The Rise of AGI with Manus: Innovations in AI Safety
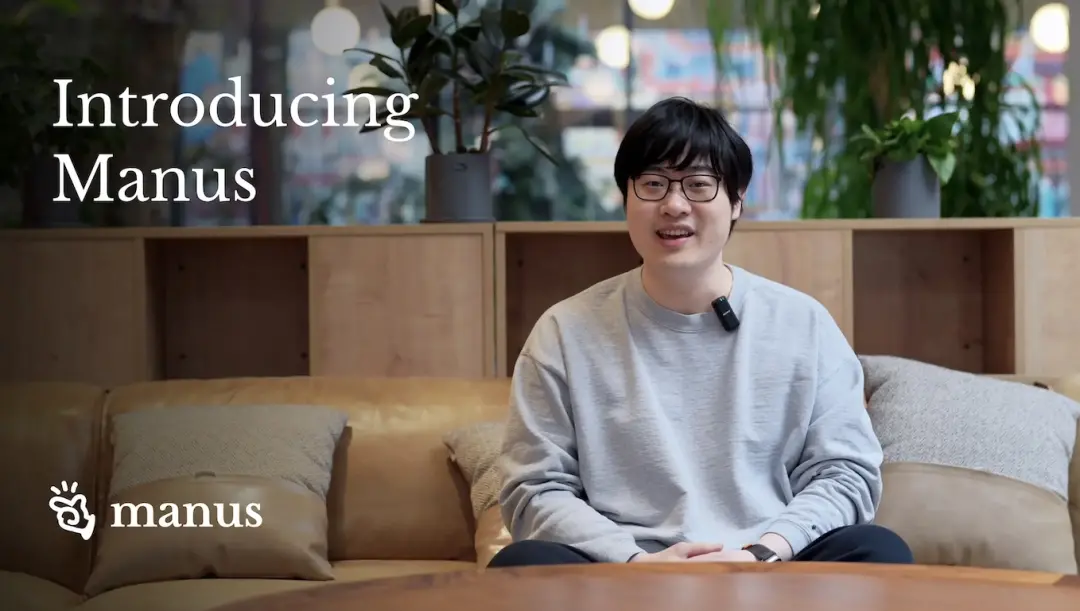
Manus: Redefining AI Performance
Achievements in Benchmarking
Manus has made headlines for achieving state-of-the-art results in the GAIA benchmark, outperforming models developed by Open AI at a similar level. This impressive performance allows Manus to tackle complex tasks independently. For example, it can handle multinational business negotiations, which involve breaking down contract clauses, making strategic forecasts, generating plans, and coordinating between legal and financial teams.
Compared to traditional systems, Manus possesses several key advantages. Its ability to dynamically decompose goals enables it to break down large tasks into hundreds of smaller, manageable subtasks. Additionally, Manus demonstrates impressive cross-modal reasoning ability, allowing it to handle and analyze diverse data types simultaneously. Its memory-enhanced learning capability means that Manus continually improves its decision-making efficiency while minimizing error rates through a process known as reinforcement learning.
The AI Development Debate: AGI vs. MAS
The rapid progress witnessed by Manus has sparked a significant debate within the tech community regarding the future directions of AI. A critical question arises: will we witness the dominance of Artificial General Intelligence (AGI), or move toward Multi-Agent Systems (MAS) collaboration?
Manus’s design philosophy hints at two distinct paths:
Path 1: AGI Focus
This path is about enhancing individual intelligence to reach comprehensive decision-making capabilities similar to those of humans.
Path 2: MAS Coordination
In this scenario, Manus acts as a super coordinator, orchestrating numerous specialized agents to collaborate effectively.
While superficially, these paths seem to focus on different areas of development, they highlight more profound contradictions in AI growth, such as the balance between efficiency and safety. As AI approaches the capabilities of AGI, risks associated with decision-making become more pronounced. Conversely, while MAS can help distribute risks, communication lags can prevent timely critical decisions.
Risks Associated with AI Development
The evolution of Manus has also made certain risks of AI technology more visible. Some of the most notable concerns include:
Data Privacy Issues: In healthcare, Manus’s need for real-time access to patient genomic data raises privacy concerns. During financial discussions, it could also deal with undisclosed corporate information.
Algorithmic Bias: Manus has displayed tendencies to provide lower salary offers to candidates from specific ethnic backgrounds during recruitment processes.
Misjudgment in Legal Tasks: When reviewing contracts, Manus has shown nearly a 50% misjudgment rate for clauses related to emerging industries.
- Vulnerability to Cyber Attacks: Clever attacks can mislead Manus during negotiations by utilizing specific audio frequencies to distort its assessment of an opponent’s bidding range.
As Manus and similar systems increase in intelligence, the potential for attacks escalates.
Security Measures in AI Systems
With the rise in concerns about security within AI, various strategies have emerged, especially in the realm of Web3 technology. The "impossible triangle" presented by Vitalik Buterin states that blockchain networks can struggle to equally achieve security, decentralization, and scalability. Consequently, different methods have been developed for enhancing system security:
Zero Trust Security Model: This model is based on the principle of "trust no one, always verify." It requires strict identity verification for every access request, ensuring that no device is trusted by default.
Decentralized Identity (DID): DID allows users to verify their identity without a central registry, contributing to a decentralized digital identity model.
- Fully Homomorphic Encryption (FHE): FHE allows computations to be performed on encrypted data without revealing the unencrypted content, making it critical for applications where data privacy is a concern, like cloud computing.
Projects like uPort and NKN have emerged in response to these challenges, focusing on decentralized identity and security models. Additionally, Mind Network has taken a lead with FHE technology, collaborating with major tech firms to protect data in AI systems.
Addressing Security at Various Levels
Solutions can be approached at different levels to mitigate risks associated with AI:
Data Level: Encrypting user-input information, including biometric data, ensures that even Manus cannot access unencrypted information.
Algorithm Level: Implementing FHE can obscure the decision-making paths of AI models from developers.
- Collaboration Level: Using threshold encryption for communication between agents can protect data integrity, ensuring that a single point of failure doesn’t compromise sensitive information.
In this complex landscape of AI development, security is not just a precaution—it’s essential for navigating the risks associated with advanced technology.