Understanding AI Factory and Nvidia’s Investment Strategy
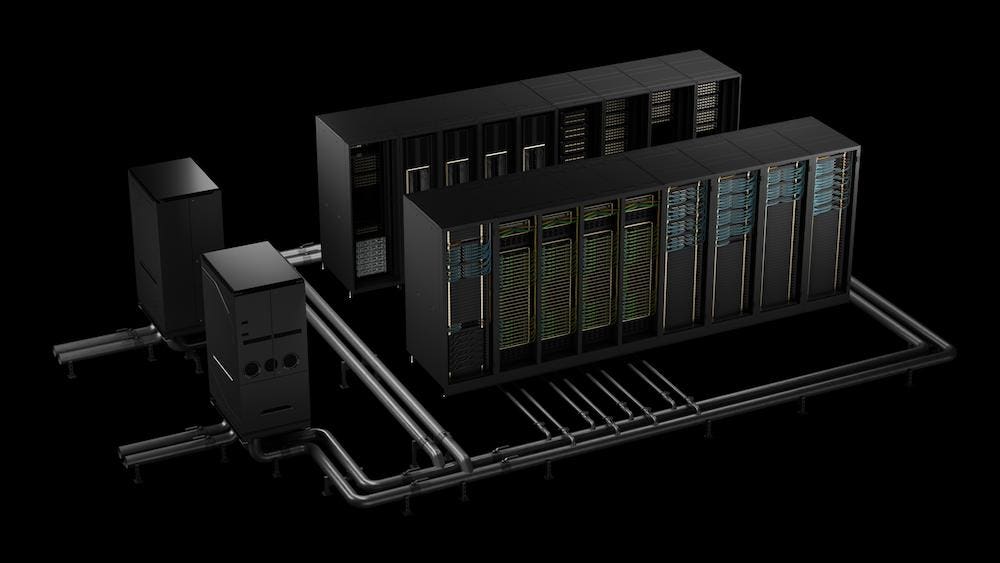
AI Factory
Nvidia
During the recent Nvidia GTC conference, the term “AI factory” was frequently used, gaining prominence particularly after Nvidia CEO Jensen Huang mentioned it in his keynote presentation. This term reflects Nvidia’s vision of large-scale AI system production and aims to transform how AI is developed.
Understanding the AI Factory Concept
An AI factory is a specialized system designed to extract value from data by managing the entire life cycle of artificial intelligence projects—from data collection and model training to adjustments and large-scale use. Think of it like a traditional factory where raw materials, in this case, data, undergo processing to create finished goods, which in the AI context are insights or decisions.
The primary output of an AI factory can be quantifiable predictions or responses, also known as AI tokens, which facilitate business decisions. Unlike general data centers that accommodate a variety of workloads, AI factories are specifically engineered for AI, streamlining processes and significantly speeding up the time it takes to deliver value. Huang has pointed out that Nvidia’s evolution shifted from just selling chips to creating substantial AI factories, establishing Nvidia as a dedicated AI infrastructure provider.
AI factories go beyond mere data storage and processing; they create various forms of content including text, images, and videos. This marks a significant change from simply analyzing data to generating customized outputs using artificial intelligence. The shift aims to make AI a beneficial tool for organizations, turning it into a competitive advantage rather than a lengthy research initiative, similar to how traditional manufacturing directly contributes to revenue.
Key Factors Driving AI Demand
The landscape of generative AI is rapidly advancing. Modern AI models are not just about simple token creation but involve complex reasoning and decision-making processes. The following three scaling laws are vital in understanding the growing need for AI computational power:
- Pre-training Scaling: Utilizing larger datasets and model parameters typically leads to greater intelligence, although this demands enormous computing resources. In the last five years, demands for computational power in this area have surged by a staggering 50 million times.
- Post-training Scaling: Fine-tuning existing AI models for real-world applications can require 30 times more computation during the inference phase compared to the initial training stage. As organizations customize these models, their infrastructure needs increase drastically.
- Test-time Scaling: Advanced AI applications that require iterative reasoning can use up to 100 times more computational resources. This makes traditional data centers insufficient for handling current AI demands, which is where AI factories come into play.
The Hardware Behind AI Factories
Establishing an AI factory necessitates a robust hardware foundation. Nvidia provides this through its cutting-edge chips and systems. Central to these factories are high-performance Graphics Processing Units (GPUs) that excel in processing large-scale parallel computations essential for AI. Since entering data centers in the early 2010s, GPUs have significantly increased performance efficiency, making them ideal for demanding AI applications.
Modern data center GPUs, like Nvidia’s Hopper and the latest Blackwell architecture, are pivotal in this new industrial setting. They are usually found in Nvidia’s DGX systems, which are ready-to-use AI supercomputers. Excitingly, the Nvidia DGX SuperPOD serves as a model for AI factory setups, providing optimized computing resources for enterprises.
In addition to computational strength, the networking infrastructure also plays a crucial role in AI factories. These operations require moving vast amounts of information rapidly between various processors. Nvidia solves these challenges with technologies such as NVLink and NVSwitch, high-speed interconnects that enable exceptional data-sharing capabilities among GPUs. For enhanced server-to-server communication, Nvidia implements rapid networking solutions like InfiniBand and Spectrum-X Ethernet switches, often combined with BlueField data processing units.
The Software Ecosystem of AI Factories
Having powerful hardware is essential, but Nvidia’s AI factory vision also includes a comprehensive software stack to leverage this infrastructure effectively. The foundation is built on CUDA, Nvidia’s parallel computing model that allows developers to harness GPU power. This has become the standard for developing AI algorithms optimized for Nvidia hardware.
Additionally, Nvidia offers Nvidia AI Enterprise, an extensive software suite that simplifies AI development processes. It integrates over 100 frameworks and tools, aligning them with Nvidia GPUs, to support every phase from data preparation to model deployment. This suite acts as the operating system for an AI factory, providing the necessary components for innovative AI development.
Moreover, tools like Nvidia Base Command are essential for efficient management within the AI factory. It helps with job scheduling, data management, and monitoring GPU resources in multi-user environments. Tools such as Run:AI, and Nvidia Mission Control offer comprehensive management solutions to optimize workloads and ensure reliability.
Nvidia’s Omniverse platform also plays a pivotal role in this ecosystem. It allows for the creation of digital twins and simulation environments inside AI factories, providing engineers with tools to optimize the design and operational aspects of their facilities. This capability to simulate before deploying helps limit risks and accelerate the implementation of new architectures.