The Increasing Cost of Benchmarking Due to the Emergence of AI Reasoning Models
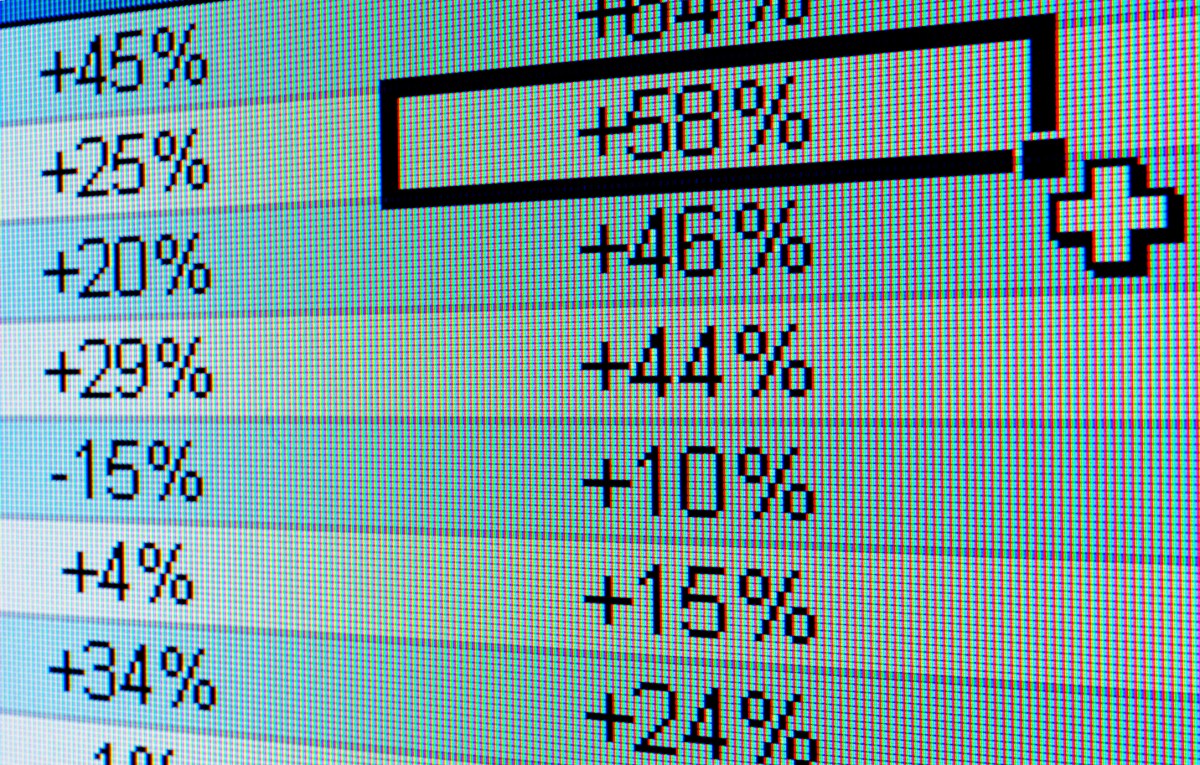
The Cost of Benchmarking AI Reasoning Models
AI labs, including OpenAI, assert that their reasoning models possess superior problem-solving abilities compared to earlier non-reasoning versions, particularly in fields like physics. While this assertion is generally supported by evidence, benchmarking these reasoning models presents significant financial challenges, complicating independent validation of their claims.
Cost Analysis of Benchmarking
According to Artificial Analysis, a third-party AI evaluation firm, assessing OpenAI’s o1 reasoning model costs approximately $2,767.05. This evaluation involves a suite of seven common benchmarks, including MMLU-Pro, GPQA Diamond, and Humans’ Last Exam. In comparison, testing Anthropic’s Claude 3.7 Sonnet — categorized as a “hybrid” reasoning model — costs around $1,485.35. Meanwhile, benchmarking OpenAI’s o3-mini model is notably less expensive at $344.59.
Interestingly, the cost of benchmarking can vary significantly among different reasoning models. For instance, Artificial Analysis spent just $141.22 on evaluating OpenAI’s o1-mini. However, when averaged out, reasoning models tend to incur higher costs overall. In total, Artificial Analysis has invested about $5,200 in evaluating around twelve reasoning models, compared to only $2,400 for over 80 non-reasoning models.
To illustrate, OpenAI’s non-reasoning GPT-4o model, released in May 2024, was much cheaper to evaluate at only $108.85. Similarly, it cost $81.41 to benchmark Claude 3.6 Sonnet, which is the earlier version of the Claude model.
Plans for Increased Benchmarking
George Cameron, co-founder of Artificial Analysis, remarked that as more AI labs introduce new reasoning models, the budget for benchmarking is set to increase. "At Artificial Analysis, we run hundreds of evaluations monthly and invest significantly in this," Cameron stated, hinting at plans to expand their benchmarking efforts as new models become available.
Escalating Costs for AI Testing
Artificial Analysis isn’t alone in facing rising benchmarking costs. Ross Taylor, CEO of the AI startup General Reasoning, reported spending $580 to evaluate Claude 3.7 Sonnet using 3,700 unique prompts. He estimates that running the MMLU Pro test alone would exceed $1,800. Taylor expressed concerns over the increasing gap between benchmarking expenses and academic resource availability, stating, “[N]o one is going to be able to reproduce the results.”
Reasons Behind High Benchmarking Costs
The primary reason for the high costs involved in testing reasoning models is their propensity to generate large volumes of tokens. Tokens can be as small as individual words, meaning that even a single evaluation can produce millions of tokens. For instance, OpenAI’s o1 model generated over 44 million tokens during the evaluation process, which is approximately eight times more than what the GPT-4o generated.
Most AI companies charge per token for usage, which significantly increases the overall cost of benchmarking. Moreover, modern evaluation benchmarks are designed to be more complex, often including multi-step tasks that require extensive token generation. Jean-Stanislas Denain, a senior researcher at Epoch AI, points out that today’s benchmarks, while having fewer questions, focus on evaluating real-world tasks — such as coding and internet browsing — thus requiring more tokens to accurately test model capabilities.
Increasing Costs Per Token in AI Models
Over time, the most sophisticated models have not only become more complex but have also increased in cost per token. For example, Claude 3 Opus was priced at $75 per million output tokens upon its release in May 2024, while OpenAI’s GPT-4.5 and o1-pro models cost $150 and $600 per million output tokens, respectively. Denain notes that while the cost for reaching certain performance levels has decreased, top-tier models remain expensive to evaluate.
While many AI labs offer free or subsidized model access for benchmarking organizations, experts have noted that this can compromise the integrity of the results. Taylor raised an important question about the validity of scientific findings in contexts where results cannot be replicated due to resource constraints.