Yann LeCun, Meta’s AI Chief, Questions the Future of Generative AI
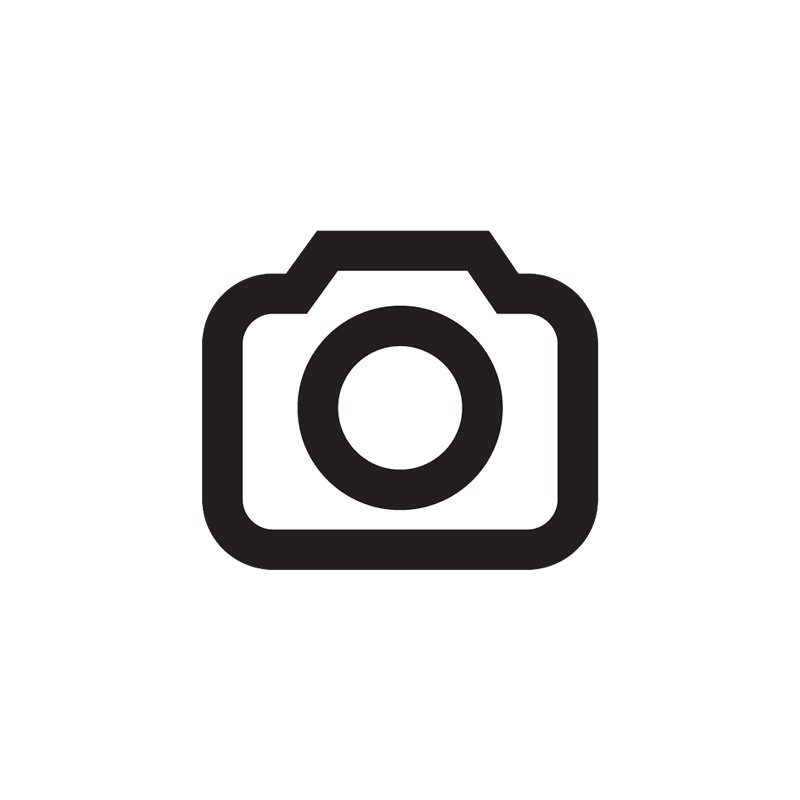
Yann LeCun’s Views on Artificial Intelligence
Yann LeCun, the Chief AI Scientist at Meta, raises important points regarding the concept of Artificial General Intelligence (AGI). At a recent event celebrating the 10th anniversary of Meta’s AI Research team, he stated that people who argue that AGI is just around the corner might not be outright lying but are certainly mistaken or have a very particular interpretation of what AGI means. This perspective is not just a jab at competitors like OpenAI, who have a profit-driven definition of AGI, but reflects a deeper concern about the trajectory of AI development.
FAIR: Meta’s Commitment to AI Research
Meta’s AI division, known as the Fundamental AI Research (FAIR), has been operational in Paris for a decade. During his talk there, LeCun emphasized the need for government support in advancing open-source AI. While most of Meta’s AI models are made available as open-source, there are some exceptions, such as the weights for the Llama model.
AMI Over AGI: A Shift in Perspective
LeCun introduced the term "AMI," which stands for Advancements in Human-Robotic Intelligence. He argues against the notion of AGI as the ultimate goal. For him, the pursuit of AMI is a more realistic objective, involving a series of incremental steps rather than a single dramatic breakthrough. One of the initial challenges is to identify the right architecture that can foster genuine AI intelligence. LeCun’s focus is on concepts such as zero-shot learning, where AI systems can perform tasks accurately without prior specific training. He likens this capability to a form of human intelligence, pointing out that even simple creatures like cats exhibit such forms of learning.
World Knowledge: A Foundation for AI Intelligence
LeCun emphasizes the importance of world knowledge as a core component of true intelligence. He critiques generative AI for not effectively building this knowledge base, as it fundamentally relies on making predictions. According to him, scaling models is a flawed approach. For instance, an AI may recognize the position of windows in a room but cannot accurately predict the room’s overall appearance. He boldly declares that the future of AI will not center on generative methods.
The Role of Visual Learning
LeCun believes that people learn through visual experiences rather than strictly through language. He posits that thoughts precede language, suggesting that non-verbal forms of cognition exist even in individuals who do not speak. This belief leads to the need for ‘natural data’—unstructured information that can be harnessed to develop an effective AI architecture. LeCun warns that misleading claims are made about current models’ capabilities, stating, “Models are trained for the tasks,” implying that they still lack genuine understanding.
Real-World Applications and Future Prospects
LeCun points out an interesting fact: A 17-year-old can learn to drive a car in just a few hours, yet fully autonomous "level 5" vehicles remain undeveloped. He cites examples like Waymo, which operates at a "level 4" capacity—limited in scope and reliant on remote human oversight when needed. LeCun stresses that current technology has not yet achieved the complexity and adaptability seen in even simple animals like cats.
In the Paris office, his team is working on creating more sophisticated intelligences, initially represented by a robotic dog named Spot. LeCun also envisions a future where smart glasses enhance daily life, showcasing a belief in the potential for AI to simplify tasks. While current generative AI serves a purpose, he believes the future will be marked by fundamentally different technological breakthroughs.
By emphasizing clear definitions and realistic goals for AI advancements, figures like Yann LeCun contribute to the ongoing dialogue about the future of artificial intelligence. Their insights inspire a more nuanced understanding of what true intelligence entails beyond current trends and marketing claims.