Trends and Milestones of AI in Healthcare
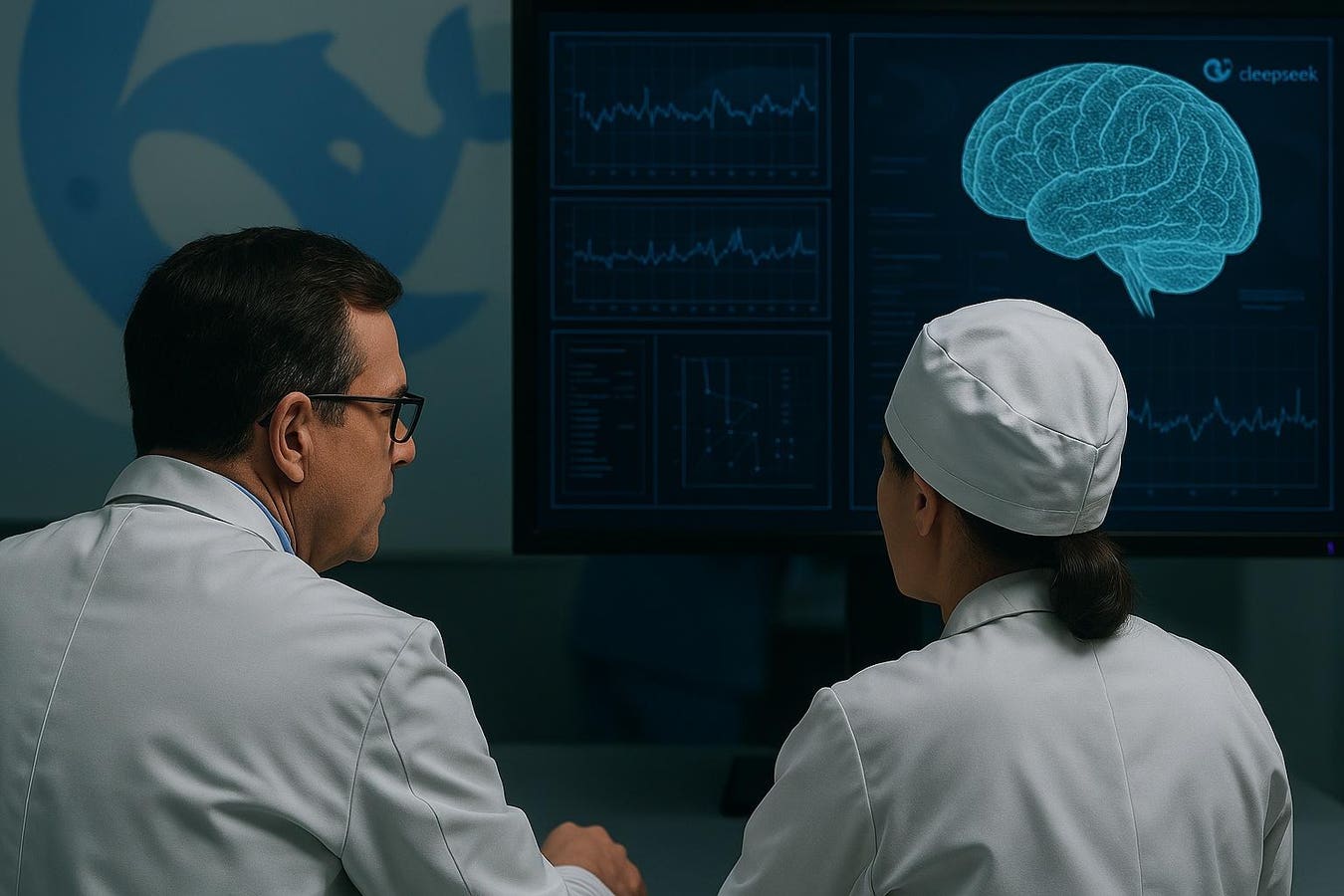
The Future of Healthcare: The Impact of AI
Transforming Healthcare with AI
Artificial intelligence (AI) is on the verge of revolutionizing the healthcare landscape both in the United States and around the globe. Various countries will employ AI systems differently; however, the primary aim is similar: to tackle challenges such as clinical workforce shortages, improve diagnostic accuracy, and enhance chronic disease management within populations.
Understanding AI in Healthcare
AI is not just about creating aesthetically pleasing visuals or generating creative text. Instead, it can serve critical roles in fields like medicine. Large Language Models (LLMs) can be utilized in both artistic and scientific domains. Nonetheless, utilizing these technologies in healthcare involves complex integration processes. This includes not only technical setup but also ensuring compatibility with existing infrastructure and business operations, which can be challenging.
Insights from Experts
Recently, Alvin Graylin from the Virtual World Society, along with GenAI Consultant Karl Zhao, shared their perspectives on the current and future applications of AI in healthcare. They discussed that while healthcare systems across the globe face unique challenges, the interconnectedness of international trade plays a crucial role in how different nations adopt AI solutions.
The Shift Towards Open-Source AI Solutions
Why Open-Source Matters
AI is changing healthcare by streamlining clinical workflows and addressing pressing issues like workforce gaps and diagnostic effectiveness. The healthcare sector in the U.S. has taken the lead in adopting generative AI, with investments projected to reach $500 million in 2024, surpassing other sectors significantly. However, leveraging AI effectively requires more than advanced technology; it necessitates smooth integration with current systems, transparency in costs, and adherence to privacy regulations.
Open-source AI models, such as DeepSeek R1 and V3, are gaining traction because they provide cost-efficient, customizable, and transparent solutions tailored for healthcare. These models allow organizations to monitor algorithms, comply with regulations, and modify systems to meet specific clinical requirements—eliminating the risks associated with proprietary software.
Nvidia and the Open-Source Movement
Nvidia’s recent strategic shift reinforces this trend. The company is now partnering with specialists in industry-specific AI solutions built on open-source frameworks rather than focusing solely on hardware. For example, tools powered by DeepSeek have recorded a 40% decrease in diagnostic turnaround times and improved identification of rare diseases by 28%. Such advancements emphasize how combining open-source models with expertise can lead to significant improvements in precision medicine.
The Financial Landscape of AI Deployment
Breaking Down Costs
An essential yet often ignored aspect of AI implementation is its cost structure. Although hardware components like GPUs draw a lot of attention, software expenses constitute nearly 70% of the total deployment costs. This category includes fine-tuning models, integrating with electronic health records (EHRs), and ongoing support. Open-source options help reduce costs by minimizing licensing fees and enabling organizations to adjust systems as needed.
Privacy Concerns and Deployment Strategies
Increasing concerns around data privacy and security are prompting many healthcare providers to shift from large cloud platforms to on-premise solutions. According to Alvin Graylin, organizations are often hesitant to store sensitive patient data in the cloud, especially in a heavily regulated environment like healthcare, where compliance with laws like HIPAA is essential.
In China, strict data localization laws require hospitals to maintain patient records on-site, spurring a demand for private AI solutions. In the U.S., while regulations are more lenient, anxiety about cloud security outweighs the convenience of remote data storage for many healthcare providers. As Karl Zhao pointed out, the flexibility of software and deployment is frequently overlooked in AI planning.
Advancements in AI Ecosystems
The Emergence of Specialized AI Ecosystems
The combination of open-source models, transparency in costs, and secure on-premise deployments is transforming the future of healthcare AI. Companies such as Stryker, Boston Scientific, and Medtronic are already experiencing stock surges linked to AI innovations. Meanwhile, leading cloud provider services like AWS and Google Cloud face increasing competition from local processing units.
As Alvin Graylin remarked, integrating AI solutions isn’t just a straightforward task; it demands time and effort to connect with existing systems. The future holds a promise of ecosystems where open models, specialized knowledge, and secure infrastructure converge to create real-world impacts in healthcare. This approach aims for quicker diagnostics, enhanced patient outcomes, and a more viable ascent of AI adoption—prioritizing privacy, cost-effectiveness, and scalability.