Revolutionizing Zero-Shot Multimodal AI with Meta AI’s MILS
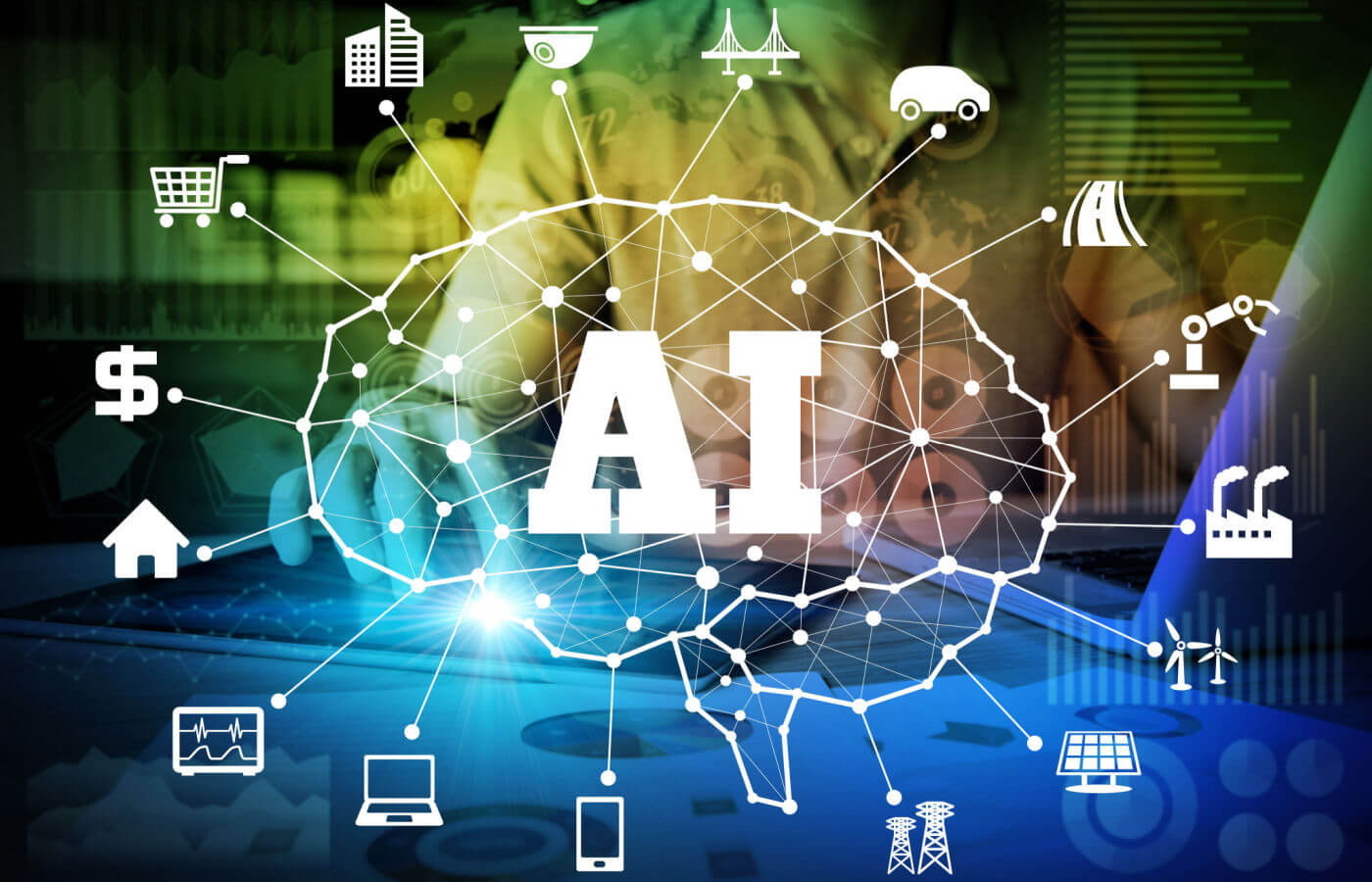
Understanding Multimodal AI: A New Frontier
Artificial Intelligence (AI) has seen remarkable advancements over the years. However, traditional AI systems have been limited to processing a single type of data—making them less adaptable and less capable of understanding complex contexts. Most AI models are unimodal, working exclusively with one format such as text, images, or audio. While these models can excel in specific tasks, they struggle to integrate or make connections across various types of data.
The Rise of Multimodal AI
In response to this challenge, multimodal AI has emerged, enabling models to process and integrate data from multiple sources to create a unified understanding. This allows for tasks such as:
– Converting images into text descriptions
– Generating captions for videos
– Synthesizing speech from text inputs
However, developing multimodal AI systems presents significant hurdles. They typically require vast amounts of labeled data, which can be both costly and difficult to gather. Additionally, these models often need customized fine-tuning for every new task they tackle, making them complex and resource-intensive.
Challenges in Traditional Multimodal AI
Many traditional multimodal AI systems face:
– **Complexity**: The integration of various data types demands more computational resources and longer training periods compared to unimodal systems.
– **Data Limitations**: The quality of data greatly affects performance, and inconsistent quality across different data formats can introduce errors.
– **Alignment Issues**: Accurately synchronizing data from diverse sources is intricate, as each data format has unique characteristics and processing requirements.
As multimodal AI relies on large, quality datasets, the lack of available labeled data can become a barrier to effectiveness. To address these limitations, advancements like Meta AI’s Multimodal Iterative LLM Solver (MILS) employ innovative strategies.
Zero-Shot Learning: A Game Changer
One significant advancement in AI is **zero-shot learning**. This approach allows models to handle tasks without needing specific prior training. Traditional machine learning models depend on large, labeled datasets for each type of task. However, zero-shot learning enables AI to adapt its existing knowledge to new situations, similar to how humans learn from experience.
For instance:
– A traditional AI model trained only on text would struggle to describe an image without prior visual training.
– In contrast, a zero-shot model like MILS can analyze and describe the image using its existing knowledge.
The adoption of zero-shot learning enhances AI’s adaptability, reduces its dependence on large datasets, and allows for a broader range of applications across fields where obtaining labeled data is challenging.
How MILS Operates
Meta AI’s MILS enhances the understanding of multimodal data by utilizing a two-step iterative process. This involves two main components:
– **The Generator**: A Large Language Model (LLM) that provides various interpretations of an input.
– **The Scorer**: A pre-trained multimodal model that evaluates these interpretations and ranks them by accuracy.
This feedback loop allows MILS to refine its outputs continuously, resulting in enhanced accuracy without the need for extensive retraining. This dynamic optimization makes MILS more flexible and less reliant on large labeled datasets.
Practical Applications of MILS
MILS can effectively handle several multimodal tasks, including:
– **Image Captioning**: By refining captions through the interplay of LLMs and multimodal models.
– **Video Analysis**: Generating coherent descriptions of video content.
– **Audio Processing**: Translating sounds into descriptive text.
– **Text-to-Image Generation**: Preparing prompts for improved image rendering in AI models.
– **Style Transfer**: Ensuring visually consistent transformations in editing.
By employing pre-trained models as scoring mechanisms, MILS achieves superior zero-shot performance, simplifying the integration of multimodal reasoning into various applications.
Advantages of MILS Over Traditional AI
MILS offers significant benefits compared to traditional AI models, particularly in terms of efficiency and cost. Conventional AI systems typically require distinct training for every data type, leading to high computational costs. In contrast, MILS dynamically refines outputs, greatly reducing resource demands and making advanced AI accessible to a broader range of organizations.
Furthermore, MILS has demonstrated higher accuracy in various benchmarks, particularly in video captioning tasks. Its iterative refinement process captures more contextually relevant results than traditional one-shot models that may falter with new data types.
Scalability and adaptability make MILS a flexible solution, capable of integrating into diverse AI systems without the need for retraining for new tasks or data types. As organizations increasingly seek to leverage AI, MILS stands out as an innovative response to the limitations of traditional approaches.