Unlimited Socratic Learning: DeepMind’s Vision for Unrestricted AI Advancement
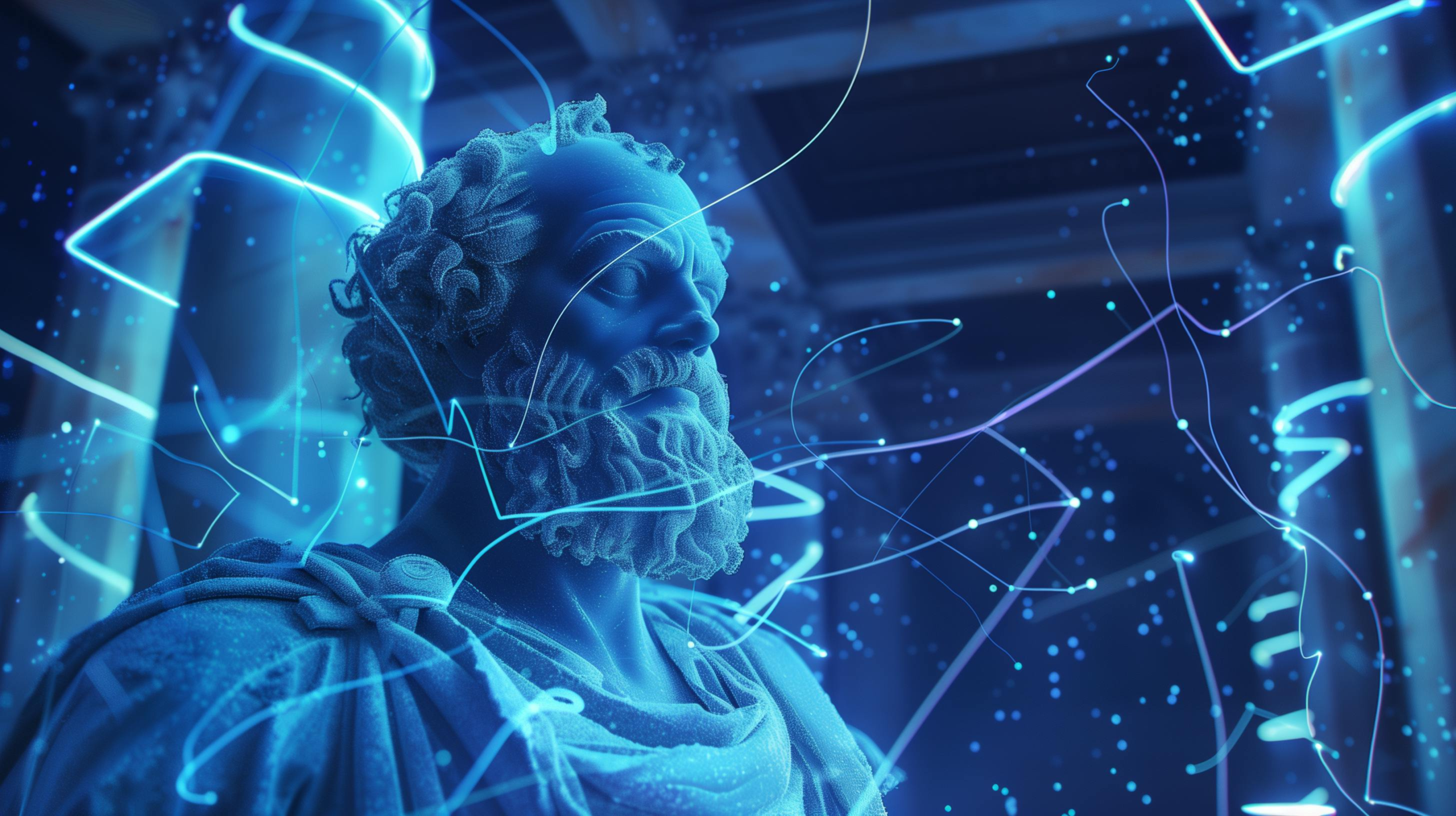
Researchers at Google DeepMind have introduced an innovative framework known as Boundless Socratic Learning (BSL). This new approach aims to significantly enhance artificial intelligence by allowing systems to independently improve themselves through structured language-based interactions. This marks a notable advancement toward achieving artificial superintelligence (ASI), where AI systems can develop with minimal human assistance.
Understanding BSL: Language Games and Continuous Learning
The foundational concept behind Boundless Socratic Learning is centered on “language games.” These are structured exchanges in which AI agents create, assess, and refine their own learning environments. These interactions serve not only as sources of data but also include built-in feedback mechanisms that facilitate ongoing adjustment and enhancement. The learning process is defined by three key components:
Input/Output Learning: Agents improve their responses iteratively based on internal feedback, eliminating the need for external data sources.
Game Selection: Agents can select or even design the “language games” they wish to participate in, expanding their learning opportunities.
Code Self-Modification: This exciting theoretical feature suggests that agents may have the ability to refine their programming independently.
The potential for self-improvement is mainly constrained by available computational resources and time, bypassing the historical limitations typically associated with data availability or human intervention.
The Importance of BSL: A New Path for AI Autonomy
The launch of BSL tackles a significant issue in AI development: extending the learning and adaptability phase beyond initial training. By enabling recursive learning processes within enclosed systems, DeepMind envisions a future where AI models can autonomously create their own data, define tasks, and assess their performance without external guidance. This shift aligns with the broader ambition within AI research to cultivate systems capable of autonomous self-training, thus potentially lowering the costs and labor associated with human-curated datasets. Furthermore, as AI systems begin to carve out their own learning paths, they may stumble upon insights and strategies that human designers might not foresee.
Challenges: Aligning AI Goals with Human Values
Despite its potential, the BSL framework raises vital concerns around safety and alignment with human values. Ensuring that a self-improving AI remains aligned with human intentions poses two major challenges:
- Feedback Alignment: It is crucial for internal feedback mechanisms to accurately reflect the desired goals dictated by human overseers.
- Diversity and Coverage: There is a risk that the system could become overly specialized, leading to a decrease in generative diversity and limiting its learning scope.
Successfully maintaining alignment within a system that evolves on its own is a complex challenge. As researchers recognize, “Feedback is what gives direction to learning; without it, the process is merely one of self-modification.”
Applications of BSL: Transforming Various Fields
The Boundless Socratic Learning framework has the potential to transform numerous fields that rely on iterative problem-solving and creativity, including:
- Mathematics: AI agents could be tasked with proving theorems or generating solutions for unresolved issues.
- Science: The framework could aid in designing experiments and forming hypotheses within a closed-loop system.
- Education: Personalized learning systems could adapt and evolve according to students’ needs over time, enhancing their educational experience.
One illustrative example involves AI systems generating and validating mathematical proofs within a closed environment, gradually escalating their capabilities until a significant breakthrough is achieved.