Seven Critical Mistakes That Derail Enterprise AI Initiatives
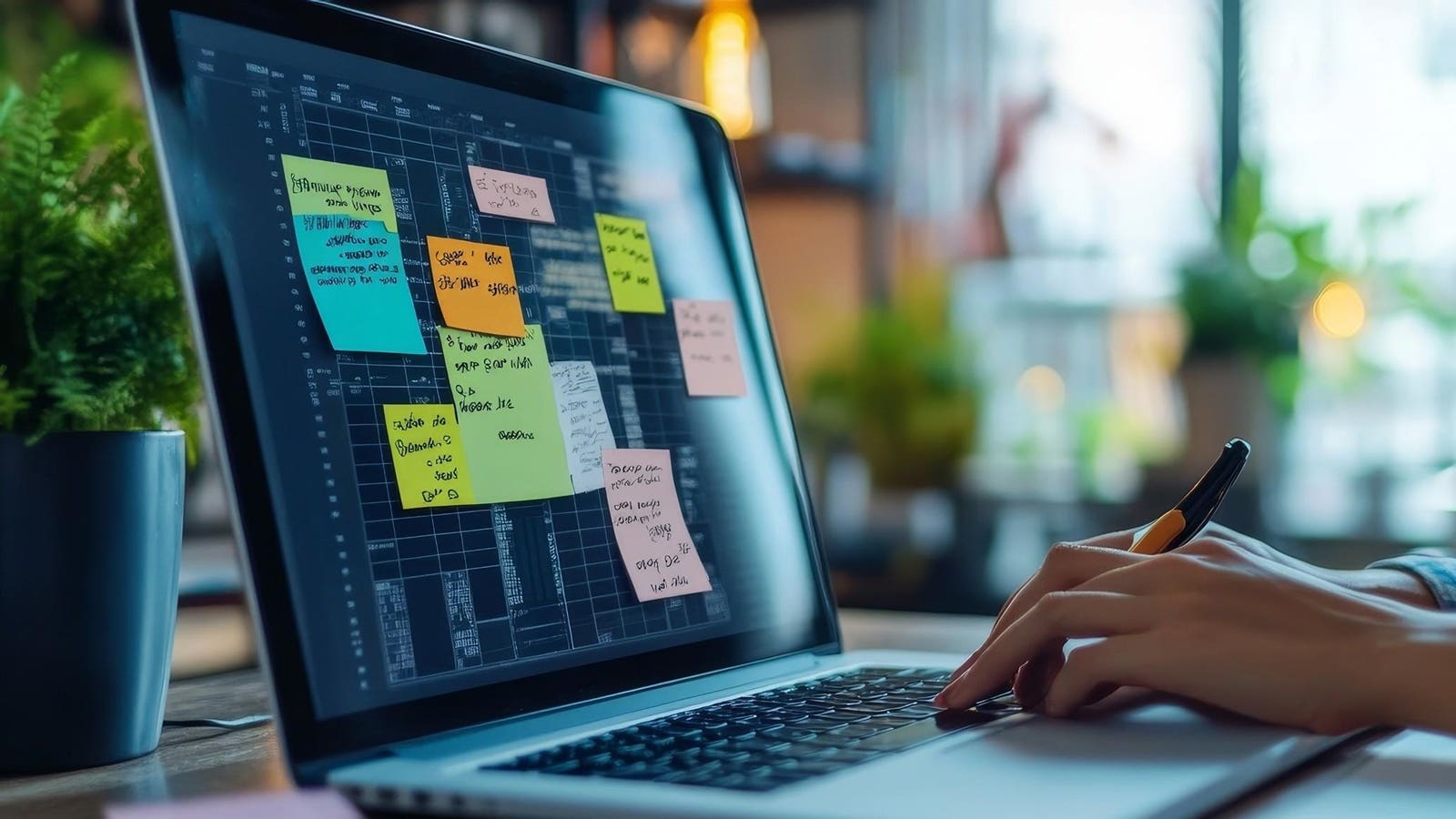
Millions of dollars are wasted every year on AI projects that fall short of their goals, leading to significant disappointment for businesses.
Within many organizations, AI projects are often struggling or failing. This could be a recommendation system meant to increase sales, a predictive maintenance tool designed to minimize downtime, or an advanced customer service chatbot expected to enhance response times. The stagnation of these projects signifies lost investments and unmet expectations, which can hinder future attempts at innovation.
The Discrepancy Between Expectations and Reality
AI projects resemble icebergs, with only a small portion visible above water—the successful results highlighted in vendor presentations or tech publications. Below the surface lies a considerable amount of work, including data preparation, technical infrastructure, skilled personnel, and necessary organizational adjustments, all critical for achieving those promising outcomes.
This gap between what is expected and the reality is a primary reason many AI projects fail. There’s a prevalent notion that AI is an instant solution to business problems, a kind of high-tech fix-all. However, the real process is complex and requires significant effort.
Take the case of a large consumer goods company I once advised. The leaders, motivated by impressive AI use cases, funded a $2.5 million project to enhance their supply chain. However, a year later, they were left with elaborate algorithms that couldn’t be implemented effectively because the inconsistent data across their numerous outdated systems had never been addressed. Essentially, they were trying to utilize a Formula 1 racing car on unpaved roads.
The Challenge of Data Quality
One of the most critical mistakes in AI initiatives is underestimating the need for high-quality data. Organizations often fail to comprehend both the volume and quality of data necessary for a successful AI deployment.
AI systems rely fundamentally on data processing. If low-quality data is fed into the system, the results will also be poor—a concept known as “garbage in, garbage out,” which has been accepted since the 1950s but still seems to catch many leaders off guard.
For example, a healthcare organization aimed to use machine learning to forecast patient readmissions. Halfway into the project, they discovered that the historical patient data they were using was riddled with biases. Instead of capturing genuine trends, the AI was learning these inaccuracies, much like trying to teach a person a language with a faulty dictionary.
Missing the Human Factor
Many organizations make the mistake of viewing AI implementation strictly as a technical task rather than considering the human elements involved. Successful AI adoption requires human collaboration and acceptance.
I remember a manufacturing company that invested $1.8 million in an AI system intended for production planning optimization. While the technology performed well in tests, the factory supervisors continued to rely on their conventional methods, disregarding the AI’s recommendations. The reason was simple: they hadn’t been included in the development process, nor had anyone addressed their concerns about how AI would impact their jobs.
The Strategy Misalignment
Many AI projects begin with a fundamental issue: they lack a clear link to real business challenges or strategic goals. Often, these initiatives emerge as solutions seeking problems rather than addressing genuine needs.
I’ve witnessed organizations kick off AI projects merely to keep up with competitors or because executives read about the technology in popular business articles. These projects ultimately fail because they lack a solid foundation in measurable business outcomes.
Talent Shortages and Governance Issues
The gap in AI talent remains significant. Data scientists with the right blend of technical skills and business insight are hard to find.
Moreover, many organizations do not establish effective governance structures for AI initiatives. Questions about ownership and decision-making remain unanswered, leading to confusion and ultimately project failure. For instance, a telecommunications provider I collaborated with had multiple departments developing separate AI solutions without coordination, resulting in wasted resources and project cancellations as initiatives competed for attention and funding.
Neglecting the Foundations
Successful enterprise AI requires laying a solid foundation first. Attempting to implement complex AI systems without first building a robust data infrastructure is like constructing a house without a proper base.
To successfully execute AI projects, organizations should master essential data management and analytics skills before moving on to machine learning and advanced AI methods. I worked with a retailer that wanted to implement AI-driven personalized pricing. However, they couldn’t even generate consistent weekly sales reports across their stores, leading to the project’s failure.