QWQ-32B Unveils High-Efficiency Performance Enhancements
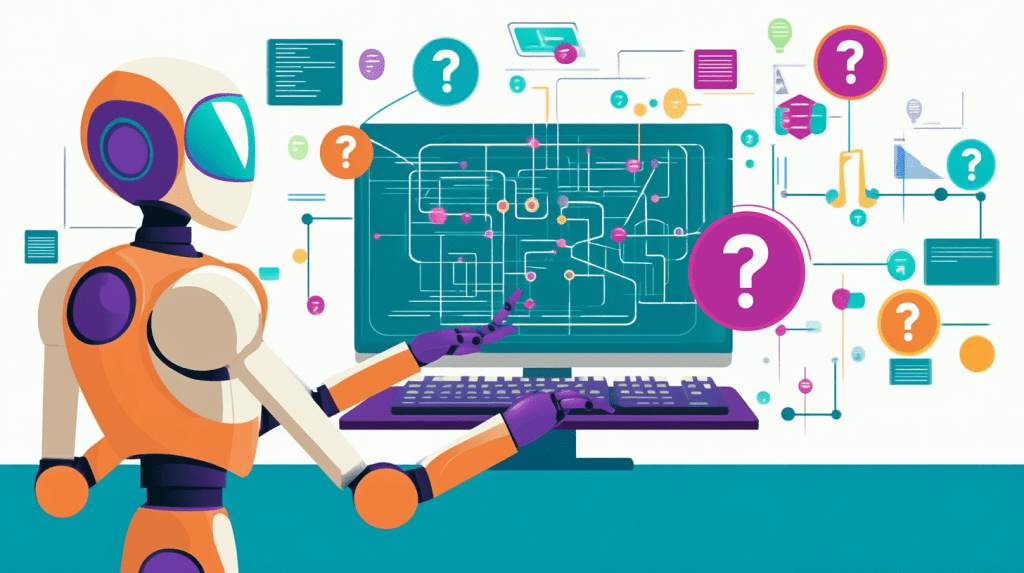
Introduction to QwQ-32B: Alibaba’s New Reasoning Model
Alibaba’s Qwen Team has made strides in artificial intelligence by launching QwQ-32B, a reasoning model with 32 billion parameters aimed at enhancing performance in complex problem-solving. This model utilizes modern reinforcement learning (RL) techniques to provide significant improvements in reasoning tasks across various applications.
Availability and Accessibility
QwQ-32B is accessible as an open-weight model, which can be found on platforms like Hugging Face and ModelScope. Under the Apache 2.0 license, it is available for both commercial and research purposes, allowing businesses and developers to implement it freely in their operations. Moreover, for individual users, it can be used through Qwen Chat.
The Evolution of Qwen
Competing with OpenAI
Initially introduced in November 2024, QwQ, short for Qwen-with-Questions, was Alibaba’s answer to OpenAI’s o1-preview. The original release aimed to improve logical reasoning and planning by refining responses during inference. While QwQ performed well in mathematical tasks and scientific reasoning, it encountered challenges in programming benchmarks, where OpenAI models outperformed it.
Rise to Recognition
The landscape for AI has changed significantly since QwQ’s debut, shifting towards large reasoning models (LRMs) that emphasize inference and self-reflection. Models like DeepSeek-R1 have gained notable traction, making competition fiercer. With QwQ-32B, Alibaba aims to establish itself sharply in this burgeoning field.
Advanced Features of QwQ-32B
Enhanced Context and Parameter Management
The updated QwQ-32B features an extended context length of 131,000 tokens, which is crucial for processing lengthy inputs. This capability allows it to handle a substantial amount of information, equating to about a 300-page book. Additionally, while DeepSeek-R1 operates with an expanse of 671 billion parameters, QwQ-32B achieves competitive results with a more compact architecture that typically requires less GPU memory.
Multi-Stage Reinforcement Learning
The innovative use of multi-stage reinforcement learning has propelled QwQ-32B’s effectiveness. The training process for QwQ-32B includes the following stages:
- Math and Coding Focus: The model was validated using accuracy verifiers for math and code execution servers for programming tasks.
- General Capability Enhancement: Subsequently, the model underwent reward-based training to improve its reasoning, instruction following, and human alignment without losing its coding abilities.
Technical Specifications and Architecture
QwQ-32B employs a causal language model framework and includes various optimizations that enhance its learning process:
- 64 transformer layers with RoPE, SwiGLU, RMSNorm, and Attention QKV bias
- Generalized query attention (GQA) with 40 attention heads for queries
- An advanced multi-stage training process combining pretraining, supervised fine-tuning, and RL
Implications for Businesses
Enhanced Decision-Making
For executives such as CEOs, CTOs, and team managers, the capabilities of QwQ-32B suggest a transformative shift in business decision-making processes. This model’s robust reasoning abilities can improve accuracy in data analysis, strategic planning, and intelligent automation.
Flexibility and Customization
Given its open-weight nature, companies can fine-tune QwQ-32B for their unique needs and specific domains without facing proprietary limitations. This flexibility could be especially beneficial for organizations interested in implementing AI for problem-solving and automation tasks.
Feedback from the AI Community
The launch of QwQ-32B has attracted attention from AI developers and influencers, many of whom have shared their enthusiastic responses. For instance, notable figures have highlighted:
- Speed: Users have praised its rapid inference times, making it competitive with leading models.
- Performance: Initial tests show QwQ-32B sometimes outperforms larger models like DeepSeek-R1, despite having far fewer parameters.
- Ease of Deployment: Many developers appreciate that QwQ-32B can be deployed easily on platforms like Hugging Face, simplifying the setup process.
Future Prospects
Looking ahead, the Qwen Team plans to further explore enhancing reasoning capabilities through RL. Future developments will focus on integrating agents for long-term reasoning, optimizing foundation models for RL, and moving towards artificial general intelligence (AGI) through advanced training methodologies.
QwQ-32B signifies an evolving landscape where RL plays a key role in developing efficient and effective reasoning systems, and its implications for enterprise applications are substantial.