Revolutionary AI Technology Outperforms Supercomputers in Weather Prediction
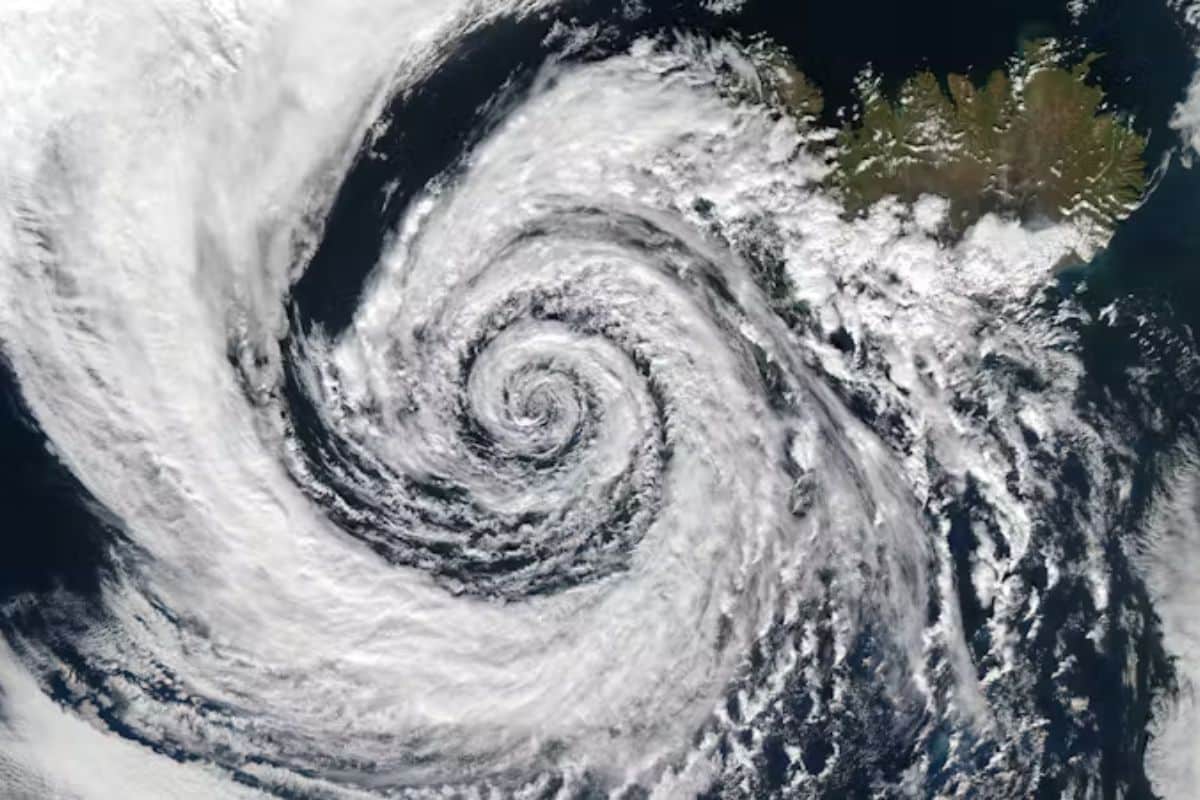
A new weather prediction system powered by artificial intelligence, called Aardvark Weather, is showing impressive capabilities, outpacing traditional supercomputers in terms of speed, efficiency, and accessibility. This advancement could make weather forecasting more accessible and environmentally friendly around the world.
Transforming Raw Data into Quick Forecasts
Aardvark Weather takes an innovative approach compared to standard forecasting methods that depend heavily on complex physical simulations. Instead of relying on extensive computational power and supercomputers running for hours, this cutting-edge system processes raw data and creates forecasts through machine learning techniques. This results in a more rapid, lightweight, and energy-saving forecasting process.
Efficient Operation on Standard Computers
Unlike traditional models like the Global Forecast System (GFS), which require substantial computing resources, the Aardvark system needs only a fraction of the data and can run effectively on a standard desktop computer.
Research from the University of Cambridge highlights that Aardvark operates using just 8% of the observational data utilized by conventional systems, yet it successfully matches or even surpasses their forecasting accuracy on a global scale.
Combining Speed and Sustainability
Aardvark’s advantages go beyond just performance. It is capable of producing forecasts tens of times faster than traditional systems and consumes thousands of times less energy, according to research published in Nature. By avoiding traditional atmospheric modeling, this innovative approach reduces the forecasting duration from hours down to mere minutes, which leads to a significant decrease in energy consumption.
“Weather forecasting systems have taken decades to develop, but in just 18 months, we have produced something that competes with the leading systems using merely a tenth of the data on a regular desktop,” stated Richard Turner, an engineer at the University of Cambridge.
Current Limitations
However, it’s important to note that the existing version of Aardvark has a lower spatial resolution compared to some of the best models. With its 1.5-degree prediction grid, Aardvark covers larger areas than the 0.25-degree grid used by GFS, which may impact the precision of localized forecasts. Nonetheless, researchers are optimistic that integrating regional data can help enhance this model over time.
“What we have achieved so far is just the tip of the iceberg for Aardvark. This comprehensive learning method can also be implemented in other weather forecasting challenges, such as predicting hurricanes, wildfires, and tornadoes. The ramifications stretch beyond just weather; it can also be used for forecasting air quality, ocean behaviors, and sea ice patterns,” explained co-author Anna Allen from the University of Cambridge.
Global Accessibility in Weather Forecasting
Aardvark has the potential to transform weather forecasting in regions not served by supercomputers. Scott Hosking from The Alan Turing Institute emphasizes the ability to deliver accurate weather forecasts to underprivileged and data-scarce regions worldwide.
By using standard computers for real-time weather predictions, this methodology paves the way for advanced forecasting to reach a global audience. The implications are significant for various sectors, including agriculture, disaster management, and infrastructure development.
As global weather patterns become increasingly unpredictable and severe, tools like Aardvark represent a major breakthrough in providing not just speed but also widespread access.