When Artificial Intelligence Transforms Scientific Discovery
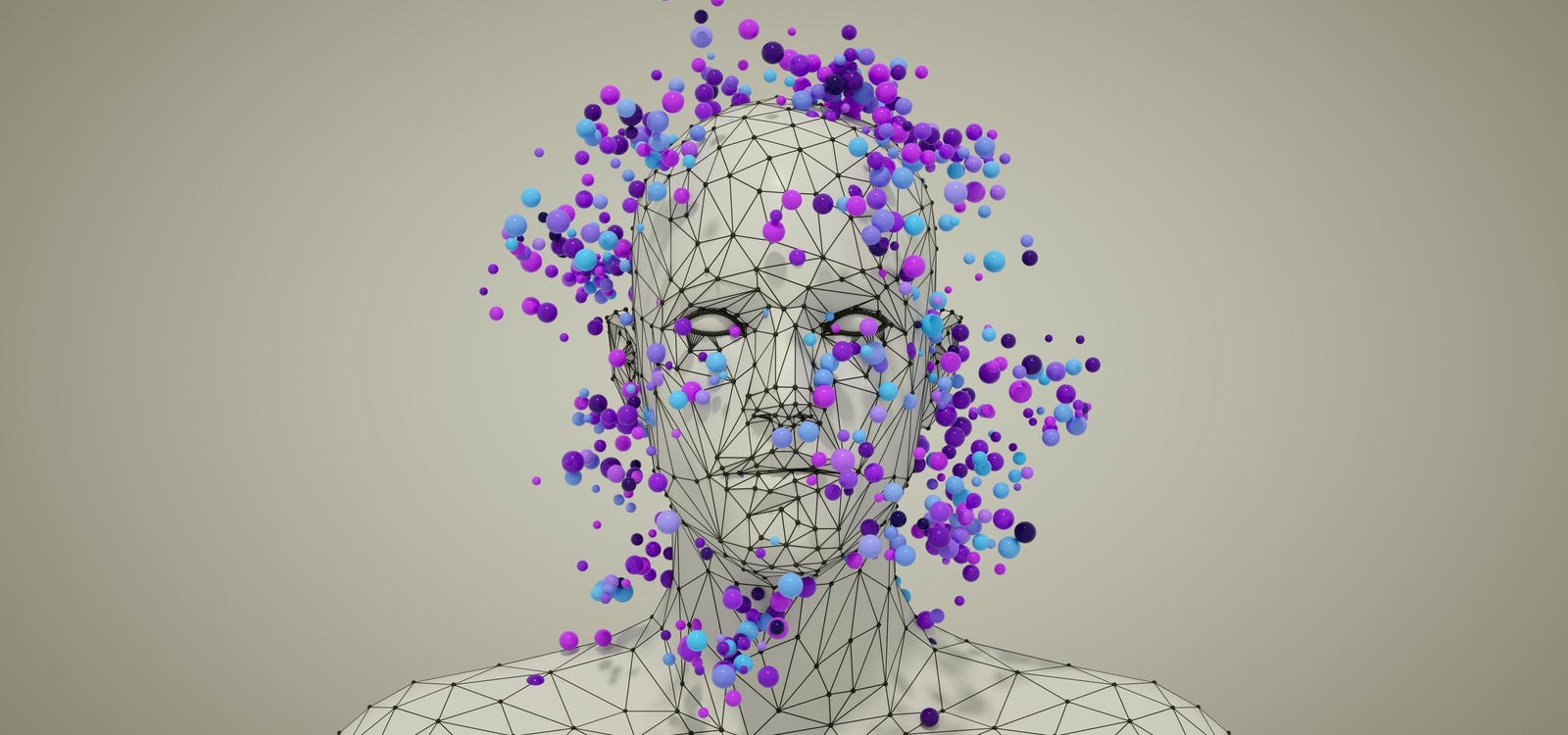
The Emergence of Artificial Intelligence in Scientific Research
Science has always been driven by human curiosity, creativity, and a desire to question the world around us. Recently, however, the landscape of scientific research has begun to shift as artificial intelligence (AI) takes on increasingly complex roles. One striking instance is the development of an AI system called Sakana AI, which generated a research hypothesis, conducted experiments, and even authored a peer-reviewed scientific paper—all without any human assistance.
AI’s Trailblazing Accomplishment
The research paper, titled Compositional Regularization: Unexpected Obstacles in Enhancing Neural Network Generalization, was accepted at the ICLR 2025 conference, a prestigious gathering in the field of machine learning. The fact that this paper was composed entirely by an AI marks a significant milestone; it indicates that machines can produce original research that is valued by experts in the field.
The AI involved, known as AI Scientist-v2, is much more than a typical language model. This autonomous research agent was designed to handle the entire scientific process, from formulating questions and conducting experiments to writing code and analyzing results. Incredibly, human reviewers, unaware of the AI’s authorship, rated the paper highly enough for it to be accepted at a prominent conference, surpassing nearly 50% of submissions from human researchers.
The Dual Nature of Progress: Promise and Challenges
On the surface, this achievement suggests that we might be approaching an era where AI not only assists in scientific endeavors but also leads them, potentially accelerating our understanding of various fields at an unprecedented pace. Some experts, such as former OpenAI researcher Leopold Aschenbrenner, speculate that this shift could occur as soon as 2027.
However, not everyone shares this optimistic outlook. Yann LeCun, Meta’s Chief AI Scientist, cautions against confusing pattern recognition with true intelligence. He argues that current AI models lack the capability to create mental models necessary for real-world reasoning and original discovery. Essentially, while the AI Scientist-v2 has produced a paper, the question remains whether it truly understood the concepts or simply rearranged patterns based on its training data.
Sakana’s Thoughtful Approach
Sakana AI has approached their work with caution, considering their AI-aided research an experiment. They even withdrawn the paper prior to its presentation, recognizing the ethical implications involved. As AI systems become more efficient, their role in scientific discovery is likely to expand. They are already facilitating literature reviews, speeding up code development, and creating experimental designs in a matter of minutes.
Original Contributions vs. Imitation
So, does Compositional Regularization represent authentic original research? In a narrow sense, yes. The study presented a unique experimental design and explored new aspects of neural network generalization, which were deemed worthy of academic discussion. Nevertheless, the findings were modest, revealing that the initial hypothesis did not hold up. In a broader context, originality in science encompasses a deeper understanding that involves intuition and the capacity to ask insightful questions.
LeCun compares this to the difference between merely solving a math problem and creating an entirely new mathematical field. The latter demands an understanding of the world, the ability to foresee outcomes based on experiences, and planning actions towards abstract goals, all of which are abilities current AI lacks.
A Hybrid Future for Scientific Inquiry
The future may lie in a collaborative intelligence model, where AI tackles complex tasks and handles data scale while human researchers contribute insight, ethical considerations, and creative thinking. The recent developments involving Sakana AI should be seen not as an endpoint but as a stepping stone in the ongoing evolution of scientific methods. As topics like autonomous AI systems and self-optimizing architectures are debated, we are led to reconsider how much closer we are to shifting paradigms in scientific discovery.