Search Signals and Data Employed by Google to Train Gemini AI Models
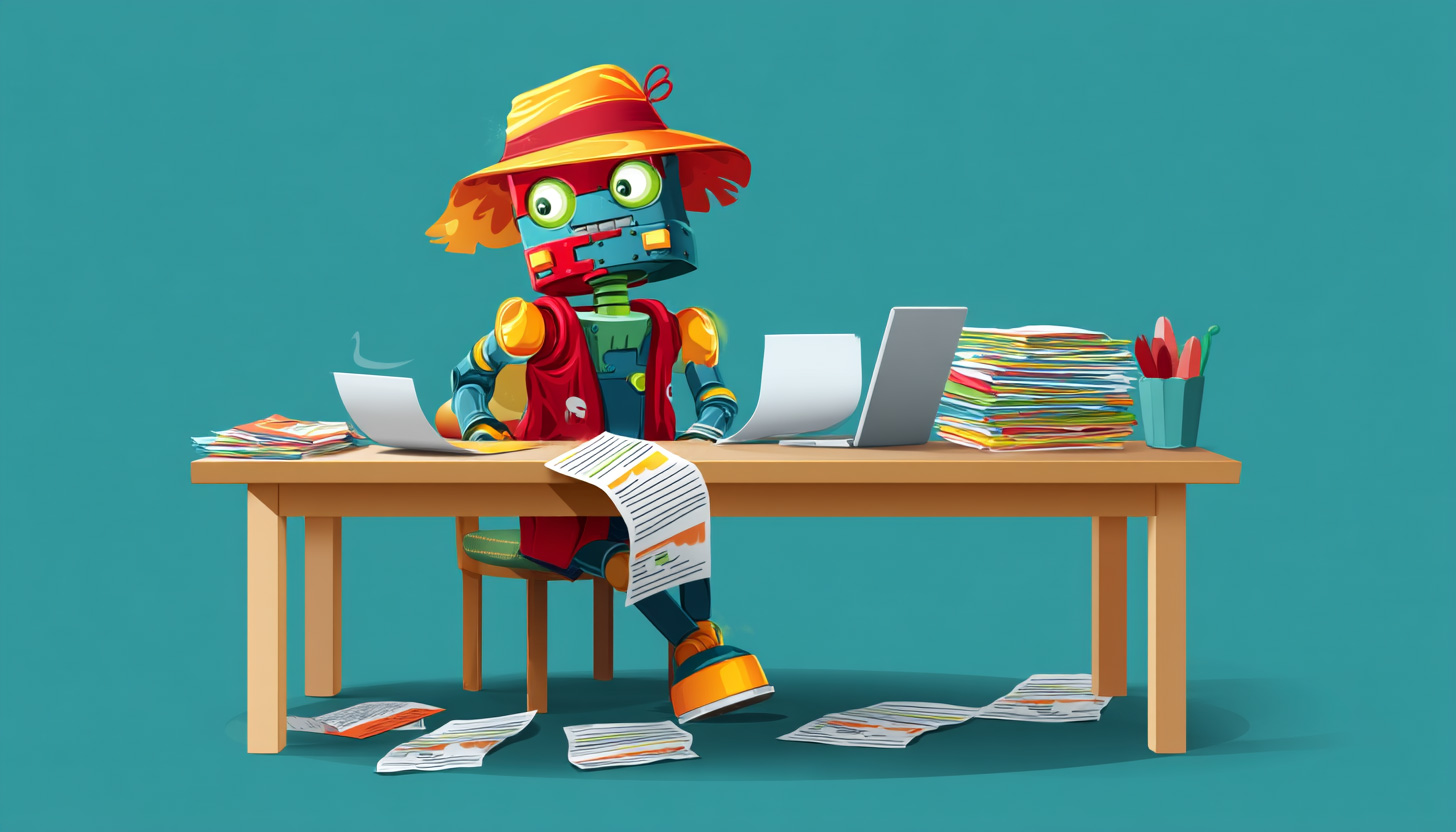
Google’s Use of Search Data to Train AI Models
Google has been leveraging its vast search engine data to enhance its artificial intelligence (AI) capabilities, particularly through its Gemini AI models. Recent testimony from a Department of Justice (DOJ) deposition sheds light on how Google’s search signals are utilized in this process, confirming what many users have suspected for some time.
How Search Data Improves AI Models
Insights from Internal Communications
In internal communications within Google, employees discussed how valuable search signals are for identifying credible sources. An example cited from an email highlights the intention to "upweight good authoritative pages" and "downweight the spammy untrustable ones." This approach aims to ensure that the information generated by AI is accurate, reliable, and of high quality.
The Role of User Feedback
Apart from search data, user feedback also plays a critical role in training AI models. According to testimony from Phiroze Parakh, a senior director of engineering at Google, search data was instrumental in pretraining models that contribute to features like AI Overviews in Google Search. Additionally, user interactions further refine how these models respond to specific queries, leading to improved results over time.
The Evolution of Google’s AI Models
Google’s AI initiatives have evolved significantly since their inception. The company has consistently stated its commitment to integrating search data into AI training. By harnessing information from its search engine, Google aims to enhance the performance and relevance of its AI offerings, including AI Mode and Gemini.
Key Implications for Search Results
The utilization of search signals for AI training carries several implications:
- Higher Quality Content: Authoritative and trustworthy sources are likely to be prioritized, improving the accuracy of search results provided by AI.
- Reduction of Spam: By downweighting poorly rated pages, Google can reduce the prevalence of spammy content in its search results, benefiting users seeking reliable information.
- Adaptive Learning: As user feedback is incorporated into training, Google’s AI systems continue to adapt and evolve, aiming for better relevance and user satisfaction.
Third-party Insights
Reports and observations from industry experts confirm that Microsoft’s competitor, Bing, is also integrating similar AI and search data strategies. As these tech giants push the boundaries of AI, the focus is not just on response accuracy but also on trustworthiness and user safety.
Ongoing Developments
Public discussions and analyses on platforms like Twitter further convey the community’s interest in how Google and other tech companies adapt their strategies around AI and data use. The recent revelations affirm that search data remains a cornerstone in Google’s quest to refine its AI capabilities.
As advancements continue, we can expect ongoing discussions on the ethical implications and effectiveness of using search data in AI training. Notably, the importance of trustworthy information will remain paramount as users navigate the evolving landscape of AI-assisted search technologies.
By leveraging search data effectively, Google is setting the stage for a new era of enhanced AI solutions that prioritize quality and user experience.