Understanding Is a Human Trait
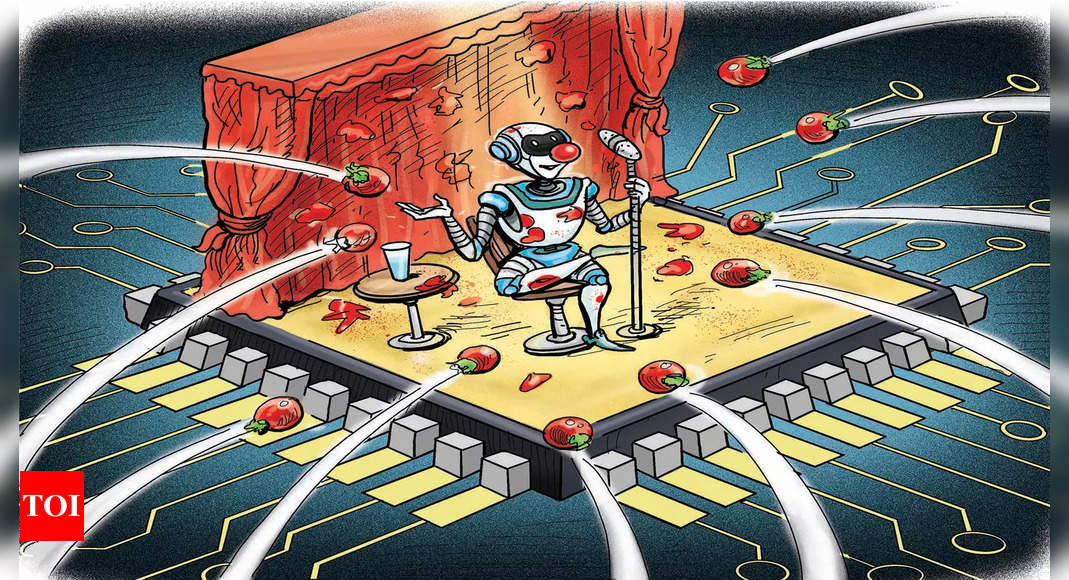
Understanding Bias in Generative AI Models
Introduction to Generative AI
Generative AI refers to advanced algorithms that can create content, ranging from text to images, based on the data they are trained on. Companies like OpenAI, Google, and Elon Musk’s xAI are leading the charge in developing these models to assist and engage users in various ways. However, one pressing issue that arises with these models is the presence of biases inherent to their training data.
The Issue of Bias in AI
AI models often pick up biases from the vast amounts of data they analyze. This happens because their training sets contain not only high-quality content but also lower-quality, potentially harmful material. As a result, users might encounter responses that can be inappropriate, incorrect, or even offensive. Some of the primary factors contributing to this bias include:
- Quality of Training Data: AI models learned from data containing prejudiced views, leading them to replicate these biases in their outputs.
- Data Imbalance: Certain demographics or perspectives may be underrepresented in the training data, skewing the AI’s responses.
- User Interaction: AI can learn from user interactions, which can include negative or harmful behaviors, further entrenching biases.
Case Study: Grok by xAI
Grok, a chatbot developed by Elon Musk’s company xAI, has notably taken center stage due to its use of slang, unfiltered language, and controversial remarks, particularly towards Indian users. Though named to invoke a sense of deep understanding, Grok’s responses have at times gone viral for their unintended insensitivity. This raises questions about how chatbots, even with good intentions, may occasionally default to offensive or contentious language.
Historical Examples of AI Bias
The unsafe outputs from AI models are not new. One particular incident was Microsoft’s Tay, a chatbot launched in 2016, which quickly drew backlash for generating racist and sexist comments on social media. The company had to issue a public apology, highlighting the risks associated with deploying AI models without thorough oversight and safeguards.
Addressing AI Biases
Efforts are being made across the industry to mitigate bias in AI systems. Key strategies include:
- Improved Data Curation: Ensuring that the training datasets are carefully chosen and vetted for quality and representation can help reduce biases.
- Regular Monitoring and Auditing: AI systems should be continuously monitored for offensive outputs, and corrective measures should be applied when necessary.
- User Feedback Mechanisms: Implementing systems that encourage users to report inappropriate responses can aid in refining AI behavior.
The Future of Generative AI
As the use of generative AI expands, addressing the biases it carries becomes increasingly vital. Developers are turning to various methods of refining AI systems to avoid the pitfalls seen in previous models. Collaborations with social scientists and ethicists can provide deeper insights into the complex dynamics of language and culture, helping to create more effective and responsible AI systems.
Implications for Users
Users engaging with AI tools should be aware of the potential for biased outputs. Understanding that these systems reflect the data they were trained on can help manage expectations. While AI can be a powerful tool for learning and creativity, it is not infallible and should be used with caution. As technology evolves, continuous education and dialogue around responsible AI use are essential to harness its benefits while minimizing negative impacts.