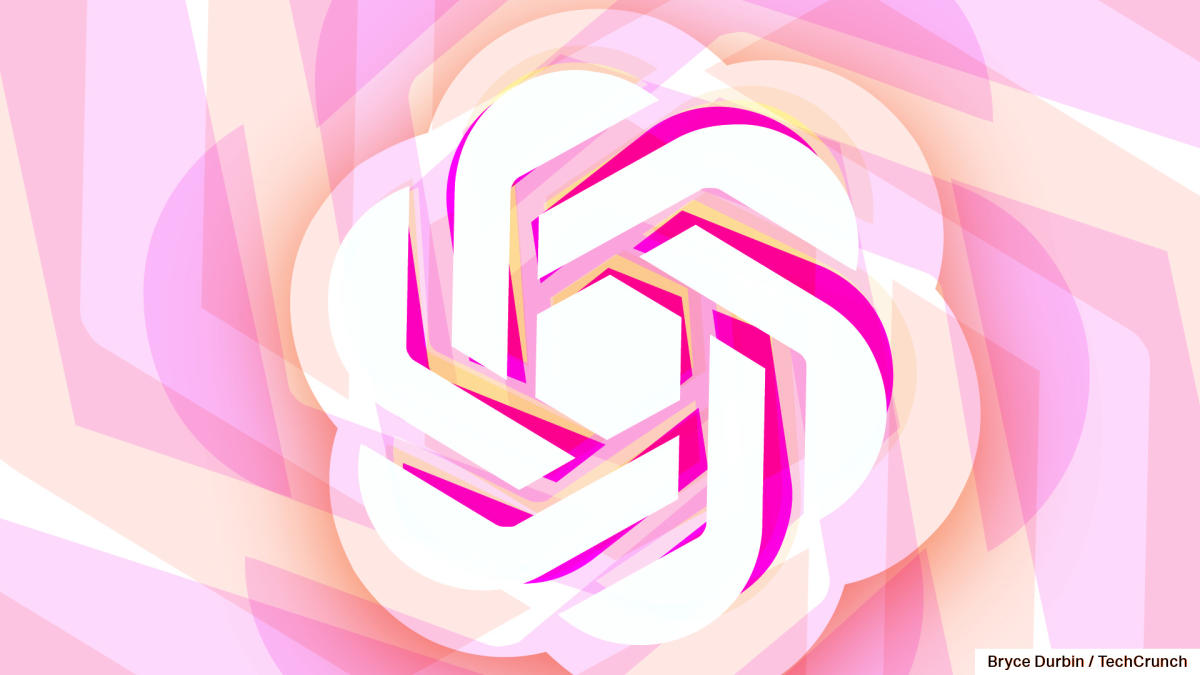
Understanding AI Reasoning and Its Evolution
The Importance of Reasoning in AI
Noam Brown, the head of AI reasoning research at OpenAI, recently highlighted the importance of reasoning within artificial intelligence (AI) models. He stated that certain methods of reasoning in AI could have been developed as early as 20 years ago if researchers had known the correct approaches and algorithms to apply. This assertion points to a missed opportunity in AI development that could have advanced the field significantly.
Brown shared his insights during a panel discussion at Nvidia’s GTC conference in San Jose. He remarked on a realization he had during his research: humans often take significant time to think through challenging situations before making decisions. He believes that introducing a similar reasoning process into AI could greatly enhance its capabilities.
Breakthroughs in Game-Playing AI
Much of Brown’s experience stems from his work at Carnegie Mellon University, where he contributed to the development of advanced game-playing AI systems, such as Pluribus. This AI system was particularly notable for its ability to defeat elite human professionals in poker. Unlike many traditional AI models that relied on brute-force calculations, Pluribus utilized a reasoning approach to tackle complex problems, showcasing how effective reasoning could be in AI applications.
Advancements with OpenAI’s O1 Model
Brown is also one of the key developers behind OpenAI’s O1 model. This model incorporates a process known as test-time inference, which allows it to "think" before responding to queries. Test-time inference adds an extra layer of computational effort that enables the model to engage in deeper reasoning, making it more accurate and reliable, especially in fields like mathematics and science.
Challenges in Academia
During the panel discussion, Brown addressed the challenges academia faces in keeping pace with advancements in AI research due to limited access to computational resources. As AI models grow increasingly intensive in their computing requirements, academic institutions struggle to conduct experiments on the same scale as major AI labs like OpenAI. Despite these challenges, Brown noted that academics could still contribute meaningfully by focusing on areas that require less computational power, such as exploring new model architectures.
He emphasized the potential for collaboration between academic institutions and leading AI labs. Frontier labs often scan academic publications for compelling ideas that could yield effective applications if scaled further. If academics present strong arguments in their research, they might find interest and collaboration opportunities with these advanced labs.
Concerns Over Funding Cuts
Brown’s discussion took place against a backdrop of concerns regarding funding cuts to scientific research, particularly those affecting AI. Recently, the Trump administration faced criticism for deep cuts to scientific grant-making, a move that AI experts, including notable figures like Nobel Laureate Geoffrey Hinton, argue could threaten both domestic and international AI research efforts.
Improving AI Benchmarks
One area where Brown believes academia could make a significant impact is AI benchmarking. He criticized the current state of benchmarks, stating that they are inadequate and don’t necessarily require vast computational resources to improve. Presently, many popular AI benchmarks focus on obscure knowledge, yielding scores that do not accurately reflect a model’s proficiency in tasks that matter to most users. This has led to a widespread confusion about the capabilities and advancements of AI models.
The Future of AI Research
As the AI landscape continues to evolve, the need for improved benchmarks and the incorporation of reasoning mechanisms in AI systems remain vital. With collaborative efforts between academia and prominent AI labs, there is potential for significant advancements in AI research, which could lead to more reliable and accurate AI models that can better assist in various applications across multiple domains.