The Importance of Open-Source for Fostering Competition in AI
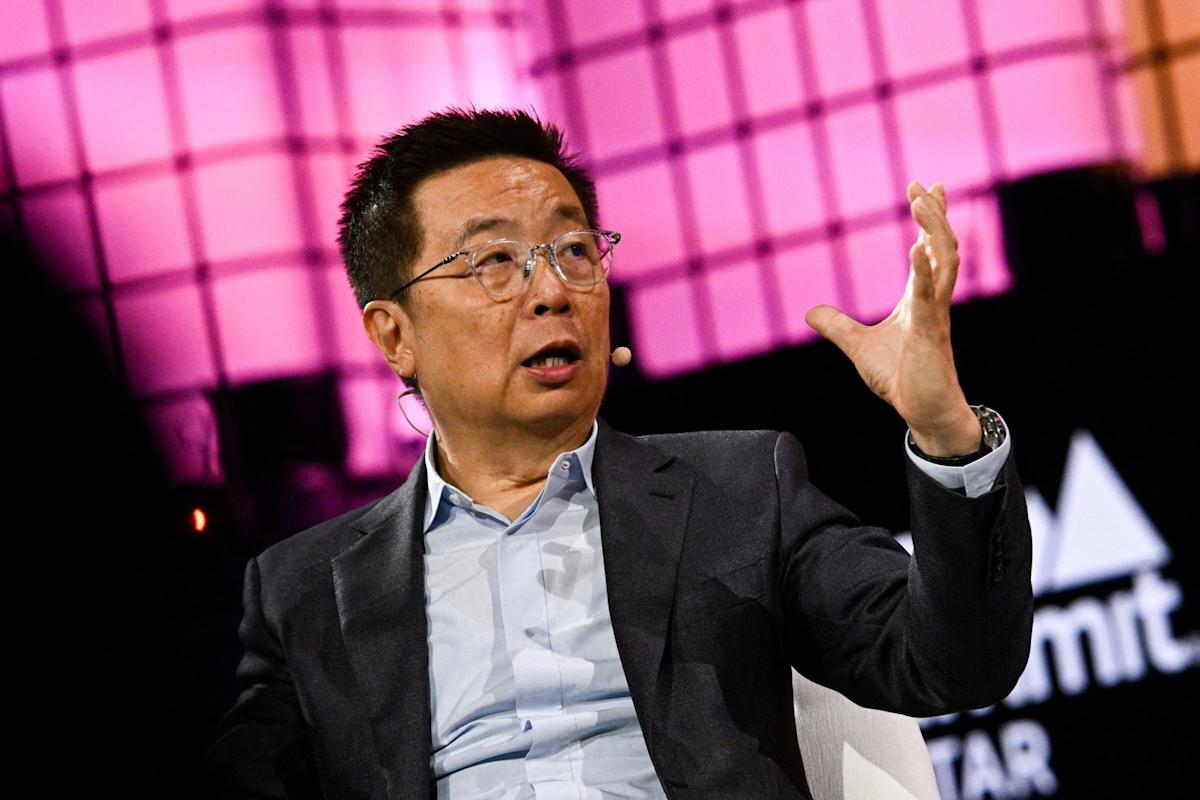
The Rise of Open-Source AI: A Changing Landscape
DeepSeek is reshaping the AI scene by bringing open-source frameworks back to the forefront. This Chinese startup’s innovative approach has prompted major companies to reconsider their own strategies. For instance, Baidu has now made its ERNIE model open-source, while OpenAI’s CEO, Sam Altman, has expressed concerns that his non-open-source philosophy places his company on the "wrong side of history."
As a result, the AI ecosystem is splitting into two distinct paths: closed systems supported by major corporations like OpenAI and Microsoft, and open-source platforms that are gaining traction thanks to companies like Meta and Mistral. This division isn’t just technical; it raises fundamental questions about the future of AI and who will control this potential trillion-dollar industry.
Historical Context: The Battle Between Open and Closed Systems
The evolution of software has always been characterized by a tug-of-war between open and closed systems. During the mainframe era, IBM’s closed systems dominated the market. The saying, “Nobody ever got fired for choosing IBM,” reflected businesses’ hesitance to stray from known giants. However, as technology evolved, companies began moving towards open systems that liberated them from vendor lock-in.
This pattern has repeated itself over time. For example, open-source Linux emerged as a strong competitor to Microsoft Windows, while databases like PostgreSQL and MySQL took center stage against Oracle. Vendor lock-in limits innovation, agility, and boosts vulnerability, especially as AI plays a more critical role in business processes. Open-source platforms help mitigate these risks by allowing organizations to switch providers or bring solutions in-house without incurring excessive costs.
The Significance of Open-Source AI
While consumers may appreciate the simplicity of closed platforms, businesses face different concerns. Organizations often deal with sensitive data and proprietary information that cannot be sent through opaque APIs. Here are some key advantages of open-source AI:
Data Security: Open-source models keep sensitive information within a company’s own infrastructure, reducing the risk of data breaches caused by external servers.
Customization: Enterprises can customize open-source models according to their specific needs, fine-tuning them with proprietary data without the limitations of closed systems.
- Cost Efficiency: Organizations can avoid high scaling fees charged by vendors by deploying open-source models on their own infrastructure.
Though closed platforms may seem more straightforward, they lack the safety, flexibility, and cost-effectiveness associated with open-source alternatives.
Interestingly, OpenAI initially succeeded due to open-source principles. The groundbreaking "Attention Is All You Need" paper from Google laid the groundwork for modern language models. Despite this backdrop, OpenAI has moved away from an open-source approach, raising doubts about its dedication to making AI beneficial for all.
Microsoft’s partnership with OpenAI has thrust the company into a leading position in the commercial AI sector, having invested over $13 billion to integrate GPT-4 across platforms such as Azure and Office applications. This creates a powerful lock-in effect for businesses reliant on these tools.
Historically, closed AI systems gained dominance through sheer scale in terms of data and computing power. However, an emerging trend—the reasoning revolution—suggests that advanced reasoning capabilities can compete with these massive proprietary systems, as demonstrated by models like DeepSeek’s R1.
Opportunities for Smaller Players
This shift provides significant opportunities for smaller companies and startups. Open-source AI encourages collaborative innovation, which is more cost-effective than closed systems, thereby democratizing access and facilitating contributions from diverse stakeholders.
Currently, the traditional AI landscape is largely ruled by a few major players, such as Nvidia in hardware and OpenAI in model development. Such dominance has created significant barriers to entry, primarily due to steep capital and computational requirements. However, innovations like optimized inference engines and specialized hardware are working to break down these barriers.
The AI stack is also becoming less centralized. Companies such as Groq are providing alternatives to Nvidia, while startups like Mistral are developing creative models that can compete with bigger firms like OpenAI and Anthropic. Platforms like Hugging Face are democratizing access to advanced models, and services like Fireworks and Together are enhancing request throughput and reducing latency. Alternative cloud marketplaces, such as Lambda Labs, are also offering competitive options against the leading firms.
Navigating the Open vs. Closed Debate
Nonetheless, open-source models come with their own set of risks. There are potential dangers, including misuse of training data, the creation of malicious applications like deepfakes, and ethical lapses in data utilization. To address these challenges, strategic governance measures may be needed. Delaying the release of sensitive models for security assessments and implementing partial weight-sharing could limit misuse while still allowing for research opportunities.
The future of AI depends on the ability to balance these competing interests, much like AI systems themselves balance weights for optimal performance. The choice between open and closed systems is more than a matter of preference; it represents a pivotal decision that will shape the future trajectory of AI development.