The Importance of AI Governance
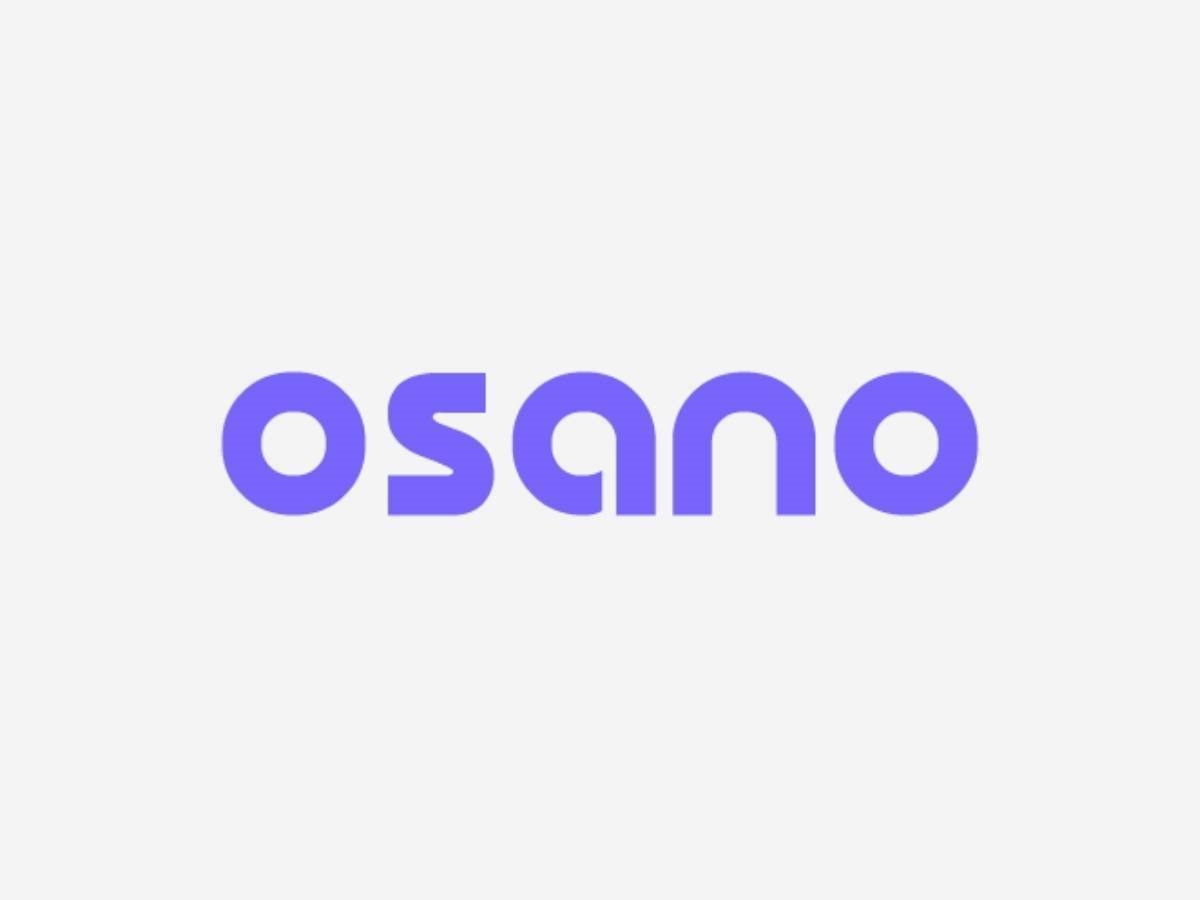
Understanding AI Governance
In March 2023, a significant open letter was signed by technology leaders, including Elon Musk and Steve Wozniak. The letter requested a halt on training advanced AI models until more robust governance regulations were established. Sam Altman, CEO of OpenAI, echoed the need for clearer AI regulations during his Congressional testimony two months later. Their efforts highlight a growing recognition of the importance of AI governance to prevent potential risks, including privacy violations and unethical practices.
What Is AI Governance?
AI governance refers to a structured framework of processes, principles, and policies to ensure that AI technologies are developed and used responsibly and ethically. With AI’s increasing presence in various sectors, from healthcare to finance, it’s critical for organizations to maintain transparency and accountability in their AI systems. This governance also addresses ethical standards and user privacy while minimizing risks, such as biases or errors that could adversely affect individuals.
The Importance of AI Governance
AI technologies are designed to automate tasks, analyze data, and predict outcomes, helping organizations save time and reduce errors. However, as reliance on AI systems grows, the need for effective governance becomes even more vital. It establishes guidelines to ensure AI applications are ethical, fulfill legal requirements, and respect user privacy.
Training AI Models and Data Dependency
AI learns to perform tasks through extensive training with data sets. This training enables the AI models to generate predictions based on patterns recognized during the learning process. Depending on the AI’s application, the volume of data needed can vary significantly. For instance, large language models (LLMs), like ChatGPT, typically require billions of data points, while smaller models may only need thousands.
AI learning relies on three essential elements:
- Volume: More data leads to a better understanding of subjects.
- Variety: Diverse data types help AI develop a nuanced understanding.
- Velocity: Rapid processing and analysis foster quicker decision-making.
Risks Associated with Data Use
While AI models continually improve, their quality is directly influenced by the data they are trained on. The potential for data privacy issues arises when proprietary or sensitive information is used without consent. Recognizing these risks is a cornerstone of effective AI governance.
Data Privacy Risks and Ethical Considerations
Critical Issues in Training Data
AI models often train on data gathered from various sources, including existing databases and the internet. This poses several privacy risks, especially if the data includes personal or identifiable information.
Informed Consent: Organizations must ensure they have informed consent from individuals before using their personal data. This includes clearly communicating the intended purpose of the data collection.
Scope of Consent: Users may agree to use their data for one purpose but not another. It’s imperative for organizations to respect these preferences while adhering to regulations such as GDPR and CCPA.
- Data Disclosure Risks: AI models might unintentionally disclose personal information in their responses if this data was included in the training set. Even with efforts to anonymize data, lapses can occur due to misunderstandings of privacy laws.
Addressing Bias and Discrimination
Biases can manifest in AI outputs through three main avenues:
- Training Data Bias: Occurs when the data set disproportionately represents certain groups, leading to skewed outcomes.
- Algorithmic Bias: Arises from errors in the programming where developers’ biases influence model behavior.
- Cognitive Bias: Involves biases introduced by developers during data selection based on their preferences.
For instance, historical medical research often underrepresents certain demographics, which can result in AI-driven health decisions disproportionately affecting those groups. These biases highlight the need for fairness in AI systems to prevent unintended discrimination.
Risks of Predictive Analytics
AI’s capacity to infer correlations between disparate pieces of data raises concerns, particularly regarding privacy. For instance, if an AI combines seemingly benign data points, it might incorrectly conclude sensitive information about a person, like their health status or lifestyle. Such inferences could have detrimental effects if misused.
Governance Frameworks and Standards
The formation of regulatory frameworks is critical as AI continues to evolve.
National and International Standards
The United States has started to establish AI governance principles through executive orders, focusing on safe and responsible AI development. Additionally, the European Union has enacted the EU AI Act, which delineates risk categories for AI systems based on their potential impact, from minimal to unacceptable risk. This act aims to safeguard consumer privacy while promoting transparency.
Other Notable Frameworks
- NIST AI Risk Management Framework: A voluntary guideline to help organizations integrate trustworthiness into their AI systems.
- ISO 42001: Offers a standard for managing AI systems effectively alongside existing frameworks.
- OECD Guidelines: Updated principles that encapsulate the need for accountable, safe, and transparent AI practices, urging collaboration among nations.
Levels of AI Governance
AI governance can be organized into several levels:
Global Governance
International guidelines help manage cross-border AI risks and encourage global cooperation in research and development.
National Governance
Country-specific regulations align AI practices with local priorities and legal requirements, fostering adherence to privacy and non-discrimination laws.
Industry-Specific Governance
Different sectors require tailored standards based on how AI is applied, ensuring safety and ethical decisions in high-risk areas like healthcare and finance.
Technical Governance
Technical standards regulate specific aspects of AI, such as algorithmic fairness and data protection protocols.
Organizational Governance
Companies need internal policies detailing how AI systems will be developed and applied to ensure ethical standards, compliance, and transparency. Educating employees about these practices and the significance of data privacy will empower organizations to navigate the complexities of AI responsibly.