OpenAI’s Deep Research Demonstrates Superior Fact-Finding Capability Yet Fails Half the Time
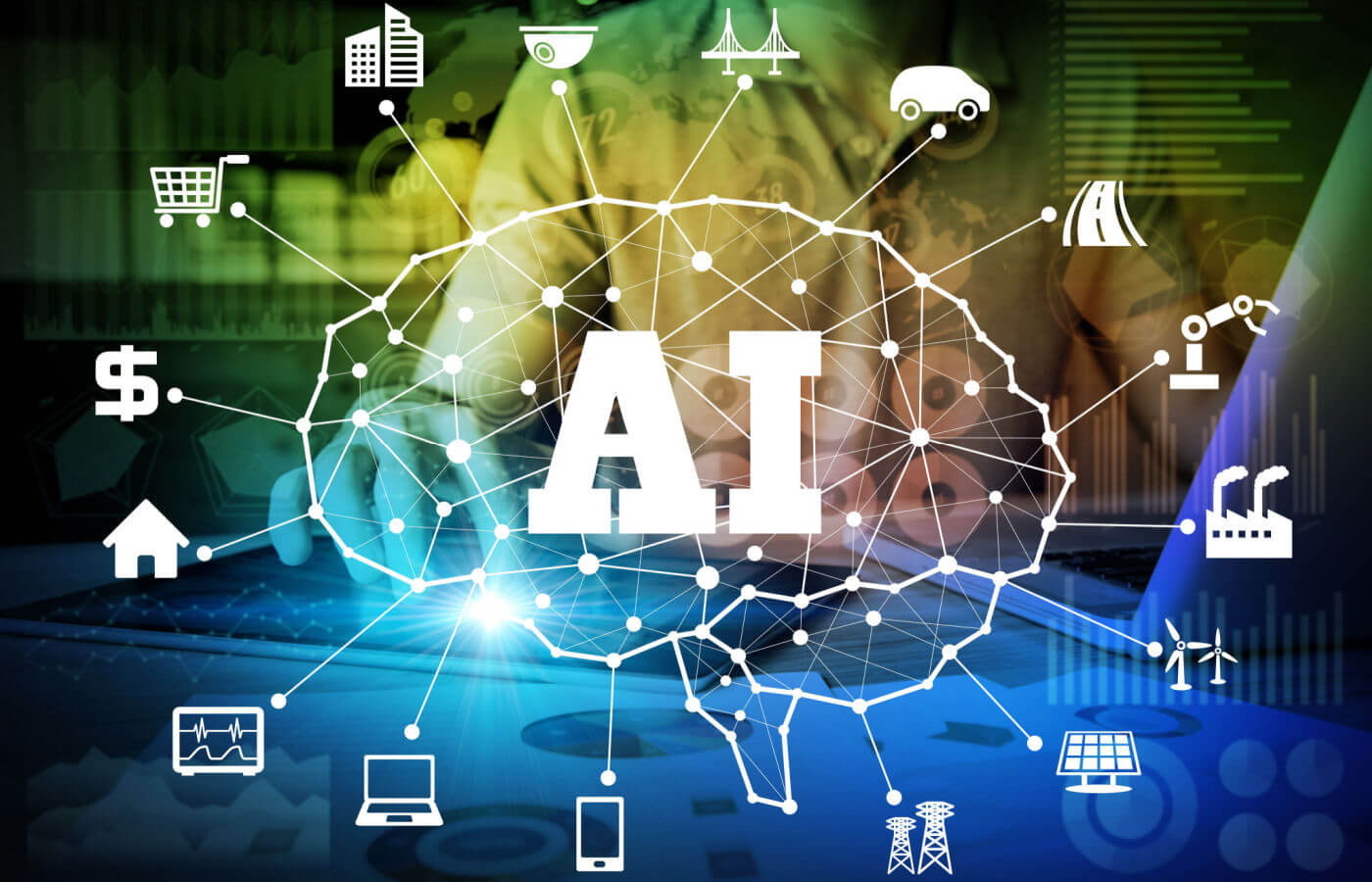
Understanding OpenAI’s Deep Research AI Technology
Overview of Generative Artificial Intelligence
Generative artificial intelligence (AI) has advanced over the years, introducing AI agents capable of browsing the web to find answers to various queries. This technology shows promise, although it still requires significant improvement. OpenAI has recently showcased its Deep Research technology, which is specifically designed to utilize the vast resources available online effectively.
Deep Research Performance
In a recent study, OpenAI’s researchers indicated that Deep Research outperformed other models developed by the company, particularly when it comes to answering questions based on web content. Additionally, it showed better performance than human researchers on complex tasks that typically require extensive searching. However, the Deep Research model still struggles, providing incorrect answers nearly half the time.
The researchers introduced a benchmark called "BrowseComp" to assess the capabilities of AI agents in navigating the web. This benchmark is intended to evaluate how well these models handle challenging questions that require in-depth research.
The Capabilities of AI Agents
AI agents like Deep Research are designed to sift through hundreds or thousands of web pages, offering potential advantages over humans. Human researchers often face limitations such as the inability to concentrate on multiple queries simultaneously and getting fatigued after long periods of searching. In contrast, AI can recall vast amounts of information without distraction.
Benefits of AI in Research
- Increased Efficiency: AI can process information at a speed and volume far greater than humans.
- Continuous Operation: Unlike humans, AI does not tire and can work round the clock to find solutions.
- Enhanced Data Processing: AI can manage multiple pieces of information at once, something humans find challenging.
The BrowseComp Benchmark
The BrowseComp evaluation includes a challenging set of 1,266 questions that test an AI’s ability to find hard-to-locate information online. These questions often involve complex relationships and deep connections between various topics. For example, one question asked about a research publication relating to cultural traditions, scientific processes, and culinary innovations, which demanded extensive searching and understanding.
Human Performance Compared to AI
In an early phase of the study, human participants familiar with the dataset attempted to answer these difficult questions. The results were disappointing; humans managed to provide answers to only 30% of the questions, and most gave up after two hours. Furthermore, 14% of the suggested answers differed from the actual correct answers. The researchers speculate that individuals with specialized search skills, such as investigative journalists or detectives, might perform better under similar conditions.
Challenges Encountered by AI
When tested against various OpenAI models like GPT-4 with and without browsing capabilities, Deep Research still faced substantial challenges. Both GPT-4o and GPT-4.5 achieved near-zero accuracy during the tests, which underscores the complexity of the problems being addressed. However, Deep Research managed to attain a success rate of 51.5%, showing effectiveness in answering niche questions requiring extensive web navigation.
Despite this success, an issue known as "calibration error" emerged, meaning that AI systems like Deep Research sometimes displayed overconfidence in their incorrect answers.
Methods to Improve Performance
To mitigate the calibration error, researchers tested a version of Deep Research that generated multiple potential answers for each question, selecting the best among them. This approach helped improve its accuracy and demonstrated that the AI could often determine the correct answer even when it struggled to express that confidence as probability.
The research revealed that the performance of Deep Research improved with more computational resources, emphasizing the correlation between processing power and question accuracy. Overall, the findings suggest that when AI models are prompted to evaluate their own responses, their accuracy tends to increase.
Limitations of the Study
While BrowseComp provides useful insights into the performance of AI agents, the researchers acknowledge its limitations. The benchmark focuses primarily on questions that AI can easily interpret and verify. Consequently, it does not encompass more complex questions requiring nuanced responses or the ability to handle ambiguous user inquiries.