Recent AI Models Utilize Only a Few Nvidia Chips for Processing
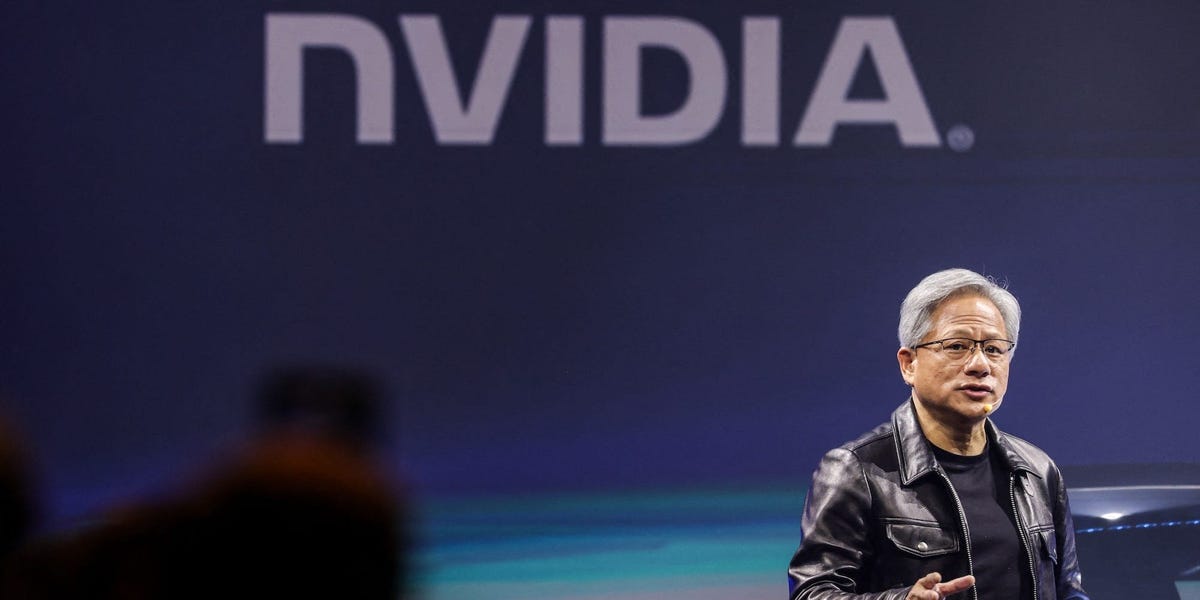
Emerging AI Models: Using Fewer Chips for Greater Efficiency
Introduction to Efficient AI Models
Recent developments in artificial intelligence highlight a trend towards creating models that deliver impressive performance while requiring significantly fewer computing resources, particularly Nvidia’s GPUs. Companies like Google and Cohere are at the forefront of this movement, unveiling advanced AI systems that challenge traditional notions of computational demands.
Google’s Gemma 3: A New Benchmark
Google’s latest offering, the Gemma 3, stands out in the AI landscape for its efficiency. During a recent announcement, CEO Sundar Pichai emphasized that this model can achieve substantial results using just one Nvidia GPU. In fact, he stated that achieving similar performance with competing models would require at least ten times the computing power. This remarkable efficiency showcases a growing trend among tech companies to optimize performance without disproportionately increasing hardware costs.
Cohere’s Command A: Minimal Hardware Requirements
The Toronto-based startup Cohere has also made waves in the AI sector with its release of Command A, another innovative model that operates effectively on merely two GPUs. This development underscores the broader push within the industry to create powerful AI models while minimizing resource consumption.
The DeepSeek Effect: Lessons Learned
The launch of the R1 model by the Chinese startup DeepSeek in early 2025 was a pivotal moment in this efficiency movement. DeepSeek’s model demonstrated competitive abilities comparable to those of OpenAI’s top models, yet it required fewer chips. The market reacted dramatically, with Nvidia’s stock suffering an almost $600 billion loss in a single day as investors worried that reduced demand for Nvidia’s hardware could disrupt the company’s flourishing business, which previously hit a record revenue of $130.5 billion.
Performance Comparisons: A New Era of Metrics
Recent performance metrics show that models like Gemma 3 outperform not only DeepSeek’s offerings but also those from OpenAI and Meta, all while utilizing fewer GPUs. This newfound efficiency raises interesting questions about the sustainability of Nvidia’s market dominance.
Understanding the Jevons Paradox
One key concept to consider is the Jevons Paradox, an economic principle suggesting that as technology becomes more efficient, its overall consumption can increase. This phenomenon may explain why companies, including Google, are planning to ramp up their investments in AI infrastructure. Google intends to allocate $75 billion this year towards AI-related capital expenditures, primarily focusing on acquiring new GPUs.
Nvidia’s Continued Relevance
Despite rising efficiency and potential challenges posed by new AI models, Nvidia remains a significant player in the industry. Recent trends indicate that the market has yet to express deep concerns about the impact of these more efficient technologies, as Nvidia’s stock has seen an upswing of around 6% since the latest announcements. This suggests that investors still regard Nvidia as a reliable option in the evolving tech landscape.
The Impact of Proprietary Chips
Interesting dynamics arise when examining how models like Google’s Gemma are trained. Although it is designed to operate on a single Nvidia GPU, the training phase utilized Google’s own chips, known as Tensor Processing Units (TPUs). This raises important questions about Nvidia’s long-term dominance as tech companies invest in developing their own chips to diminish reliance on Nvidia’s GPUs.
Balancing Efficiency and Power
Simultaneously, Google is also pursuing the development of larger, more complex models, such as the Gemini lineup. These models aim to harness extensive computing power to push the boundaries of AI capabilities. The contrasting trends of developing efficient models versus larger-scale models requiring more resources will characterize the future of AI technology.
Conclusion
In summation, the evolution of AI models that utilize fewer chips presents both challenges and opportunities within the industry. As organizations strive to balance efficiency with power, the landscape of AI continues to transform, making it a compelling space to watch in the coming years.