“OpenAI’s o3 Achieves a Score of 136 on the Mensa Norway Test, Outperforming 98% of Humans.”
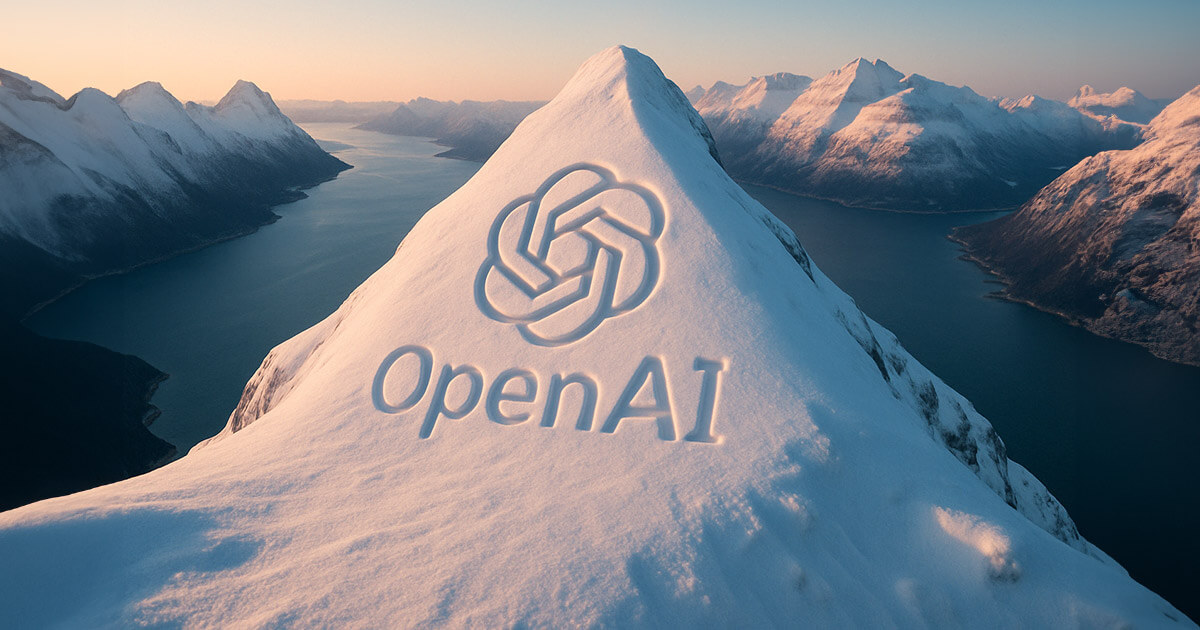
OpenAI’s o3 Model Achieves High IQ Score
OpenAI has recently introduced its latest language model, named the "o3," which has made headlines by scoring an impressive 136 IQ on a public Mensa Norway intelligence test. This score not only surpasses the entry threshold for Mensa but also positions the o3 model above approximately 98% of humans based on standard IQ distribution.
Testing Background
The IQ score of the o3 was derived from a rolling average across seven attempts during the assessment, using a standardized bell curve. This method of benchmarking highlights the strengths of the o3 model compared to both proprietary and open-source alternatives in cognitive evaluations. The score was published through independent platform TrackingAI.org, emphasizing the trend that closed-source models often outperform their open-source counterparts in similar tests.
O-Series Benchmarking and Results
The "o3" model is part of OpenAI’s O-series of large language models, which have excelled in various testing formats. The evaluation for o3 included two primary tests: the proprietary Offline Test from TrackingAI and the public Mensa Norway test. On the Offline Test, o3 achieved a score of 116, but it showed a significant improvement on the Mensa test, boosting its score by 20 points. This discrepancy may point to a better alignment with the structure of the Mensa test or data-related factors like familiarity with the prompts used.
Structure of Assessments
TrackingAI.org follows a systematic approach to its assessments. Each model receives a set of statements paired with four response options: Strongly Disagree, Disagree, Agree, and Strongly Agree. The model must select one and justify its choice using a brief response, typically structured for clarity. If a model refuses to answer, the prompt may be repeated multiple times to encourage a response, and the last successful attempt is recorded for scoring.
Performance Comparisons
The results from the Mensa test have clarified the performance landscape among various AI models. The o3’s score of 136 places it distinctly ahead of other top contenders, such as the GPT-4o, which scored 95 on the Mensa test and notably lower on the Offline Test with a score of 64. Among open-source models, Meta’s Llama 4 Maverick scored 106 on Mensa and 97 on the Offline benchmark, which highlights the performance gap between corporate-backed models and those developed through community efforts.
Limitations of Multimodal Models
It’s important to note that models designed to handle both text and images generally performed poorer on these tests compared to text-only versions. For example, OpenAI’s o1 Pro scored 107 in its text version but only 97 when adjusted for vision capabilities. The Mensa test reflected this trend even more starkly, where the text variant achieved 122, while the visual version fell to 86. This suggests that certain multimodal training methods may inadvertently hinder reasoning capabilities.
Insights Into Model Performance
While high IQ scores provide a glimpse into a model’s cognitive abilities, they do not completely reflect the models’ multifaceted capabilities, such as planning, factual accuracy, or engaging in multi-turn reasoning. Furthermore, the testing conditions—like instant access to prompts and high processing speeds—blur the lines between AI performance and human cognition.
Researchers at TrackingAI.org have acknowledged the challenges in avoiding exposure to training sets entirely, highlighting the intricacies involved in interpreting the results.
The Role of Independent Evaluators
With the rise of models like OpenAI’s o3, the need for third-party evaluations is becoming increasingly important. Organizations like LM-Eval, GPTZero, and MLCommons are stepping in to provide additional assessments, filling the gap left by the limited disclosures from model developers. This trend is shaping the standards for AI testing, making it important to understand that while IQ scores are intriguing, they represent a narrow view of a model’s overall intelligence capabilities.
As we see more rapid developments in AI and language modeling, continued scrutiny through independent evaluations will be essential for clarifying the validity and reliability of current benchmarks.