Reviving a Vintage AI Method in Light of DeepSeek
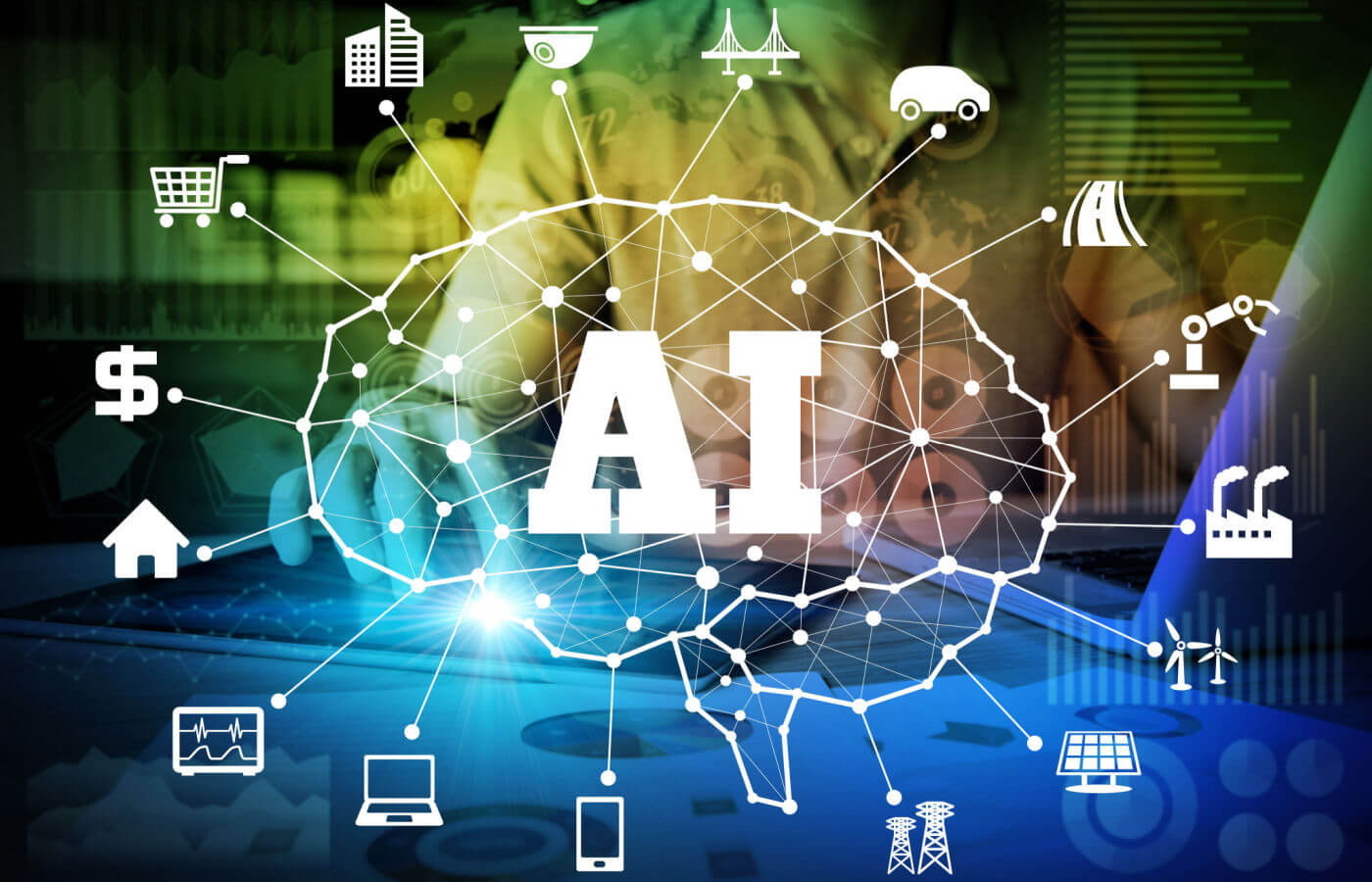
Reviving Traditional AI Techniques: The Impact of DeepSeek
Artificial Intelligence (AI) has undergone substantial evolution over the years, with various methods gaining and losing traction. Recently, traditional AI approaches are experiencing a revival, largely due to advancements like DeepSeek. This article explores what these old techniques entail, how DeepSeek is breathing new life into them, and the implications for the future of AI.
Understanding Traditional AI Methods
What Are Traditional AI Techniques?
Traditional AI refers to older methods that predate the widespread use of machine learning and deep learning. Some of the primary focus areas include:
- Rule-Based Systems: Logic-based systems utilizing predefined rules and heuristics to make decisions.
- Expert Systems: Programs designed to solve specific problems by mimicking the decision-making ability of a human expert.
- Symbolic AI: An approach that uses high-level symbolic representations of problems and logic to derive conclusions.
Even though these methods have been overshadowed by newer techniques, they still play a significant role in specific contexts.
Reasons for Their Decline
Several factors contributed to the decline of traditional AI methods:
- Complexity of Problems: As the complexity of data increased, traditional techniques struggled to keep pace.
- Computing Power: The rise of powerful GPUs has made data-driven methods more feasible and attractive.
- Data Availability: The proliferation of big data favored statistical methods over rule-based systems.
The Role of DeepSeek
What is DeepSeek?
DeepSeek is an innovative AI framework developed to enhance knowledge discovery in large datasets. By combining traditional AI principles with advanced machine learning techniques, it aims to provide a comprehensive solution for data analysis.
How DeepSeek Revives Traditional AI
DeepSeek reintroduces older AI methodologies in several key ways:
- Hybrid Models: It employs both traditional rule-based logic and modern machine learning techniques to analyze data, providing a more robust framework.
- Knowledge Representation: DeepSeek focuses on constructing knowledge graphs that leverage symbolic reasoning, ensuring valuable insights are derived from the data.
- Interpretability: By integrating symbolic AI, DeepSeek offers greater transparency in its decision-making processes compared to purely data-driven approaches, making results easier to understand.
Benefits of Renewed Interest in Traditional AI
Enhanced Decision-Making
- Combining Strengths: The revival of traditional techniques allows for enhanced decision-making by leveraging the precision of rule-based systems alongside the adaptability of machine learning.
- Improved Efficiency: Hybrid systems can streamline processes, making them both faster and more efficient.
Increased Transparency
- Understanding the ‘Why’: Utilizing traditional AI techniques fosters a clearer understanding of how AI systems arrive at decisions, addressing concerns over "black box" models.
Challenges Ahead
Integration Issues
- Data Compatibility: Combining traditional techniques with modern AI can pose challenges, particularly in ensuring data compatibility.
- Skill Gap: There may be a shortage of expertise in traditional AI methods, which could hinder successful integration into current AI frameworks.
Keeping Up with Innovation
- Rapid Developments: The pace of advancement in AI can make it challenging to implement older techniques effectively within new models.
Future Implications
Reviving traditional AI methodologies like those seen in DeepSeek may be a turning point for the AI field. By harnessing the strengths of both traditional and modern approaches, researchers and practitioners could develop more effective, interpretable, and efficient AI systems. As we look ahead, the potential for these renewed methods to enhance various sectors—such as healthcare, finance, and technology—remains significant as organizations seek reliable and actionable insights from their data.