Yann LeCun from Meta: Increasing AI Scale Doesn’t Enhance Its Intelligence
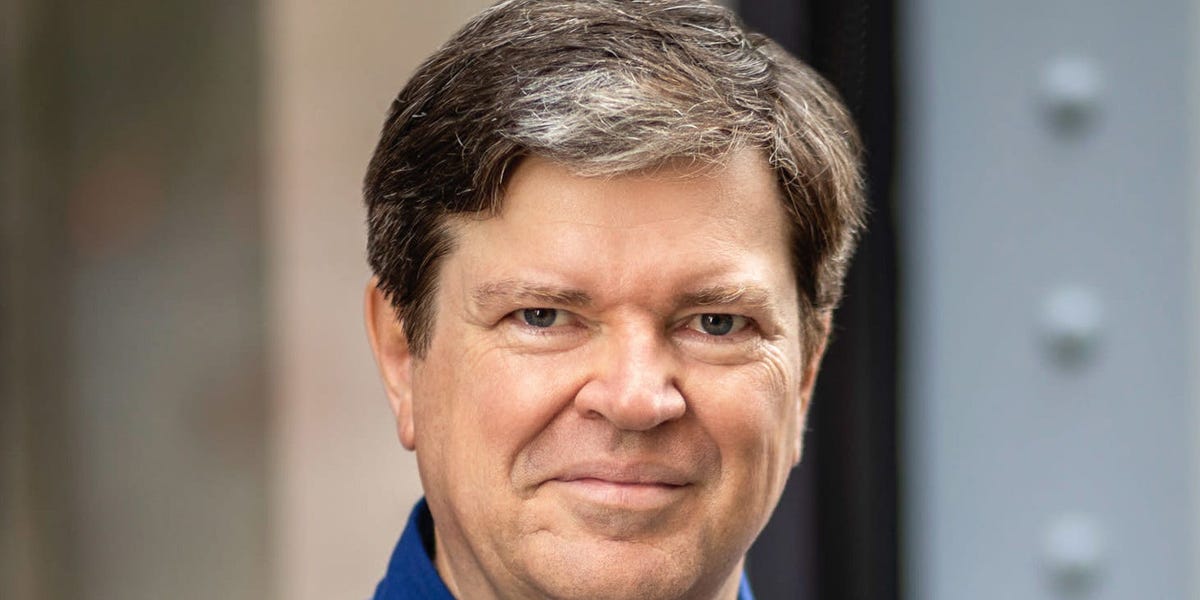
Understanding the AI Scaling Laws and Emerging Perspectives
What Are Scaling Laws?
The concept of scaling laws in artificial intelligence (AI) has guided researchers and developers for several years. These principles were notably articulated in OpenAI’s influential 2020 paper titled "Scaling Laws for Neural Language Models." In essence, scaling laws suggest that the performance of AI models is significantly linked to three main factors:
- Number of Parameters (N): Refers to the complexity of the model, excluding embeddings.
- Size of the Dataset (D): Indicates the amount of information the model can learn from.
- Compute Resources (C): Relates to the computational power utilized during training.
According to these laws, the idea that "more is more" drives the growth of highly advanced AI systems. This principle has encouraged substantial investments in data centers, enabling AI models to analyze and learn from vast troves of data.
A Shift in Perspective
Despite the long-standing belief in the effectiveness of scaling laws, notable figures in the AI field are beginning to question this doctrine. Yann LeCun, Meta’s chief AI scientist, expressed skepticism during a recent talk at the National University of Singapore. He emphasized that simply increasing data and computational resources doesn’t necessarily lead to more intelligent AI systems.
LeCun pointed out that many current AI models, which excel in performance, often rely on relatively simple data. He noted that the data utilized in training some of today’s largest language models is roughly equivalent to what a four-year-old can process. The implication is that as problems grow in complexity and ambiguity, scaling alone won’t suffice.
The Limits of Scaling
LeCun argued that a fundamental misunderstanding exists in the AI community, where simple algorithms are incorrectly extrapolated to solve complex issues. Many AI practitioners fall into the trap of believing that merely enhancing scale will naturally yield smarter AI.
He explained that much of the recent advancement in AI has involved relatively simple breakthroughs. As AI systems face more intricate and nuanced real-world challenges, relying solely on a scaled-up approach becomes less effective.
Industry Insights
LeCun is not the only expert raising concerns about the limitations of scaling. Other leaders in the AI space share similar sentiments. For instance, Alexandr Wang, CEO of Scale AI, described scaling as "the biggest question in the industry." Meanwhile, Aidan Gomez, CEO of Cohere, referred to the scaling approach as the "dumbest" way to enhance AI.
LeCun advocates for training AI systems that can swiftly learn and adapt to new tasks. He suggests these systems should not only understand text and language but also grasp aspects of the physical world, suggesting a need for common sense reasoning and planning abilities similar to what humans possess.
The Case for World Models
During appearances, including an episode on Lex Fridman’s podcast, LeCun noted that world models should be developed in contrast to current large language models. Unlike systems that simply predict their next step based on input patterns, world models can anticipate how actions may influence future events, allowing for deeper cognitive capabilities.
To summarize, while scaling laws have provided a foundational understanding of AI capabilities, an increasing number of experts are advocating for a more nuanced approach to AI training. By focusing on developing systems that reflect a real-world understanding and memory retention, the AI industry can aspire to achieve genuinely intelligent and adaptive systems.