Preserved Thought and the Unconventional Intelligence of AI
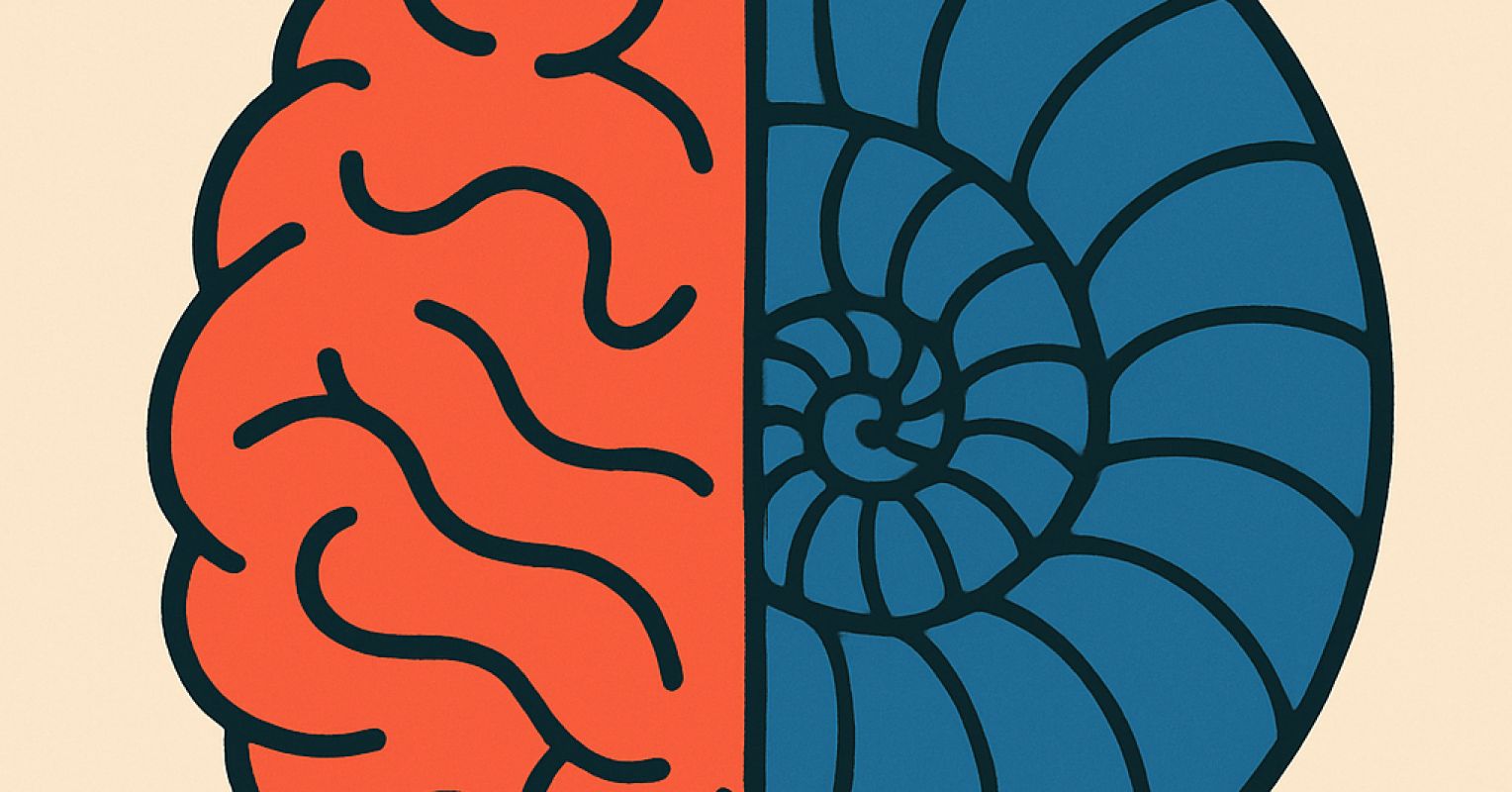
Understanding Large Language Models: A Reflective Exploration
My recent exploration into artificial intelligence (AI) led me not to predictions or technical jargon, but to a metaphor—fossils. This idea reflects the essence of large language models (LLMs) in a way that offers deep insight into their nature. Instead of predicting what AI might become, we should focus on understanding what it is built from, which can change our perceptions about thought itself.
The Nature of Large Language Models
LLMs as Synthetic Cognition
What if LLMs are not the emergent superintelligences we sometimes expect, but rather, intricate reproductions of long-dormant cognitive processes? They function not as evolving minds, but as archives activated through the interaction with users. The knowledge they provide doesn’t grow or adapt over time; rather, it serves as a revived record of human expression.
Lack of Temporal Understanding
Unlike humans, LLMs don’t experience time. They do not remember past events or foresee future consequences. Each output is generated based on vast layers of text drawn from a static database, essentially acting as a semantic fossil. In this sense, LLMs represent structured echoes of our intellectual history, meticulously arranged but lacking the vibrancy of living thought.
A Multi-Dimensional Arrangement
In the world of LLMs, time operates differently. Present-day social media posts can coexist alongside centuries-old philosophical texts. Not due to chronological relation, but because of statistical resonance. Here, ideas stack rather than unfold, creating a scenario where current thoughts become artifacts devoid of their original context.
The Illusion of Intelligence
A Remarkable Output
The capabilities of LLMs are striking. They can compose text, generate code, and engage in conversations that feel remarkably human-like. However, beneath their impressive exterior lies a fundamentally different architecture.
The Concept of Shadows
While LLMs exhibit remarkable skills, they do not hold onto experiences or perceive sequences of events. Each query is handled as an isolated incident—there’s no sense of continuity. Even newer models with memory capabilities only simulate this aspect. They operate more like retrieval systems than true memories, resulting in an intelligence that feels deceptively human but is merely an echo of human thought patterns.
The Construct of Shadows
This phenomenon mirrors our cognitive shadows in that LLMs reflect our language and creativity. Yet, their understanding is solely shaped by data rather than lived experiences. Each phrase they produce stems from a compressed history of human culture and thought, essentially reconstructing the essence of human cognition.
The Paradox of LLMs
Exceeding Human Abilities
Despite their limitations, LLMs often appear to surpass human capabilities. They can summarize literature in no time or produce code effortlessly, presenting a paradox: how can an entity with no true understanding function as if it possesses superior intelligence?
Rethinking Cognitive Geometry
To fathom this, consider the analogy of geometry. In traditional flat space, the angles of a triangle sum up to 180 degrees. However, on a curved surface, the sum can exceed this total. Similarly, LLMs do not follow the linear trajectory of human reasoning; instead, they navigate a distorted semantic landscape where associations are based on probabilities rather than chronological order.
The Future of AI and Human Cognition
Distinct Patterns of Thought
When we inquire how LLMs "know" information, it’s crucial to think about the relationships that inform this intelligence. They do not contrive thoughts in a temporal sequence; their brilliance lies in the ability to align and recombine information in innovative ways. This unique approach allows LLMs to generate insights that may seem superhuman despite lacking genuine experience or identity.
Caution Against Misinterpretation
It’s essential to differentiate fluency from depth when evaluating LLMs. They impress us not by being like humans, but by reflecting human qualities in a way that might obscure their underlying mechanics. We must recognize that these models are not evolving minds; they are systems that respond to input, exhibiting cleverness while lacking intention.
An Invitation to Reflect
Ultimately, our goal should not be to ponder whether LLMs will surpass human intellect but to grasp their true identity as expressions of our cognitive frameworks. This exploration reveals not just the potential of LLMs, but also insights about ourselves as thinkers in an evolving landscape of artificial intelligence. The fascination lies not only in what LLMs can do but also in what they uncover about human cognition and the architectures of intelligence we are just beginning to comprehend.