With AI Models Dominating Every Benchmark, Human Evaluation is Now Essential
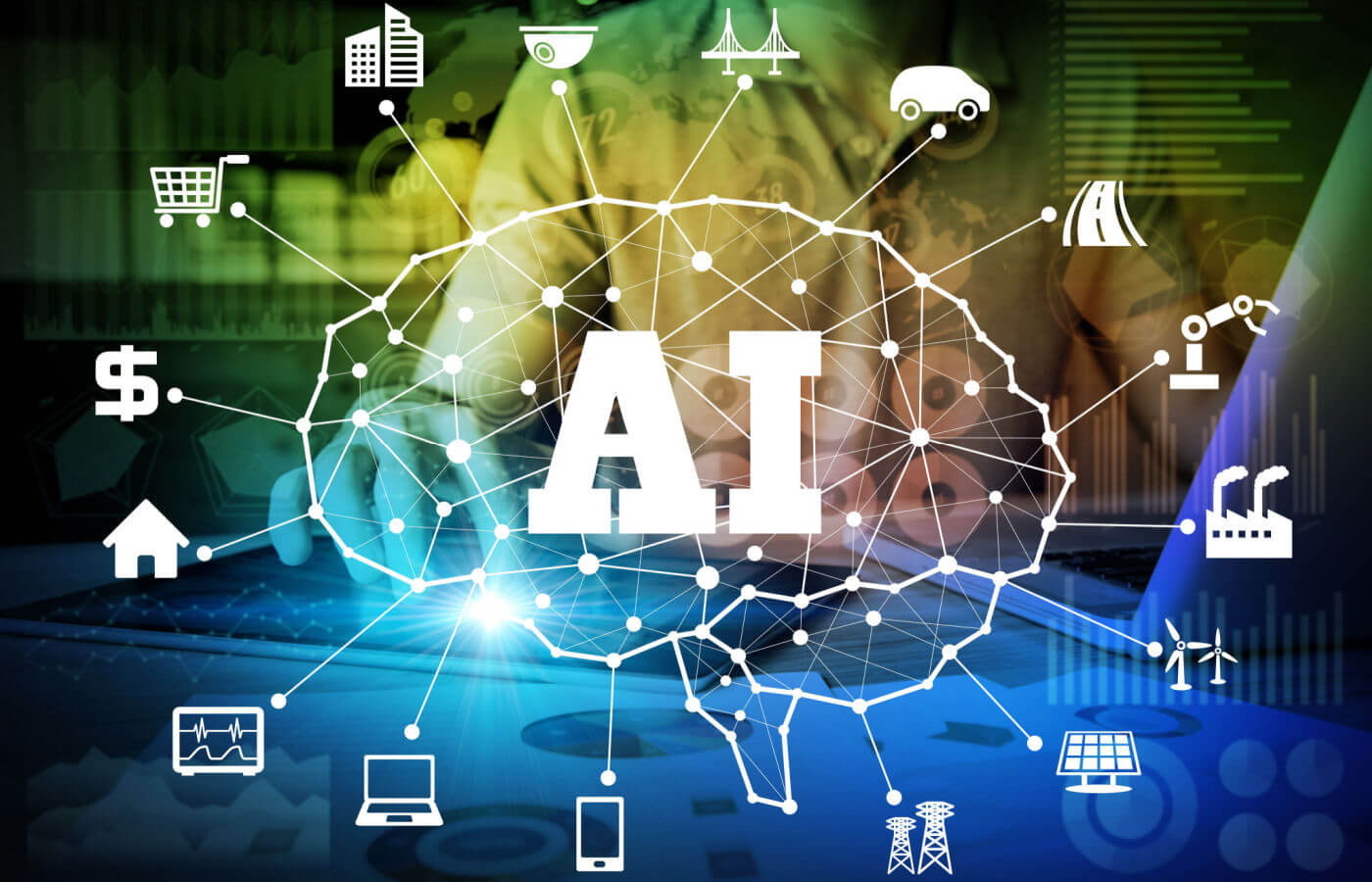
The Evolving Role of Human Assessment in AI Evaluations
Introduction to AI Benchmarks
Artificial Intelligence (AI) has advanced through various evaluation methods designed to mimic human knowledge. Notable benchmarks, including the General Language Understanding Evaluation (GLUE) and the Massive Multitask Language Understanding (MMLU) dataset, have played a key role. These frameworks use a vast array of questions to judge how well an AI model comprehends and processes information.
Transitioning from Conventional Benchmarks
However, many experts argue that traditional benchmarks are becoming insufficient for adequately assessing the capabilities of generative AI. Michael Gerstenhaber, head of API technologies at Anthropic, has pointed out that “we’ve saturated the benchmarks.” The industry now recognizes that human evaluations could provide a more effective means of assessing AI outputs.
Importance of Human Evaluations
A recent study published in The New England Journal of Medicine by researchers from various institutions, including Boston’s Beth Israel Deaconess Medical Center, emphasizes human assessments as crucial for evaluating AI benchmarks. The study argues that commonly used benchmarks like MedQA, developed at MIT, are no longer challenging for AI models, as they can effortlessly pass these exams without truly applying their knowledge in real-world clinical settings.
The researchers recommend adapting training methods typically used for human physicians, such as role-playing, to better assess AI performance. Although these human-computer interaction studies may take longer, they highlight the importance of human involvement as AI systems become more sophisticated.
Reinforcing Human Oversight in AI Development
The integration of human input into AI development is not a new trend; it has been a fundamental part of progress in generative AI. For instance, the development of ChatGPT in 2022 extensively utilized a method known as "reinforcement learning with human feedback." This approach allowed humans to evaluate AI outputs repeatedly, helping guide the AI towards more desirable results.
Currently, OpenAI and other leading AI developers are inviting human evaluators to rank and assess their models’ performances. For example, when Google launched its open-source model Gemma 3, it placed significant emphasis on human ratings rather than solely on automated benchmark scores to argue for its superiority.
Innovative Evaluation Methods in AI Luminaries
In a similar vein, OpenAI’s recent introduction of model GPT-4.5 underlined the significance of human reviews. The company highlighted that human preference assessments play a vital role in gauging whether users preferred the new model over its predecessor, GPT-4. The assertion of GPT-4.5 possessing a higher "emotional quotient" also demonstrates a shift toward incorporating subjective evaluations in AI performance assessments.
New Benchmarks Involving Human Participation
As the landscape of AI continues to evolve, benchmark developers are increasingly incorporating human involvement into their frameworks. For instance, OpenAI’s GPT-3 "mini" was the first large language model to surpass human scores in an abstract reasoning test known as the Abstraction and Reasoning Corpus for Artificial General Intelligence (ARC-AGI).
The ARC-AGI has recently been updated, with its creator, François Chollet from Google, introducing a challenging new version that directly used feedback from over 400 members of the public. This approach not only measures AI performance against human benchmarks but also actively engages human participants in defining the challenges.
Conclusion: The Future of AI Evaluation
The ongoing blend of automated testing with enhanced human participation suggests significant potential for refining AI development processes. As researchers and developers explore these synergies, the path towards greater integration of human feedback stands to reshape the future of AI technologies. Through this advanced evaluation framework, AI systems can better align with real-world applications, ensuring they meet the needs of users effectively.